After running Clay-powered outbound ops for leading GTM teams like Sendoso, I’ve developed a playbook I want to share with you.
I call it the “AI Outbound Sales Funnel,” and it has 5 steps. We’re going to break them down in this post:
- Scoring accounts
- Surfacing the right contacts
- Personalizing outbound with account-level data
- Scaling personalized outbound emails
- Measuring and iterating

Before we dive in, you’re probably wondering why you should listen to me. I run Sculpted, a done-for-you Clay agency (Claygency). We power the outbound motion for leading SaaS companies like Sendoso, sending hundreds of thousands of emails a month.
I’ve emailed and called way more strangers than you could think of for over a decade. Here are some outbound motions I’ve run at several different companies:
- Founding GTM hire at ServiceBell, where I took the outbound motion from 0-1 and hired SDRs
- Full-cycle sales at an agency, using cold email to source and close $1mm+
- Client acquisition outreach for my first agency as a college dropout
- Outreach to fulfill client campaigns for backlink outreach
- Backlink outreach for Easy PC (my first online business in high school)
As an SDR myself, an SDR leader, and now working with leading SDR teams, I know a thing or two about outreach. I've seen winning outbound teams follow a five-step process.
In this post, I will walk through the process, step-by-step.
You'll see what we do, why we do it, and how you can automate the motion with Clay from end to end.
Don’t even start your outbound campaign unless you have a good offer
No matter how clever your copy is, your outbound campaign will only succeed if you nail your target market and offer.
In other words, you can't replace a valuable product or service with clever copywriting.
Poor product market fit is one of the main reasons why I turn down new clients. They want me to fix their outbound problem with clever email copy.
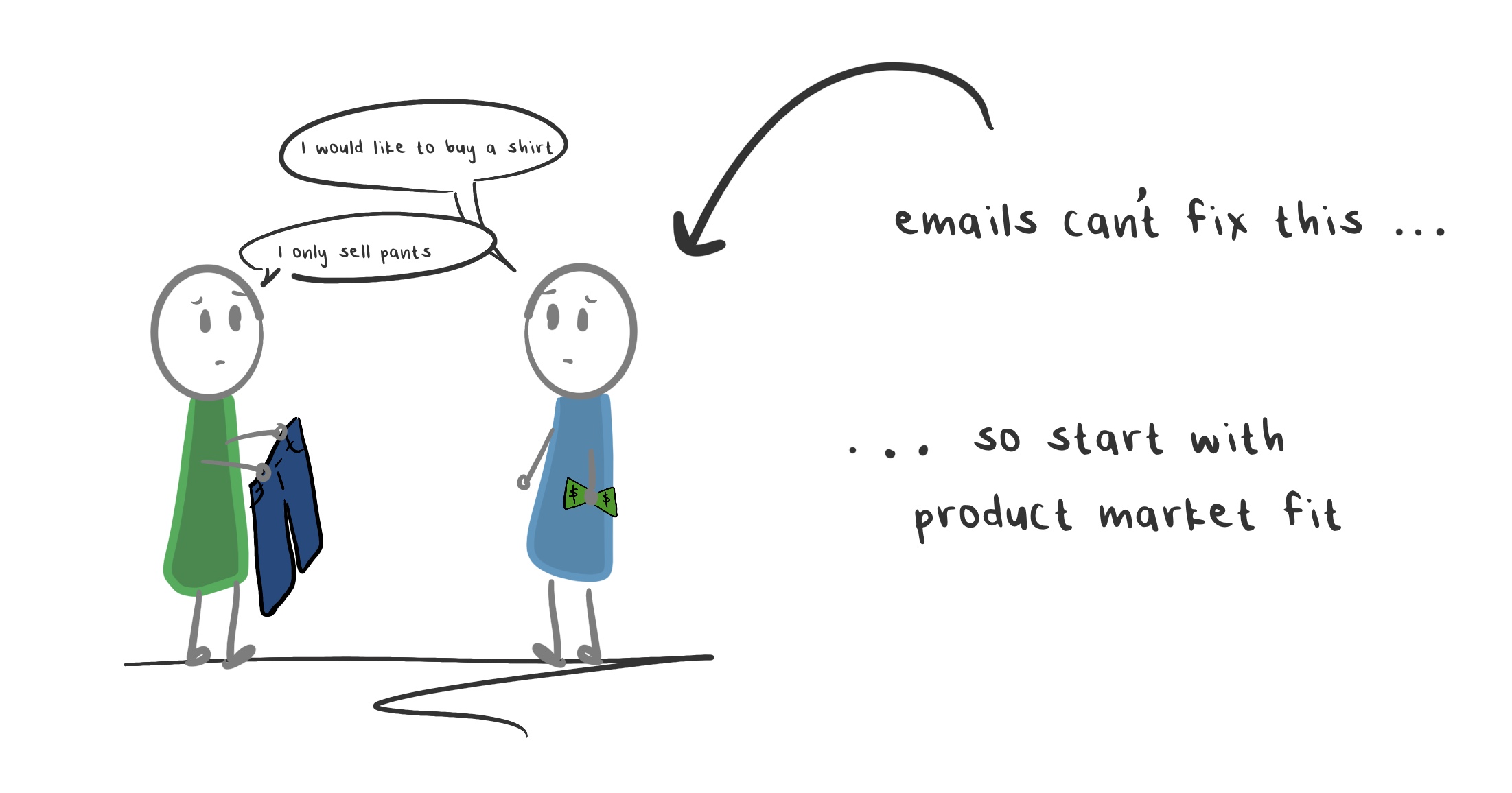
While poor copy does impact outbound conversion rates, the final decision is ultimately based on product market fit. Without good product market fit, no email sequence I can develop will replace or make up for a lousy offer.
To do account scoring the right way, get really specific
Account scoring starts with defining the problems you solve for your customers. Be specific and think through the symptoms of pain you can "spot in the wild.”
These are the signals you’ll use in your account scoring process to determine whether a company is a good fit.
(Why is it important to reach out to good-fit companies? This is probably self-explanatory, but they have problems you can solve and will be more enticed to take a meeting—and then more likely to turn into qualified pipeline.)
When most people think of symptoms, they use extremely basic data points. This is a huge missed opportunity considering Clay's power for problem-sniffing.
These are the standard fields I see folks using almost all the time:
- Employee count (from ZoomInfo or similar)
- Broad (and often incorrect) industry category
- Tech stack (from BuiltWith, often inaccurate)
These leave a ton to be desired. There are many more detailed data points you could use to score accounts. For example, you can obtain all of the below data points automatically on Clay:
- Scrape websites to look for specific keywords
- Infer specific industry categories that you define with LinkedIn company description + AI
- Look for open jobs that signal a company has a relevant problem. Scrape the job description and use AI to infer current pains
- Recent hires or fires
- Employee headcount by job title
- Employee headcount growth (overall or by department)
- Look for tech stack in their website code or job descriptions
- Score accounts by website traffic amount with SEMRush
- Funding round data
- Online reviews from websites like TrustPilot, Google Maps, etc.
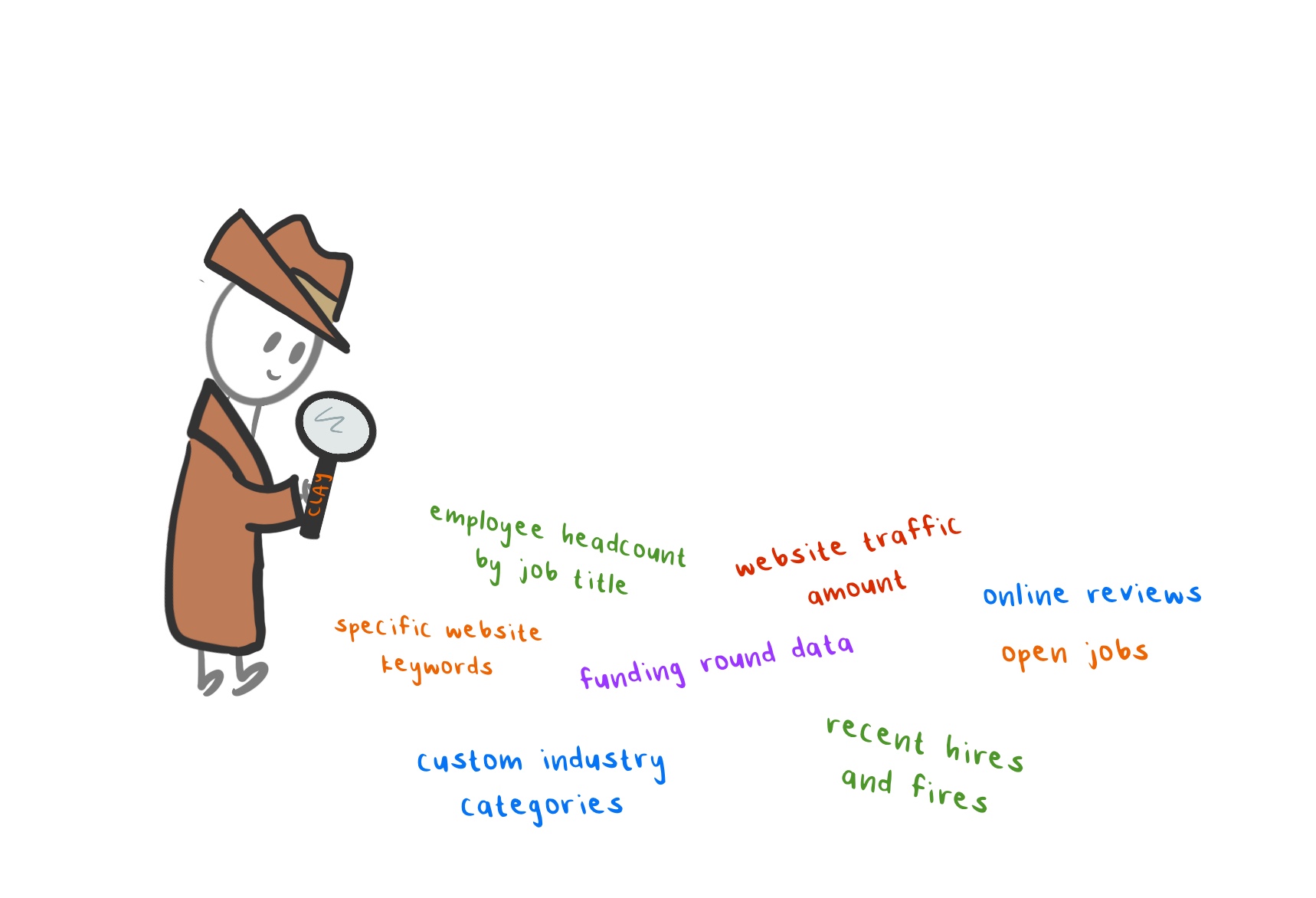
My previous, painful, manual way of account scoring
As a sales rep for ServiceBell, I wanted to find B2B SaaS companies running on HubSpot, with at least a few SDRs manning live support chat, and ideally a substantial amount of website traffic (which implied a good inbound leads.)
