Using AI recruiting strategies can transform your recruiting process from slow, manual, and ineffective to blazingly fast—and better. Today, recruiting can feel like a lose-lose. People either spend unsustainable amounts of time on manual candidate research OR send generic messages with terrible response rates.
AI recruiting tools and workflows can shatter that tradeoff. Today, for the first time, you can research and write hyper-personalized messages that land candidates—without any manual work.
In this guide, we’re going to show you how, drawing upon the success of Mike Cohen—a.k.a the “Batman” of recruiting.
Mike routinely books calls with 33% of the candidates he emails with little to no manual work. We’ll walk through Mike’s entire end-to-end AI recruiting process, including the exact scripts and strategies he uses.
This guide includes:
- What’s wrong with the recruiting status quo
- How to use artificial intelligence to automate your manual research
- How to use artificial intelligence to personalize emails
- How to build Mike’s AI recruiting strategy in Clay, complete with email scripts and GPT prompts
The future of recruiting is intelligent and personalized at scale. Let’s dive in!
Results first: What can AI recruiting do for you?
TLDR: AI recruiting strategies will save you research time, make your emails much more personalized, and land more candidates.
AI gets beautiful when you combine your automated research with clever GPT prompts to create insanely relevant cold email copy. Using this strategy, we’ve sliced our time sending emails by more than 50%—with better results.
Here’s an AI generated email we sent with a 13% positive reply rate:
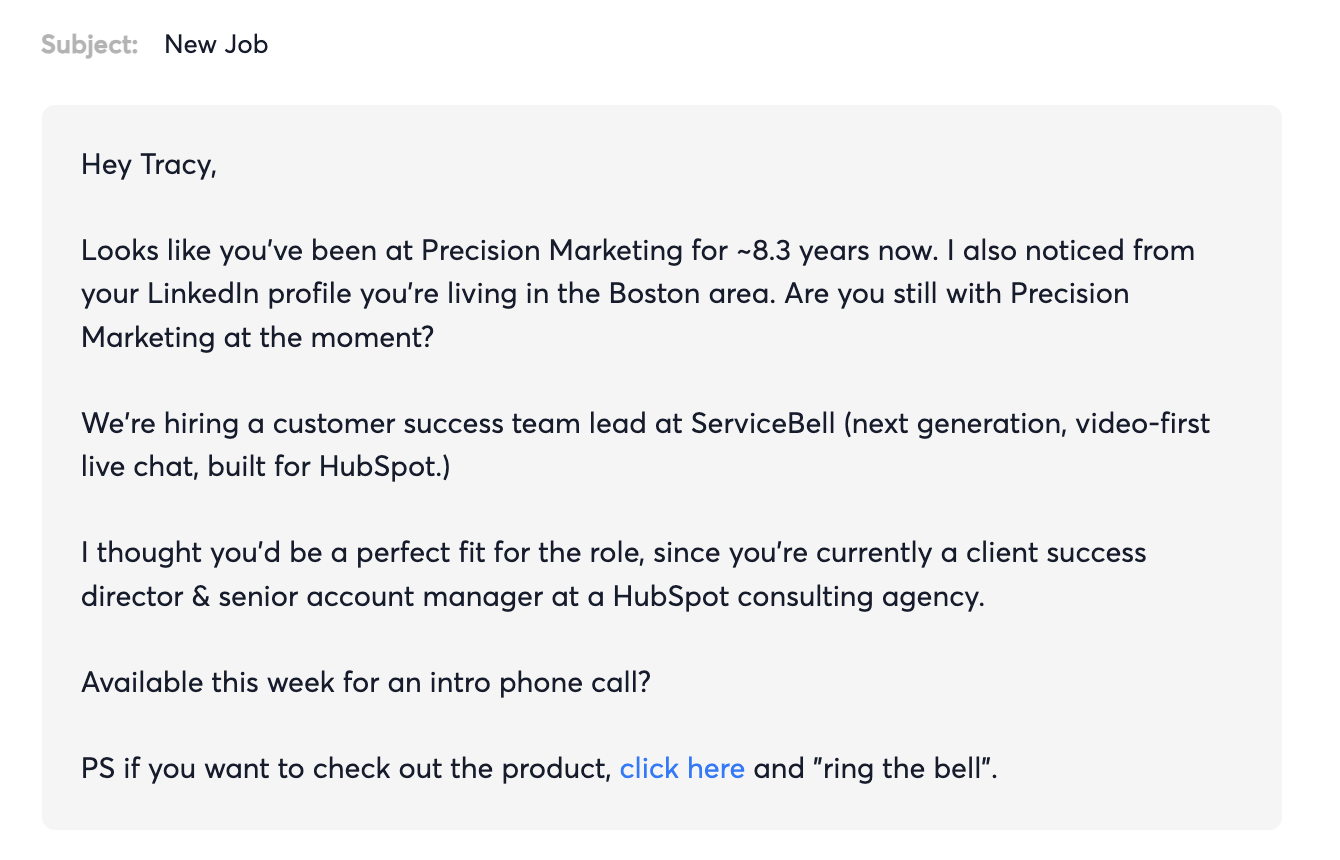
The secret to solid cold outreach is 1:1 personalization. Your candidates need to feel like the message in their inbox was written just for them.
There’s no way someone receives that email and thinks it was sent to 100 other people.
To write it, we just scraped the candidate’s title, company, location, and time in their role, and massaged the data with GPT
On the other hand, here’s one of the “best” recruiting cold email templates you’ll find on Google:

It’s totally generic, doesn’t look personalized at all, and will likely be ignored by quality candidates.
Why recruiting today is broken
There are two main parts of recruiting: first, sourcing & initial outreach, and second, continuing interactions and interviews with quality candidates.
We’ll focus our guide on sourcing, which is the first step of recruiting. Sourcing focuses exclusively on identifying and engaging the best candidates through research and outreach.
Recruiting teams today struggle with two critical issues.
No metrics or tracking
Sourcing is a nightmare to measure and track. Unless you’re terrific at spreadsheets, sourcers have zero visibility into any of the work their team is doing until the message is sent.
Because teams can’t track what outreach strategies and channels are working, they often fly blind—unable to measure performance or improve their own efficiency. And that means they use broken processes to message unqualified or uninterested candidates.
Most candidate sourcing teams, e.g., don’t know how many:
- Searches ran
- Profiles returned
- Profiles were checked
- Potential candidates were identified
- Potential candidates were messaged
- Suitable candidates replied
- Intro calls were booked
Generic, ineffective messaging
Sourcers rarely have time for detailed research on potential candidates' backgrounds, skills, and motivations. That means they lump candidates into massive, generic outreach campaigns—with messages that sound robotic and spammy.
It makes sense. Every email you’ve seen in your inbox and instantly deleted all have one thing in common: it was generic. The email wasn’t written for you. And if the sender didn’t take the time to learn about you, why would you take the time to learn about them?
Potential candidates must feel like they are getting the message from you. If candidates feel they’re getting the message because they have a specific title and some buzzwords on their profile, and the message was sent to 200 other people, they won’t respond.
Writing personalized messages to the most suitable candidates used to require tons of time and effort—until AI recruiting tools came along.
How AI Recruiting strategies work
Using AI in your recruiting workflows can help you send hyper-personalized messages at scale—and track your success with real metrics.
AI recruiting strategies can help you:
- Filter for tons of qualified candidates
- Automate all your manual research — including everything about someone education, work history, specializations, and more
- Write personalized sourcing emails at scale (with a 30% message to book rate)
Let’s go through each step to get you started with the use of AI in your recruiting process.
Step One: Automatically Find Qualified Candidates with AI
Your AI recruiting journey starts with sourcing a curated list of people to reach out to.
There are two ways to do this. Consider the below:
- Search A returned 300 people, but we only messaged 25 people.
- Search B only returned 80 people, but 35 were worth messaging
Search B is far superior—saving everyone time and money.
Filter for the right list, so your team can spend more time messaging good candidates instead of sifting through poor profiles.
Find Candidates from LinkedIn
Clay has access to LinkedIn’s entire database…which means you can run your boolean searches from Clay without spending tons of money on LinkedIn Sales Navigator.