I'd use the following manual steps.
- Filter a list of companies using the "Computer Software'' industry filter on LinkedIn Sales Navigator
- Check the company LinkedIn and website to confirm they're a SaaS company and not a software development agency
- Look at the LinkedIn company page and manually search through their employees to look for salespeople on staff
- Check the website source code to look for the HubSpot scripts
- Check the website to see if they had a pricing page (which would be a good place for ServiceBell to live) and understand if they sold to engineering, security, or legal titles. (Companies who sold to any of those personas weren't usually a good fit for ServiceBell.)
- Lastly, plug their website into Ahrefs for SEMRush to see how much website traffic they have, to get a feeler for total inbound lead volume.
After all was said and done, I had a solid account, but that was after tons of manual research—at least ten minutes per qualified account.
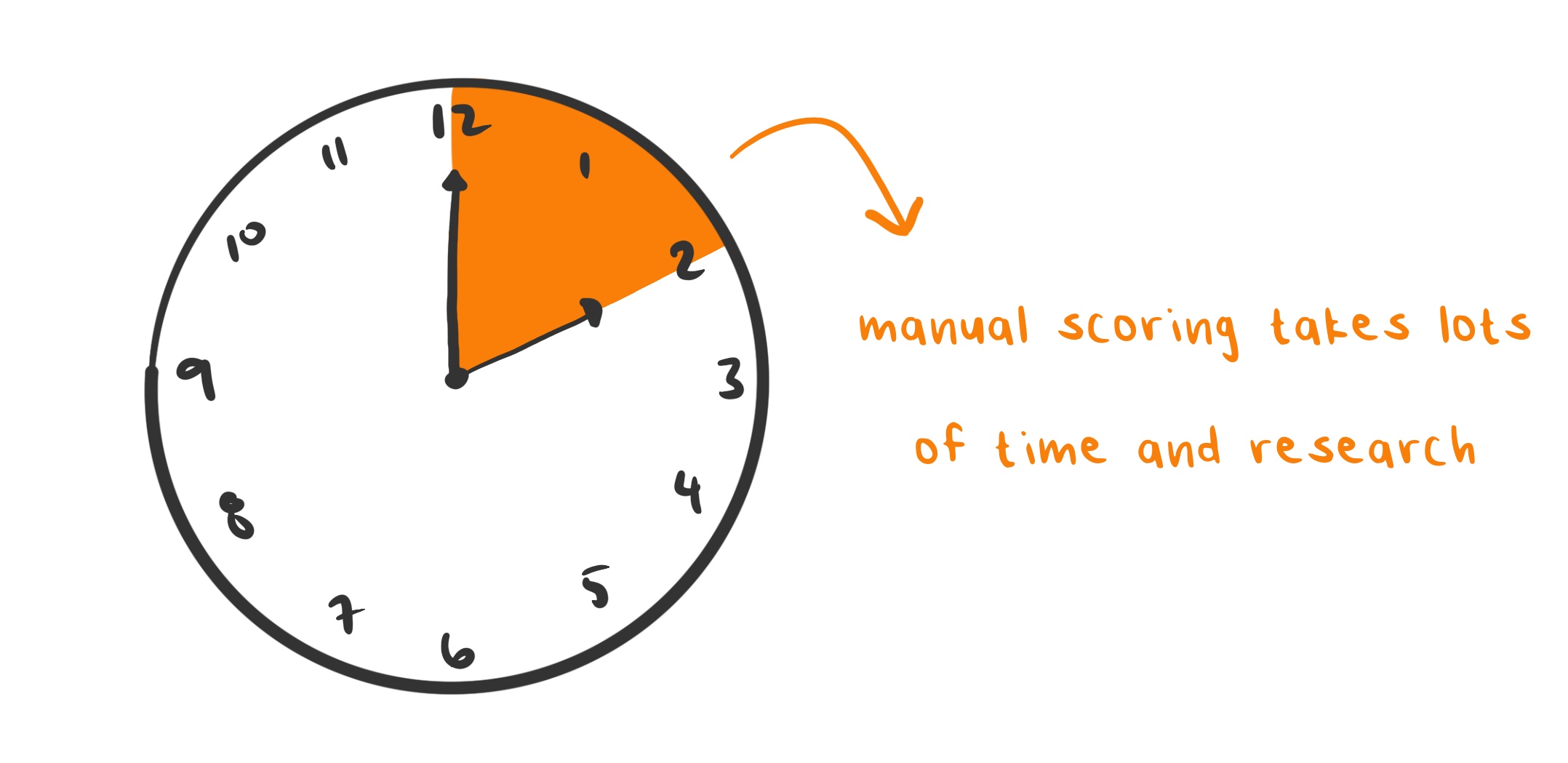
If I was working non-stop all day, I could find 48 qualified accounts. Of course, nobody works for 8 hours straight (lunch, bathroom, break, etc.) so the total number of accounts I could find in one day drops down to less than 48 daily.
This is not productive. At this point, I still hadn’t found the right contacts or their emails or sent any personalized outreach messages.
Sure, you could hire a VA or another service to do account research…but good VAs are hard to come by, need training, and are still limited by hours in the day. They are slower, less reliable, and more expensive than an automated solution like Clay.
Manual account scoring sucks—so most reps don’t even bother
Since the account scoring process is so tedious, most reps skip this step and opt for volume instead to show their manager "how much activity they're doing" daily despite the lackluster downstream results.
They pull a list from their prospecting tool, whether it's ZoomInfo, Sales Nav, etc., and call it a day. Their resultant account list is a mix of employee count and an often incorrect industry categorization, since huge companies like ZoomInfo can't possibly categorize accounts the same way you do.
Here’s the problem: your definition of your ICP is unique, and can't possibly be serviced by an "out-of-the-box" solution like ZoomInfo.
You either need to manually research the accounts or suffer from broad lists full of mostly junk accounts that wastes your team's time and guarantee that your booked meetings don’t turn into qualified pipeline.
How to automate your manual account scoring
With Clay, you can set up an account scoring model once, and automatically turn a raw list of company names or domains into a fully enriched account list, complete with all of the data that was previously only possible to find with a manual research process.
You can build lists of companies that are a good fit for your service, instead of blasting to unqualified accounts or spending too much time (i.e. any time) on manual research.
Here’s how I do it when I onboard new customers:
I understand how they'd define a "dream account".
I ask them to define the specific account-level qualification fields they care about.
We define their industry taxonomies so we can properly categorize accounts with web scraping and AI prompts
We determine if those fields exist in their CRM and with what data type. That helps Clay output the exact data they need to enrich their records.
This model is defined once in a Google Sheet, before we build an account scoring table template
We take a sample list of companies from the client and run it through the account scoring model. Usually, the sample list includes some of their customers, so the client knows whether the answers are accurate.
Once this is done, the account scoring model can be re-used for every new list provided by the client. Whether it's cleaning up records in their CRM or sourcing totally net-new account lists from a prospecting tool like Apollo and cleaning up the raw list so the final output contains qualified accounts, this template can be reused over and over again to surface qualified accounts without all of the manual research.
Surface relevant contacts at qualified accounts
Once you have a qualified account list, it's time to find the right contacts at those accounts for outreach.
To do this, historically, sales reps had to manually check LinkedIn company pages, and search for profiles with specific job title keywords.
Then, they’d have to manually scrape those people into a spreadsheet, or manually click on an "enrich contact" button from a data enrichment Chrome extension like Apollo or Lusha.
To be fair, there are some (slightly) more automated solutions on the market to take a list of companies and return a list of contacts. For example, with Apollo, you can import a list of accounts and surface contacts at those accounts with specific job title keyword matches.
This feature is nice, but it still falls short compared to Clay for several reasons:
First, it’s still manual. Reps or outbound leaders still need to manually import and export CSVs, which is time-consuming and so not 2024. With Clay, you can configure your contact search once and automatically surface relevant contacts at qualified accounts, fully enrich those contacts, and push them to your CRM without manually uploading and downloading CSVs or mapping fields every time.
Second, it lacks granular search results. You're limited to mostly job title keyword matches. With Clay, you can go far beyond job title matches, filtering with data points like time in seat, keywords in their LinkedIn bio, etc.
Once you have your account scoring model built and contact search configured, you can take a raw list of companies, and generate a list of qualified accounts in seconds, complete with a list of target contacts at those accounts, segmented by seniority, department, etc.