You also don’t have to manually extract candidate data from Sales Navigator after searching or use a scraping tool. Clay gives you the data in spreadsheet form off the bat.
Sign up for Clay and click New Table > People > Find People from LinkedIn.
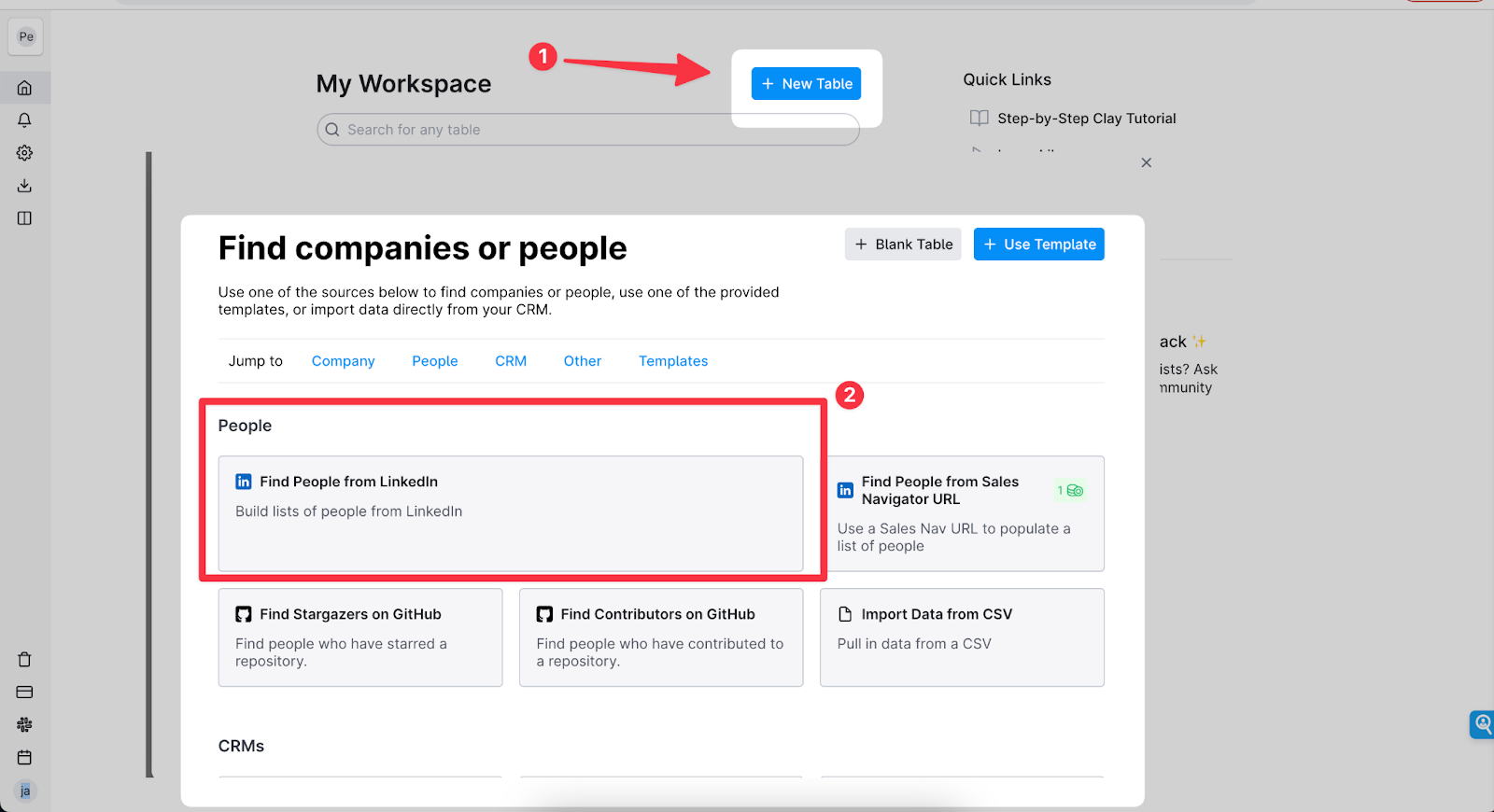
Next, enter your search criteria. In our example, we’re looking for marketing leaders with at least two years at their current company, in the San Francisco area.