Enrich your contact data with Clay waterfalls
Unlike other data platforms, which limit users to just one provider's data and often have low coverage, Clay unlocks data waterfalls to maximize coverage and minimize cost.
Clay buys data from over 75+ different data providers in bulk and passes on the discounts of economies of scale to you without making you sign a single contract to access the data.
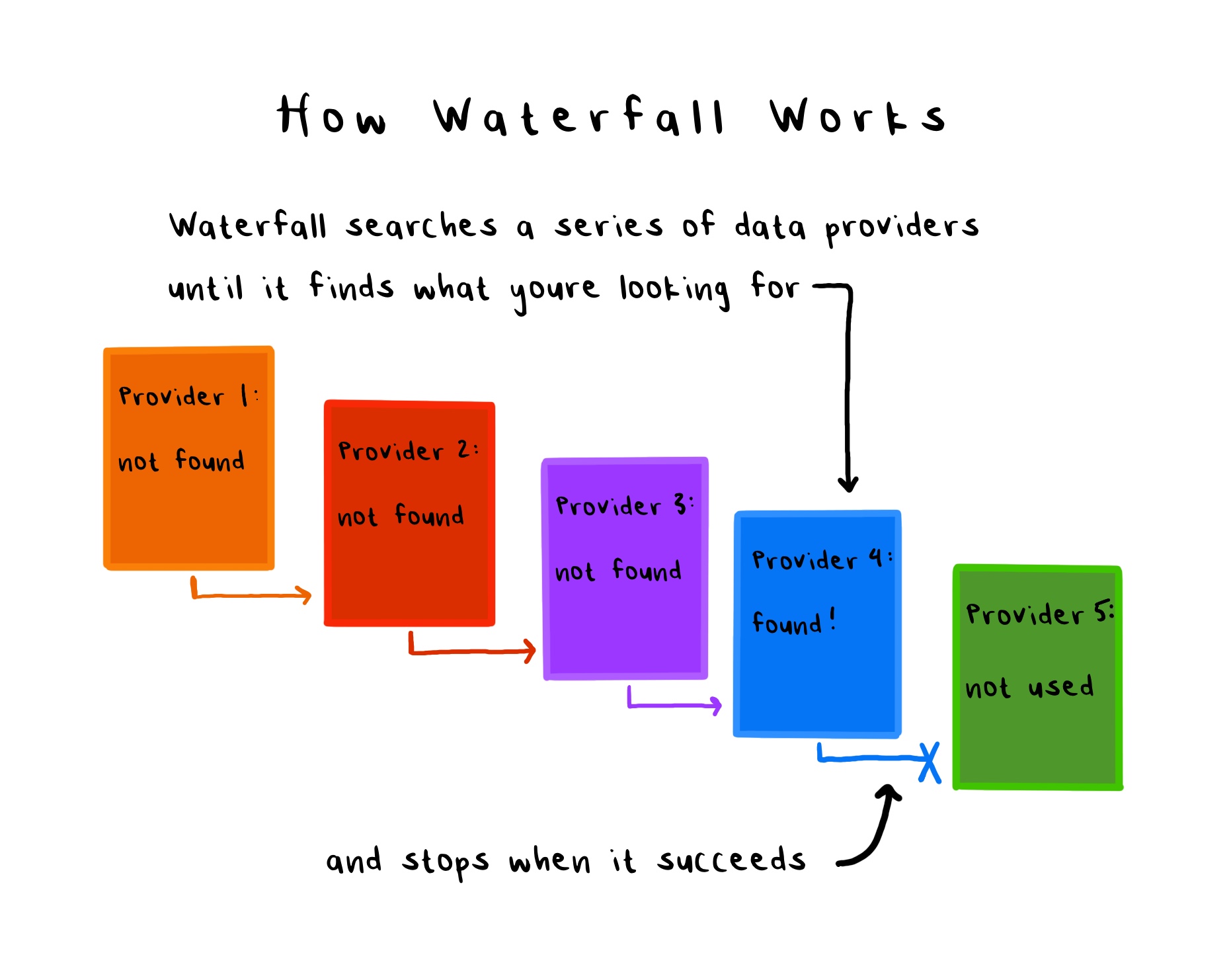
For example, you can check up to 10 data providers to find a contact's email. If one data provider can't find a match, Clay checks the next provider, and the next provider, etc. until a match is found. You only pay when you find a match.
You can use Clay credits for these enrichments or buy a license with each provider and connect them to Clay via API. Either way, you're maximizing coverage and minimizing cost.
You can do this with work or personal emails, along with mobile or company phone numbers.
I often achieve 80-90% coverage when I want to enrich a contact list with emails using Clay data waterfalls, compared to 20-30% coverage from any provider alone.
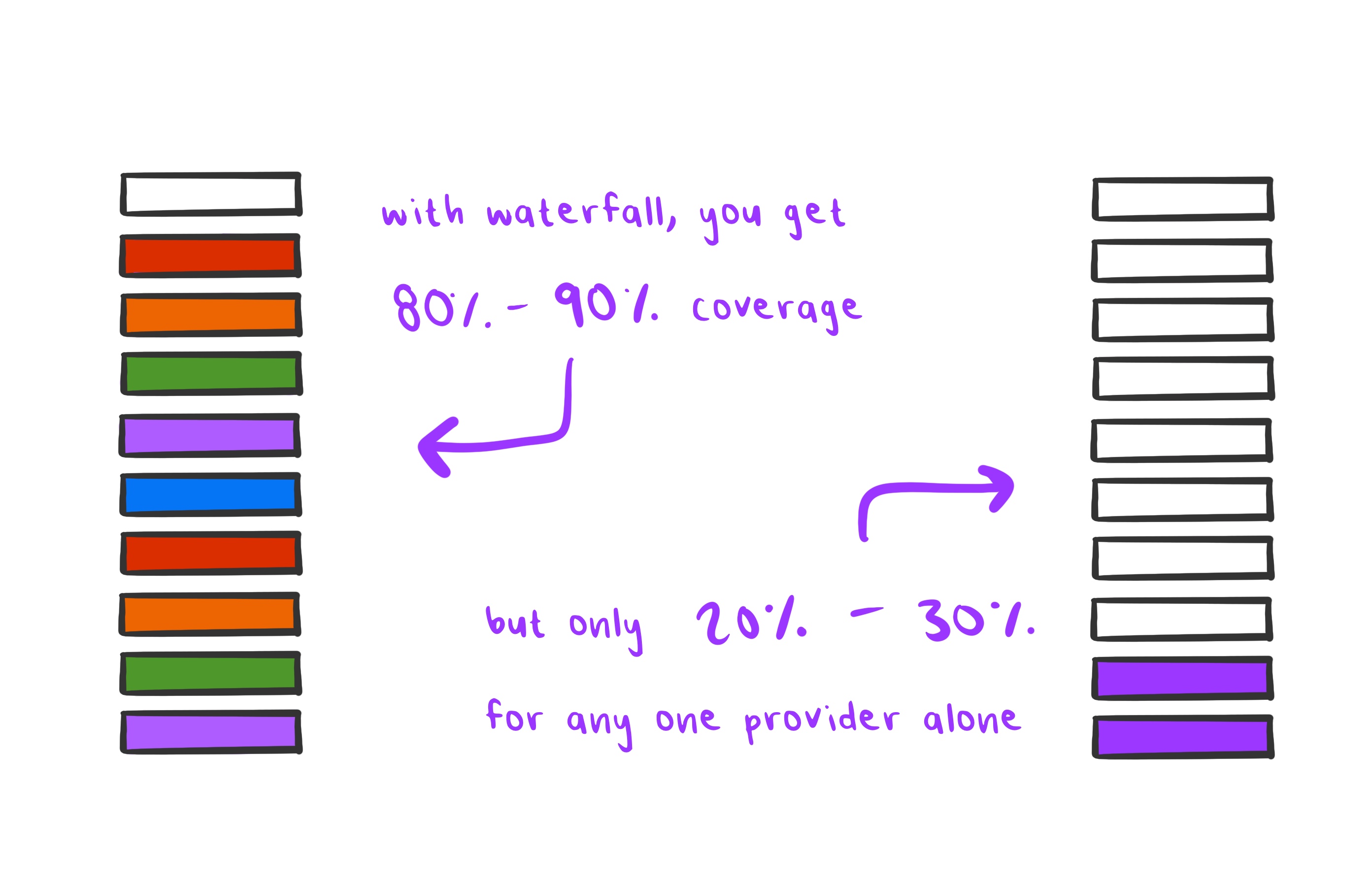
We've run tests comparing Clay coverage to standalone platforms. You can see the work email provider comparison we ran linked above. We have a mobile provider and personal email provider test coming soon.
Personalize messages for every contact
Historically, to send an email they were proud of, an SDR had to take 5-10 minutes per prospect to combine their account and lead research to craft a personalized message.
The messaging usually contained a mix of observations about the target contact’s account qualifiers (why they're a good fit) and personal notes about the prospect to add human touch.
Before AI, this was an entirely manual process. You could take specific data points about a prospect and insert them in a dynamic variable field in an outreach sequence, but it was hard to automate emails that looked handwritten.
Most people resorted to standard personalization fields like company name, a broad (often inaccurate) industry field, etc. Opening line email templates looked something like this:
Hey {first name}, it looks like {Company name} is in the {restaurant business}.
The output looked something like this:
Hey John, it looks like McDonalds is in the restaurant business.
Not too compelling if you ask me.
First of all, it's evident to most that this email was automated. But even if that's not obvious, the copy isn't compelling.
The cold email framework I like to follow goes something like this:
Hey {name},
{Observation about you/why I'm reaching out}
{Insight from that observation/value proposition}
{Soft CTA}
The opening line of your cold email should be all about them. It should make an observation about the prospect, showing that you know them, you did your research, and you're reaching out for a good reason.
Since you’ve already pulled tons of detailed account qualification data in our previous steps, you now have plenty of information to include in your opening line about “why you reached out.” You can even use a GPT prompt to create relevant opening lines.
For example, for a client that helps their customers (like 7-11) track reviews at the corporate level, we scraped 20 store locations to look for bad reviews and categorize them by sentiment.
In the opening of our email, we mentioned how we checked 20 of their locations and found 100 negative reviews, 50 of which were not responded to, and 80% of the bad reviews were about dirty bathrooms.
How’s that for a relevant and personalized opening line?
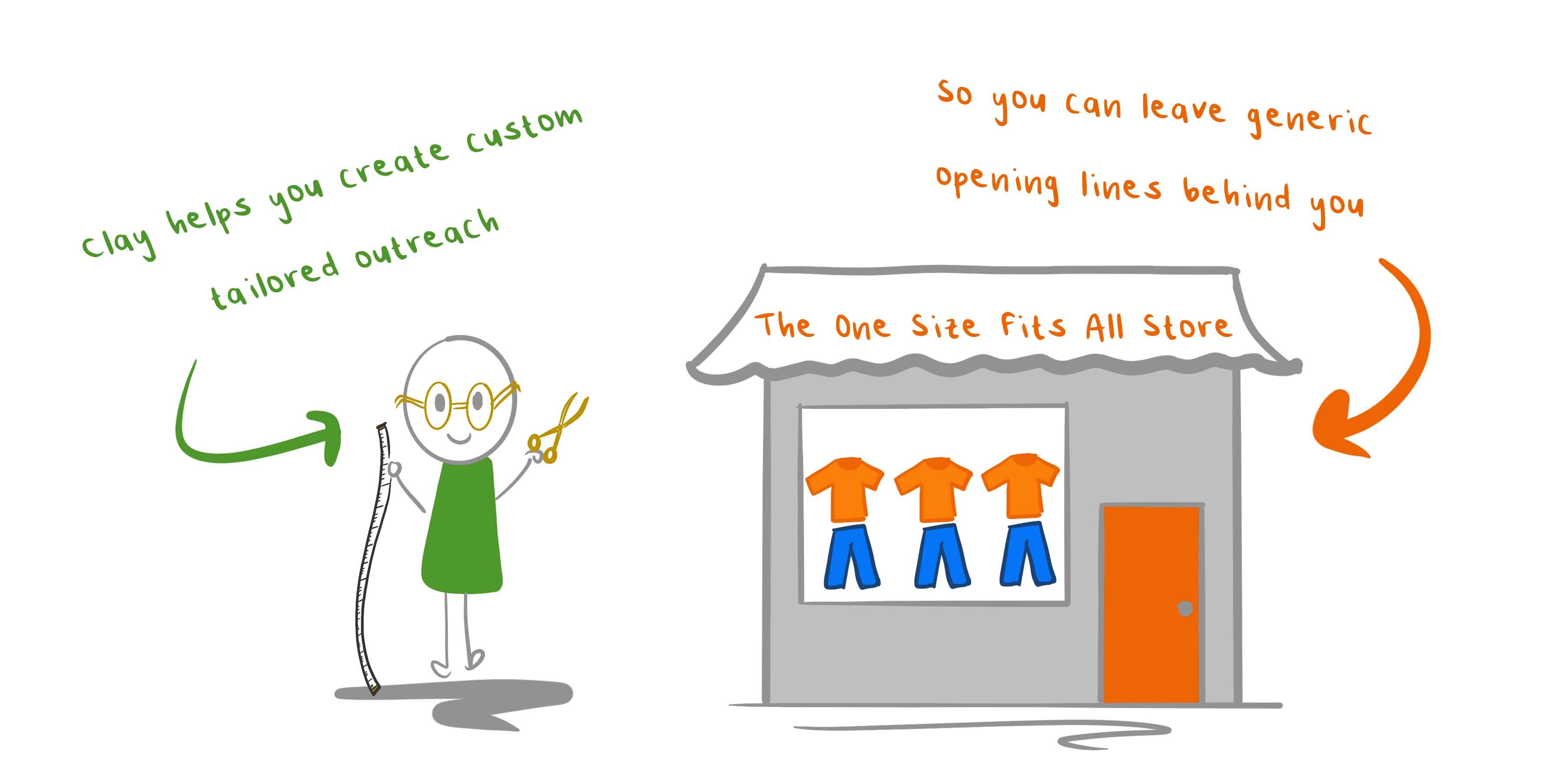
You can also grab personal data from someone’s LinkedIn and other social media to create personalized lines (by combining that data with a clever AI prompt).
For example, I have a recruiting email that works like magic, courtesy of Batman. I recently emailed 950 candidates and had a 17% reply rate (~150 replies and 80 leads for the job) using this strategy.
I enriched them with LinkedIn profile data and figured out where they live, where they work, their role, and how long they’ve been in that role. Then, I created personalized opening lines that look handwritten:
For more info on this recruiting strategy, check out the AI for Recruiting guide I wrote on Clay’s blog.
Now, you’re automating outreach emails like crazy.
Measure and iterate your campaign performance
Once your campaigns are live, it’s time to measure performance and iterate.
An in-depth explanation of this step would require a dedicated post, as iteration is both an art and a science.
In terms of projected performance, clients often ask me during sales calls about “the expected performance from their Clay campaigns.”
The truth is it depends.
It depends on your target market, your offer, how well you build your account and lead lists, your offer, copy, etc.
That said, I always tell my prospects that whatever they’re doing now, Clay can do it faster, cheaper, and more scalable than their current system. And, if they play their cards right, better performance.
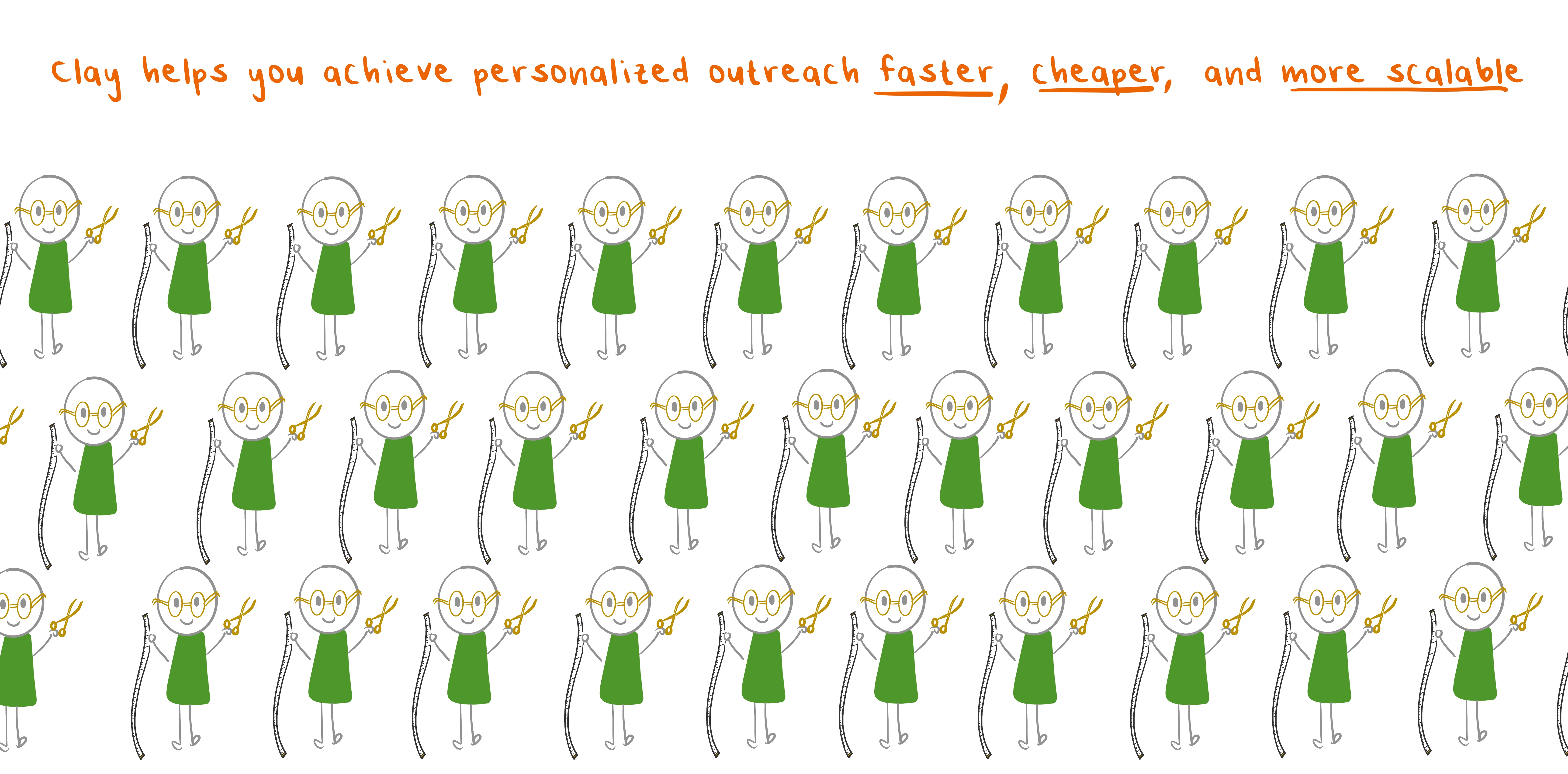
Theoretically, if you target better accounts, with more personalized messaging (thanks to Clay data), you should have higher conversion rates at all funnel stages.
It’s not a guarantee, but it’s probable.
And when you’re automating all your account and prospect research on top of rock-solid personalization, you’ll be able to scale your outbound further than before—with lower costs and better performance.