You can filter by minimum or maximum months in a specific role, number of jobs, keywords in their job descriptions, keywords in their about section, and more.
Next, we’ll import our results to a New Table:
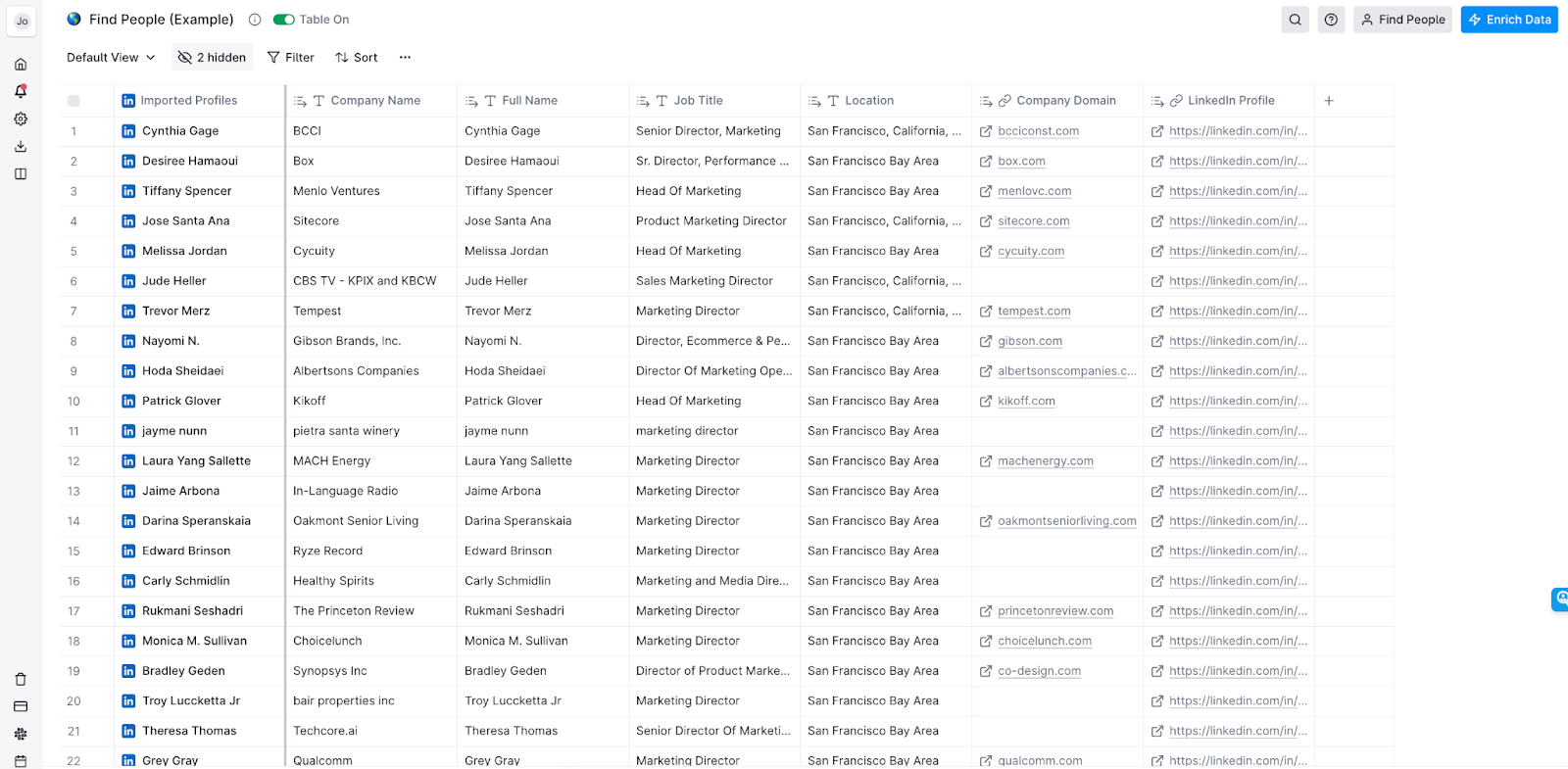
Extract Candidate Profile Data
With all of your candidates now in a Clay table, it’s time for further qualification.
Our basic find people search gives us a candidate’s company, full name, job title, location, company website, and LinkedIn profile URL.
More candidate data is available on LinkedIn, like education, current company industry and employee count, prior experience data, etc.
You can use the “Enrich Person from LinkedIn Profile” integration to unlock the extra data in your Clay table:
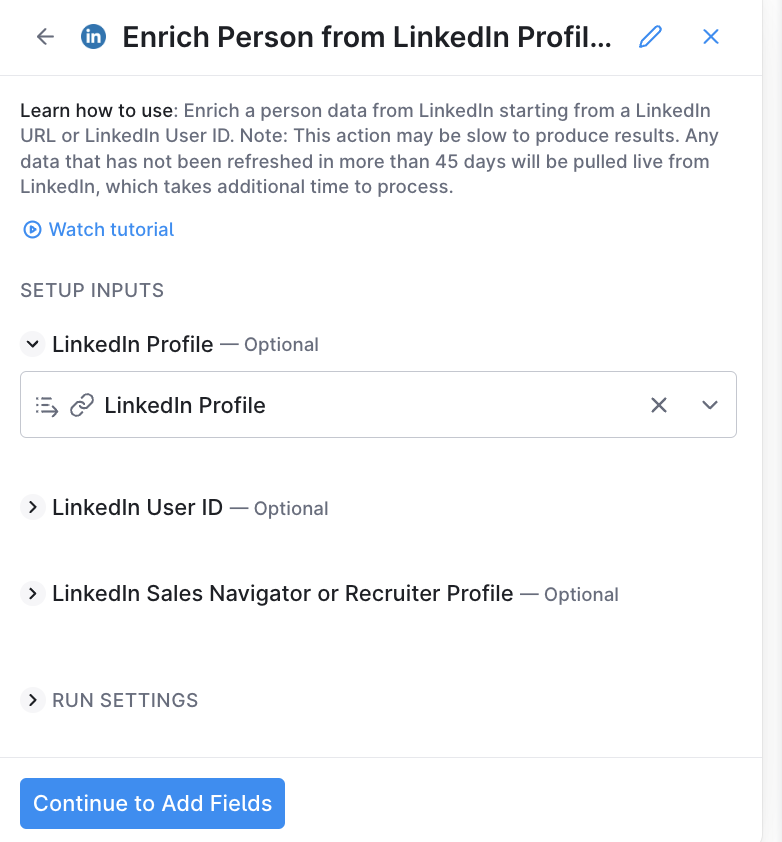
Now you have access to all of their LinkedIn data in Clay:

In this example, we want to know more about the candidate’s prior experience and education, so we’re going to pull that data out into our Clay table as individual columns.
Click on the “Enrich Person from LinkedIn” cell to open the data menu on the right, and select “Add as column” to create a new column:
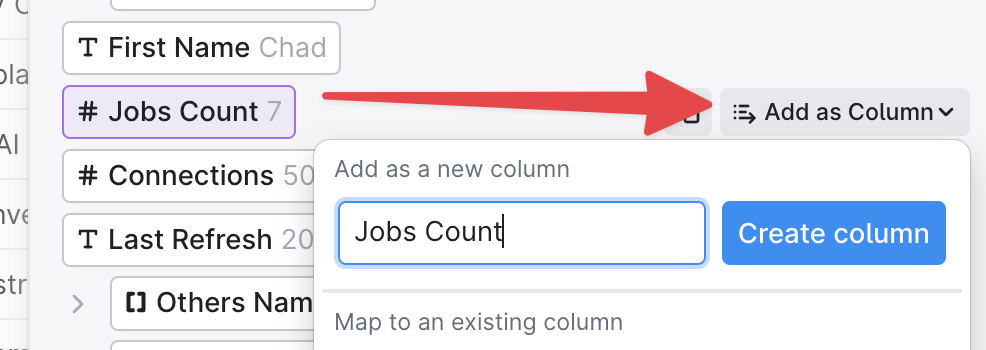
Create new columns for all the information you want in the table.