After running Clay-powered outbound ops for leading GTM teams like Sendoso, I’ve developed a playbook I want to share with you.
I call it the “AI Outbound Sales Funnel,” and it has 5 steps. We’re going to break them down in this post:
- Scoring accounts
- Surfacing the right contacts
- Personalizing outbound with account-level data
- Scaling personalized outbound emails
- Measuring and iterating

Before we dive in, you’re probably wondering why you should listen to me. I run Sculpted, a done-for-you Clay agency (Claygency). We power the outbound motion for leading SaaS companies like Sendoso, sending hundreds of thousands of emails a month.
I’ve emailed and called way more strangers than you could think of for over a decade. Here are some outbound motions I’ve run at several different companies:
- Founding GTM hire at ServiceBell, where I took the outbound motion from 0-1 and hired SDRs
- Full-cycle sales at an agency, using cold email to source and close $1mm+
- Client acquisition outreach for my first agency as a college dropout
- Outreach to fulfill client campaigns for backlink outreach
- Backlink outreach for Easy PC (my first online business in high school)
As an SDR myself, an SDR leader, and now working with leading SDR teams, I know a thing or two about outreach. I've seen winning outbound teams follow a five-step process.
In this post, I will walk through the process, step-by-step.
You'll see what we do, why we do it, and how you can automate the motion with Clay from end to end.
Don’t even start your outbound campaign unless you have a good offer
No matter how clever your copy is, your outbound campaign will only succeed if you nail your target market and offer.
In other words, you can't replace a valuable product or service with clever copywriting.
Poor product market fit is one of the main reasons why I turn down new clients. They want me to fix their outbound problem with clever email copy.
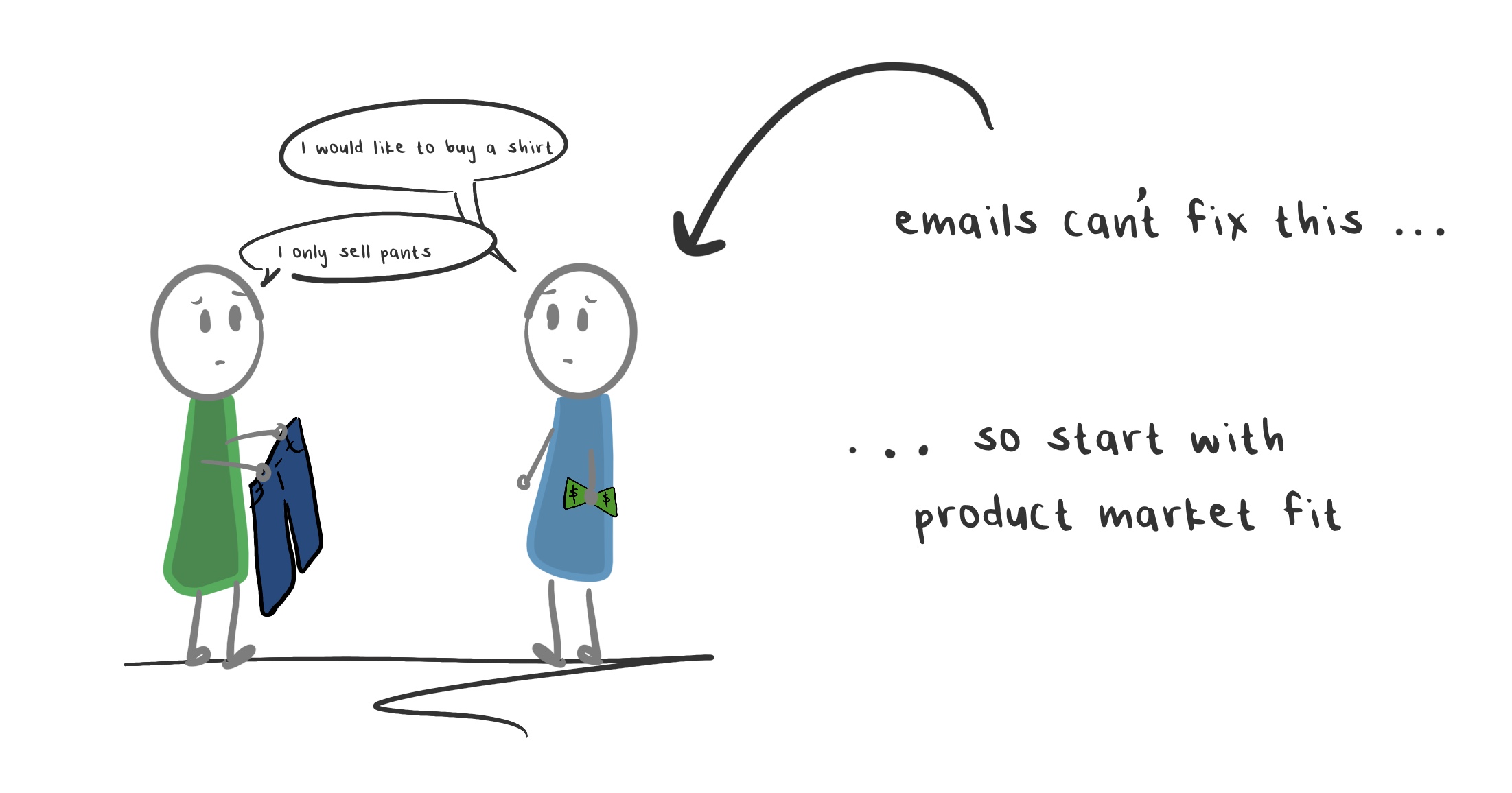
While poor copy does impact outbound conversion rates, the final decision is ultimately based on product market fit. Without good product market fit, no email sequence I can develop will replace or make up for a lousy offer.
To do account scoring the right way, get really specific
Account scoring starts with defining the problems you solve for your customers. Be specific and think through the symptoms of pain you can "spot in the wild.”
These are the signals you’ll use in your account scoring process to determine whether a company is a good fit.
(Why is it important to reach out to good-fit companies? This is probably self-explanatory, but they have problems you can solve and will be more enticed to take a meeting—and then more likely to turn into qualified pipeline.)
When most people think of symptoms, they use extremely basic data points. This is a huge missed opportunity considering Clay's power for problem-sniffing.
These are the standard fields I see folks using almost all the time:
- Employee count (from ZoomInfo or similar)
- Broad (and often incorrect) industry category
- Tech stack (from BuiltWith, often inaccurate)
These leave a ton to be desired. There are many more detailed data points you could use to score accounts. For example, you can obtain all of the below data points automatically on Clay:
- Scrape websites to look for specific keywords
- Infer specific industry categories that you define with LinkedIn company description + AI
- Look for open jobs that signal a company has a relevant problem. Scrape the job description and use AI to infer current pains
- Recent hires or fires
- Employee headcount by job title
- Employee headcount growth (overall or by department)
- Look for tech stack in their website code or job descriptions
- Score accounts by website traffic amount with SEMRush
- Funding round data
- Online reviews from websites like TrustPilot, Google Maps, etc.
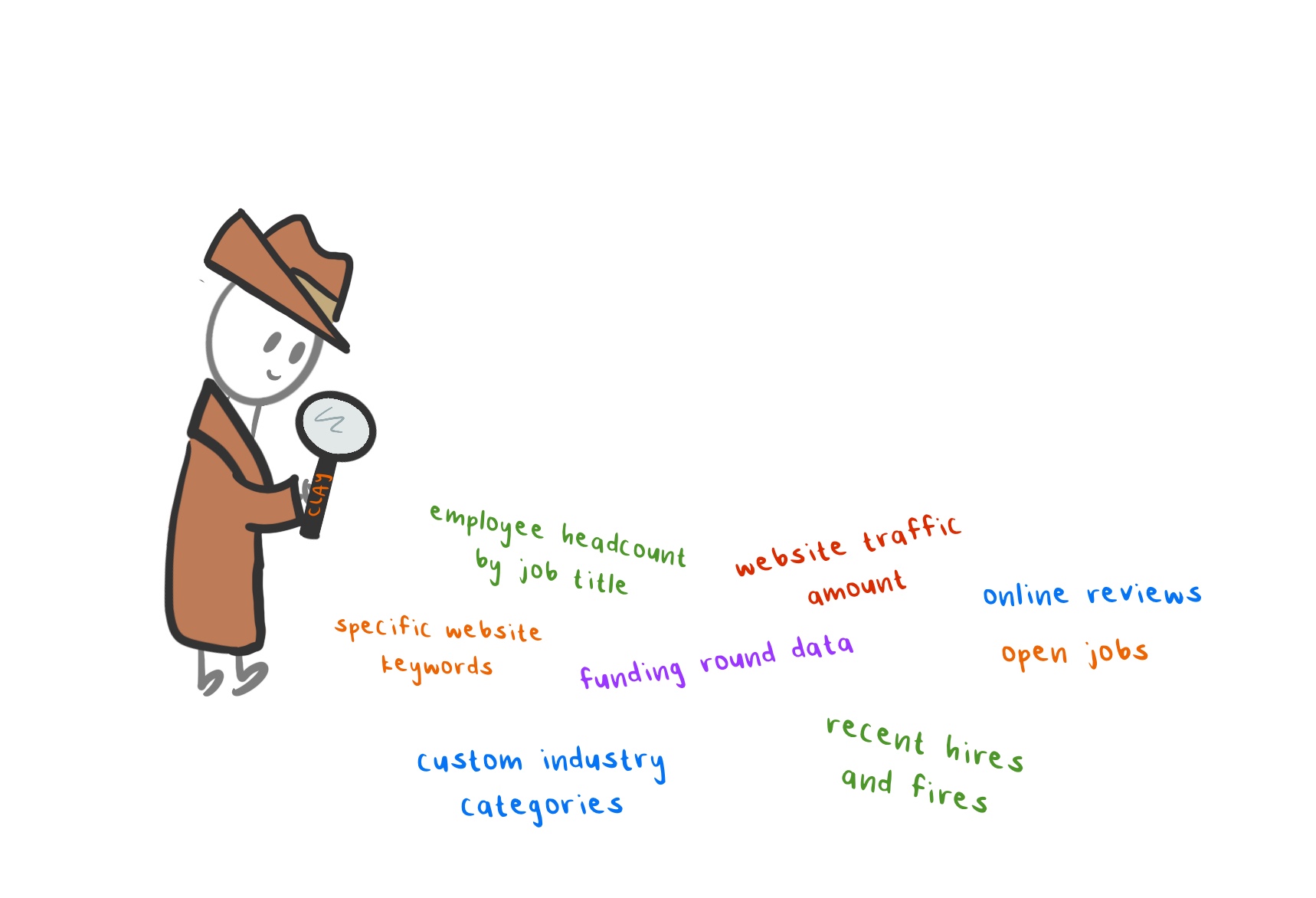
My previous, painful, manual way of account scoring
As a sales rep for ServiceBell, I wanted to find B2B SaaS companies running on HubSpot, with at least a few SDRs manning live support chat, and ideally a substantial amount of website traffic (which implied a good inbound leads.)
I'd use the following manual steps.
- Filter a list of companies using the "Computer Software'' industry filter on LinkedIn Sales Navigator
- Check the company LinkedIn and website to confirm they're a SaaS company and not a software development agency
- Look at the LinkedIn company page and manually search through their employees to look for salespeople on staff
- Check the website source code to look for the HubSpot scripts
- Check the website to see if they had a pricing page (which would be a good place for ServiceBell to live) and understand if they sold to engineering, security, or legal titles. (Companies who sold to any of those personas weren't usually a good fit for ServiceBell.)
- Lastly, plug their website into Ahrefs for SEMRush to see how much website traffic they have, to get a feeler for total inbound lead volume.
After all was said and done, I had a solid account, but that was after tons of manual research—at least ten minutes per qualified account.
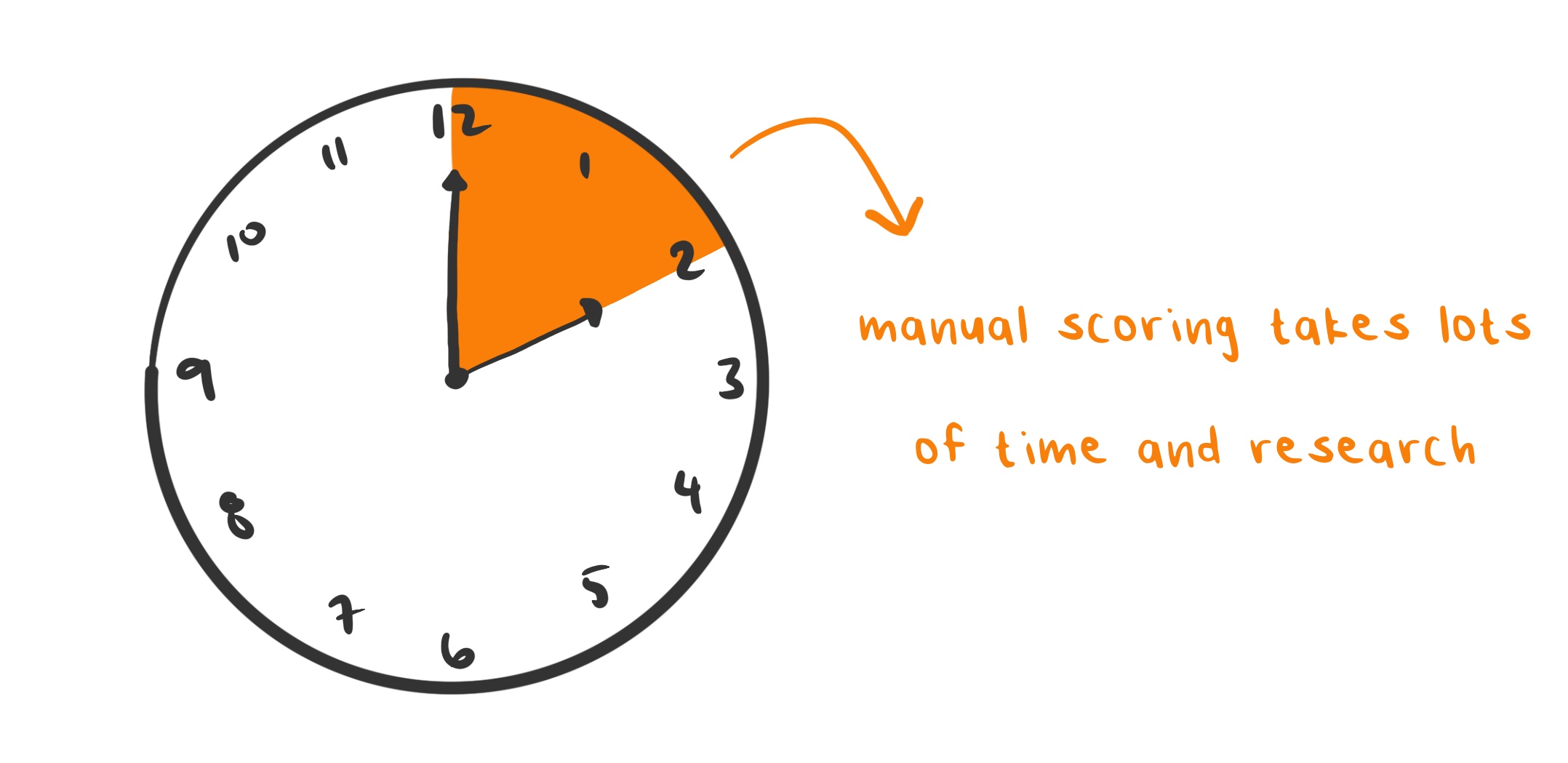
If I was working non-stop all day, I could find 48 qualified accounts. Of course, nobody works for 8 hours straight (lunch, bathroom, break, etc.) so the total number of accounts I could find in one day drops down to less than 48 daily.
This is not productive. At this point, I still hadn’t found the right contacts or their emails or sent any personalized outreach messages.
Sure, you could hire a VA or another service to do account research…but good VAs are hard to come by, need training, and are still limited by hours in the day. They are slower, less reliable, and more expensive than an automated solution like Clay.
Manual account scoring sucks—so most reps don’t even bother
Since the account scoring process is so tedious, most reps skip this step and opt for volume instead to show their manager "how much activity they're doing" daily despite the lackluster downstream results.
They pull a list from their prospecting tool, whether it's ZoomInfo, Sales Nav, etc., and call it a day. Their resultant account list is a mix of employee count and an often incorrect industry categorization, since huge companies like ZoomInfo can't possibly categorize accounts the same way you do.
Here’s the problem: your definition of your ICP is unique, and can't possibly be serviced by an "out-of-the-box" solution like ZoomInfo.
You either need to manually research the accounts or suffer from broad lists full of mostly junk accounts that wastes your team's time and guarantee that your booked meetings don’t turn into qualified pipeline.
How to automate your manual account scoring
With Clay, you can set up an account scoring model once, and automatically turn a raw list of company names or domains into a fully enriched account list, complete with all of the data that was previously only possible to find with a manual research process.
You can build lists of companies that are a good fit for your service, instead of blasting to unqualified accounts or spending too much time (i.e. any time) on manual research.
Here’s how I do it when I onboard new customers:
I understand how they'd define a "dream account".
I ask them to define the specific account-level qualification fields they care about.
We define their industry taxonomies so we can properly categorize accounts with web scraping and AI prompts
We determine if those fields exist in their CRM and with what data type. That helps Clay output the exact data they need to enrich their records.
This model is defined once in a Google Sheet, before we build an account scoring table template
We take a sample list of companies from the client and run it through the account scoring model. Usually, the sample list includes some of their customers, so the client knows whether the answers are accurate.
Once this is done, the account scoring model can be re-used for every new list provided by the client. Whether it's cleaning up records in their CRM or sourcing totally net-new account lists from a prospecting tool like Apollo and cleaning up the raw list so the final output contains qualified accounts, this template can be reused over and over again to surface qualified accounts without all of the manual research.
Surface relevant contacts at qualified accounts
Once you have a qualified account list, it's time to find the right contacts at those accounts for outreach.
To do this, historically, sales reps had to manually check LinkedIn company pages, and search for profiles with specific job title keywords.
Then, they’d have to manually scrape those people into a spreadsheet, or manually click on an "enrich contact" button from a data enrichment Chrome extension like Apollo or Lusha.
To be fair, there are some (slightly) more automated solutions on the market to take a list of companies and return a list of contacts. For example, with Apollo, you can import a list of accounts and surface contacts at those accounts with specific job title keyword matches.
This feature is nice, but it still falls short compared to Clay for several reasons:
First, it’s still manual. Reps or outbound leaders still need to manually import and export CSVs, which is time-consuming and so not 2024. With Clay, you can configure your contact search once and automatically surface relevant contacts at qualified accounts, fully enrich those contacts, and push them to your CRM without manually uploading and downloading CSVs or mapping fields every time.
Second, it lacks granular search results. You're limited to mostly job title keyword matches. With Clay, you can go far beyond job title matches, filtering with data points like time in seat, keywords in their LinkedIn bio, etc.
Once you have your account scoring model built and contact search configured, you can take a raw list of companies, and generate a list of qualified accounts in seconds, complete with a list of target contacts at those accounts, segmented by seniority, department, etc.
Enrich your contact data with Clay waterfalls
Unlike other data platforms, which limit users to just one provider's data and often have low coverage, Clay unlocks data waterfalls to maximize coverage and minimize cost.
Clay buys data from over 75+ different data providers in bulk and passes on the discounts of economies of scale to you without making you sign a single contract to access the data.
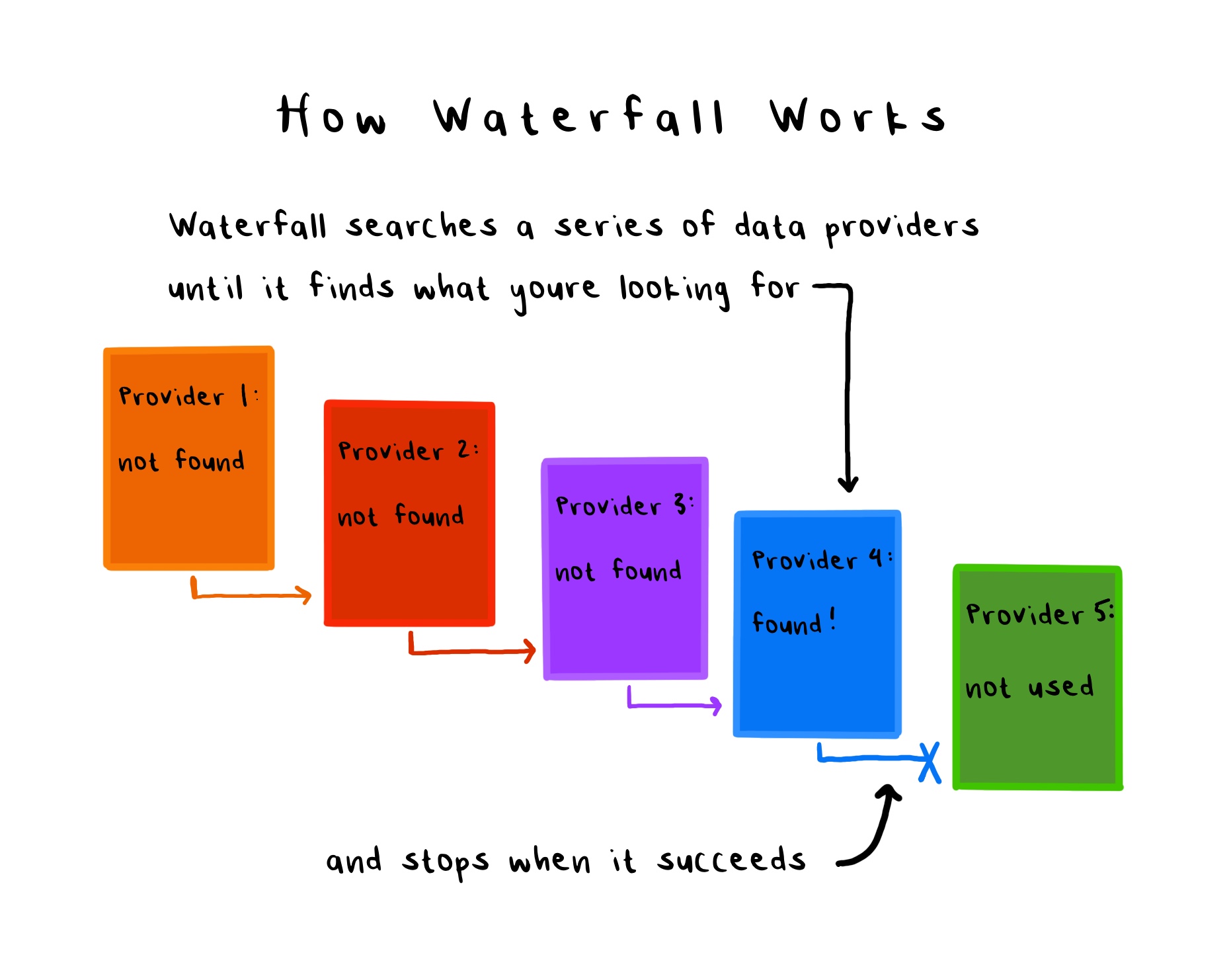
For example, you can check up to 10 data providers to find a contact's email. If one data provider can't find a match, Clay checks the next provider, and the next provider, etc. until a match is found. You only pay when you find a match.
You can use Clay credits for these enrichments or buy a license with each provider and connect them to Clay via API. Either way, you're maximizing coverage and minimizing cost.
You can do this with work or personal emails, along with mobile or company phone numbers.
I often achieve 80-90% coverage when I want to enrich a contact list with emails using Clay data waterfalls, compared to 20-30% coverage from any provider alone.
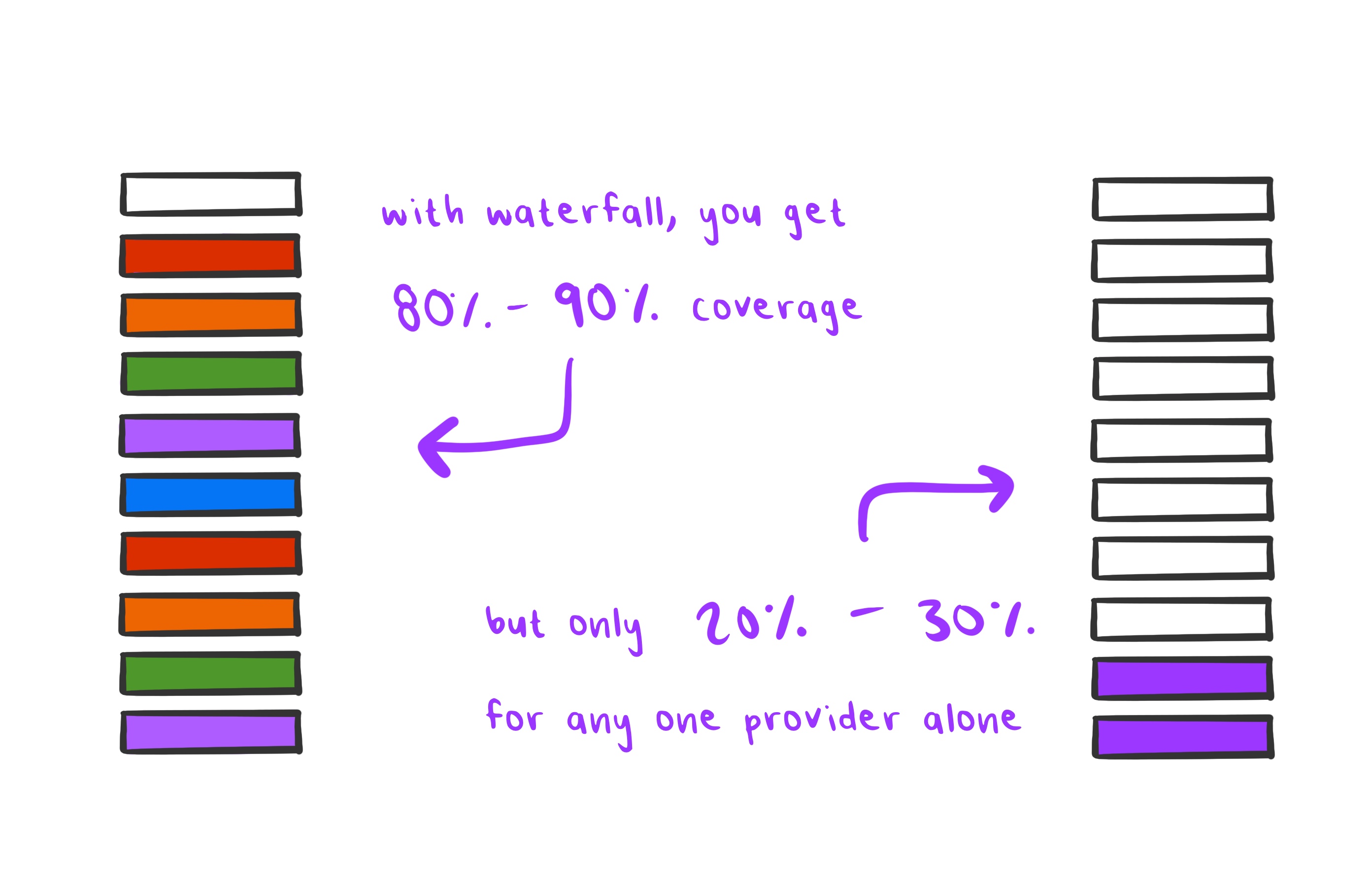
We've run tests comparing Clay coverage to standalone platforms. You can see the work email provider comparison we ran linked above. We have a mobile provider and personal email provider test coming soon.
Personalize messages for every contact
Historically, to send an email they were proud of, an SDR had to take 5-10 minutes per prospect to combine their account and lead research to craft a personalized message.
The messaging usually contained a mix of observations about the target contact’s account qualifiers (why they're a good fit) and personal notes about the prospect to add human touch.
Before AI, this was an entirely manual process. You could take specific data points about a prospect and insert them in a dynamic variable field in an outreach sequence, but it was hard to automate emails that looked handwritten.
Most people resorted to standard personalization fields like company name, a broad (often inaccurate) industry field, etc. Opening line email templates looked something like this:
Hey {first name}, it looks like {Company name} is in the {restaurant business}.
The output looked something like this:
Hey John, it looks like McDonalds is in the restaurant business.
Not too compelling if you ask me.
First of all, it's evident to most that this email was automated. But even if that's not obvious, the copy isn't compelling.
The cold email framework I like to follow goes something like this:
Hey {name},
{Observation about you/why I'm reaching out}
{Insight from that observation/value proposition}
{Soft CTA}
The opening line of your cold email should be all about them. It should make an observation about the prospect, showing that you know them, you did your research, and you're reaching out for a good reason.
Since you’ve already pulled tons of detailed account qualification data in our previous steps, you now have plenty of information to include in your opening line about “why you reached out.” You can even use a GPT prompt to create relevant opening lines.
For example, for a client that helps their customers (like 7-11) track reviews at the corporate level, we scraped 20 store locations to look for bad reviews and categorize them by sentiment.
In the opening of our email, we mentioned how we checked 20 of their locations and found 100 negative reviews, 50 of which were not responded to, and 80% of the bad reviews were about dirty bathrooms.
How’s that for a relevant and personalized opening line?
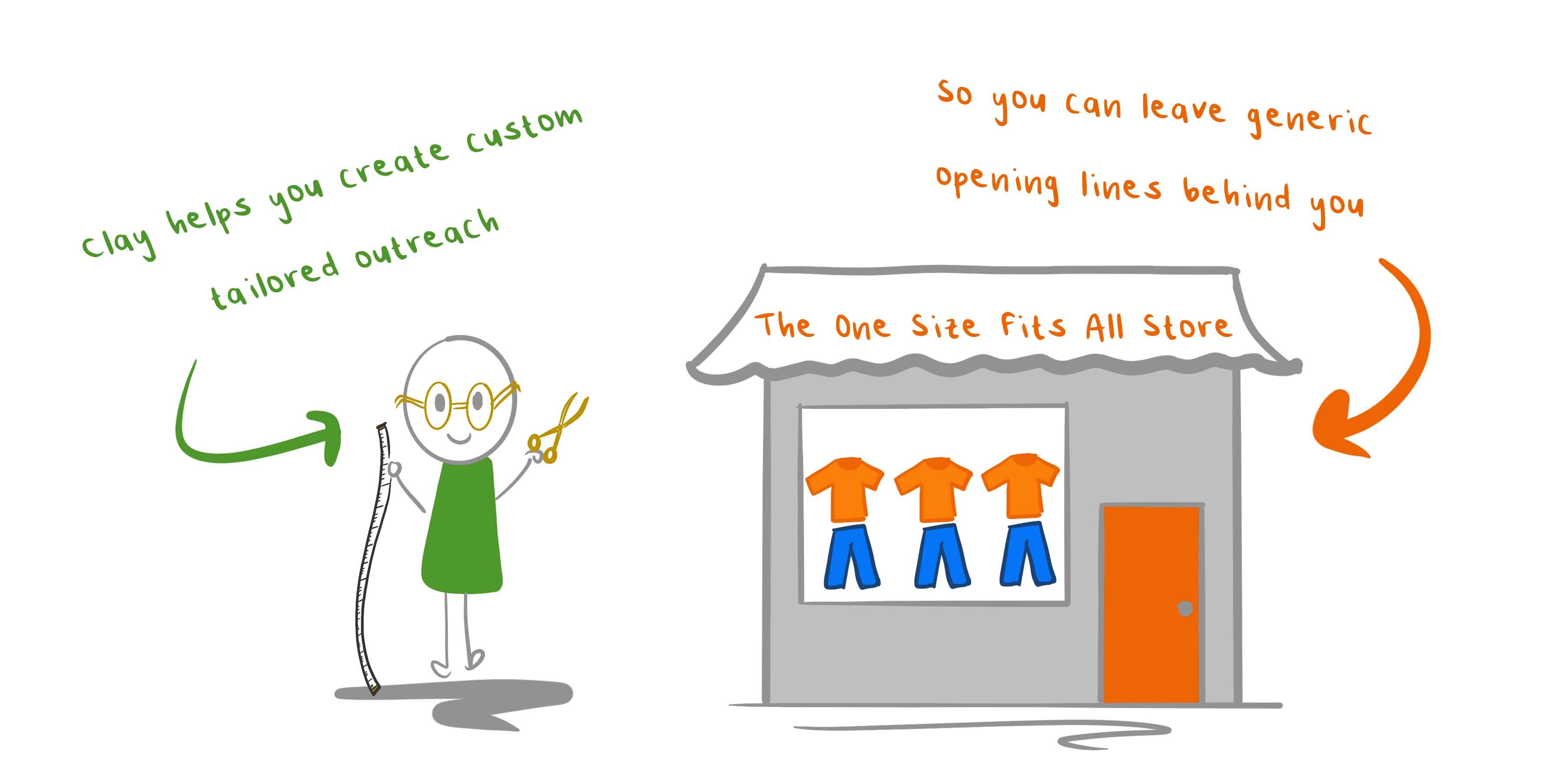
You can also grab personal data from someone’s LinkedIn and other social media to create personalized lines (by combining that data with a clever AI prompt).
For example, I have a recruiting email that works like magic, courtesy of Batman. I recently emailed 950 candidates and had a 17% reply rate (~150 replies and 80 leads for the job) using this strategy.
I enriched them with LinkedIn profile data and figured out where they live, where they work, their role, and how long they’ve been in that role. Then, I created personalized opening lines that look handwritten:
For more info on this recruiting strategy, check out the AI for Recruiting guide I wrote on Clay’s blog.
Now, you’re automating outreach emails like crazy.
Measure and iterate your campaign performance
Once your campaigns are live, it’s time to measure performance and iterate.
An in-depth explanation of this step would require a dedicated post, as iteration is both an art and a science.
In terms of projected performance, clients often ask me during sales calls about “the expected performance from their Clay campaigns.”
The truth is it depends.
It depends on your target market, your offer, how well you build your account and lead lists, your offer, copy, etc.
That said, I always tell my prospects that whatever they’re doing now, Clay can do it faster, cheaper, and more scalable than their current system. And, if they play their cards right, better performance.
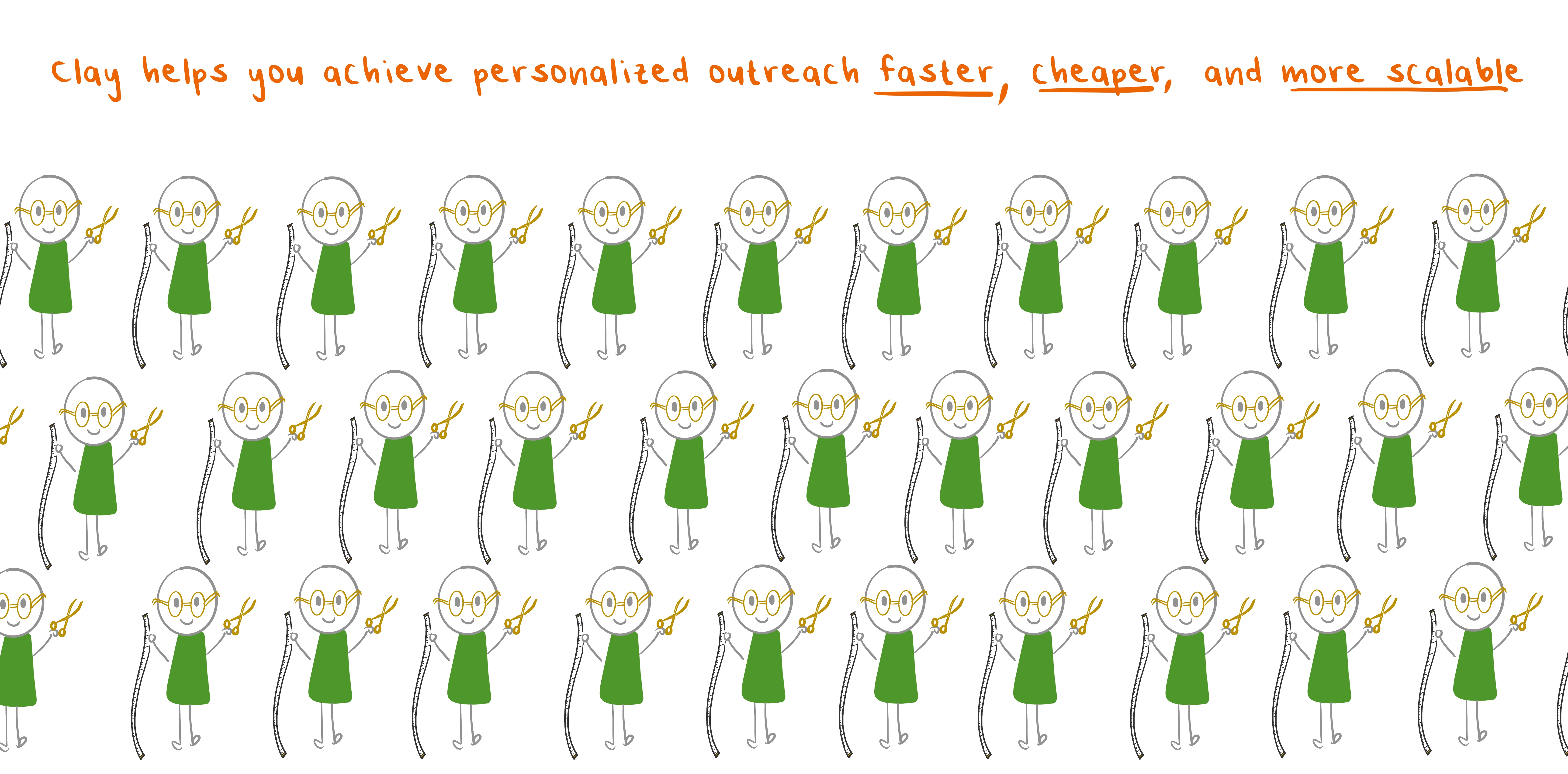
Theoretically, if you target better accounts, with more personalized messaging (thanks to Clay data), you should have higher conversion rates at all funnel stages.
It’s not a guarantee, but it’s probable.
And when you’re automating all your account and prospect research on top of rock-solid personalization, you’ll be able to scale your outbound further than before—with lower costs and better performance.