Here’s our example:
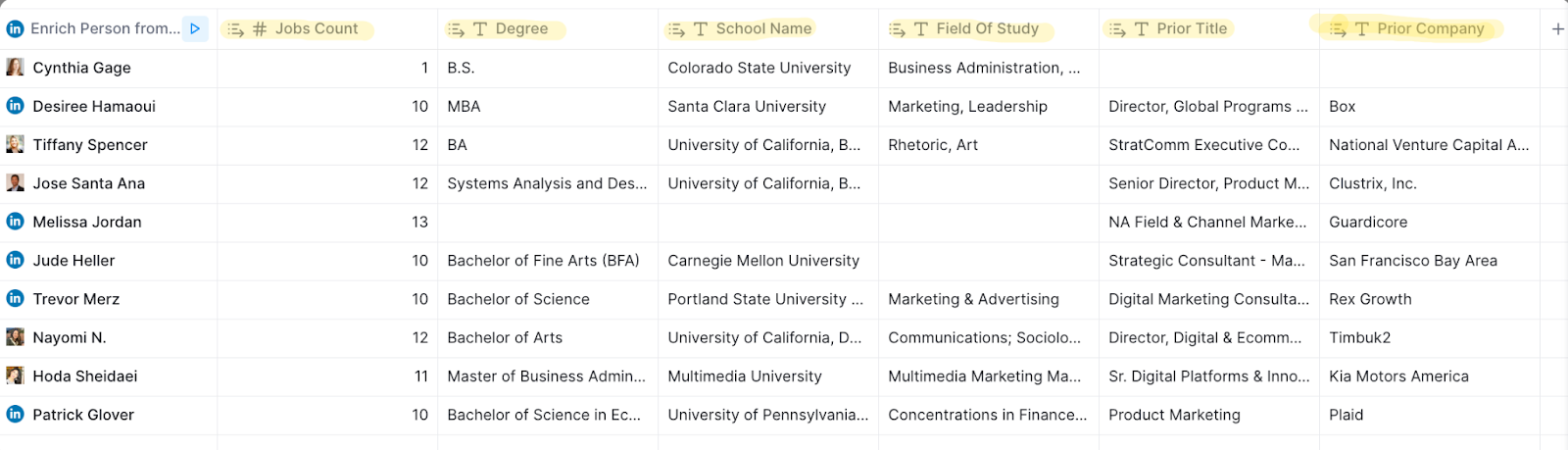
Extract Candidate Company Data
It’s often helpful to know information about your candidate’s current employer, like employee count and industry.
We can grab that information with the “Enrich Company” LinkedIn integration:
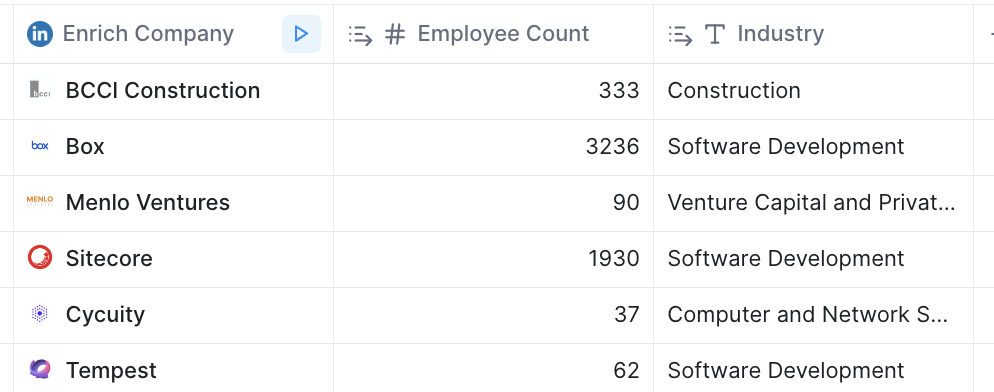
Pro Tip: AI can help determine each specific company’s industry
If you’ve used LinkedIn before, you know their industry categories are pretty broad.
In the example image above, “Tempest” and “Box” are both categorized as “Software Development” companies.
But Tempest is a “secure browser”:
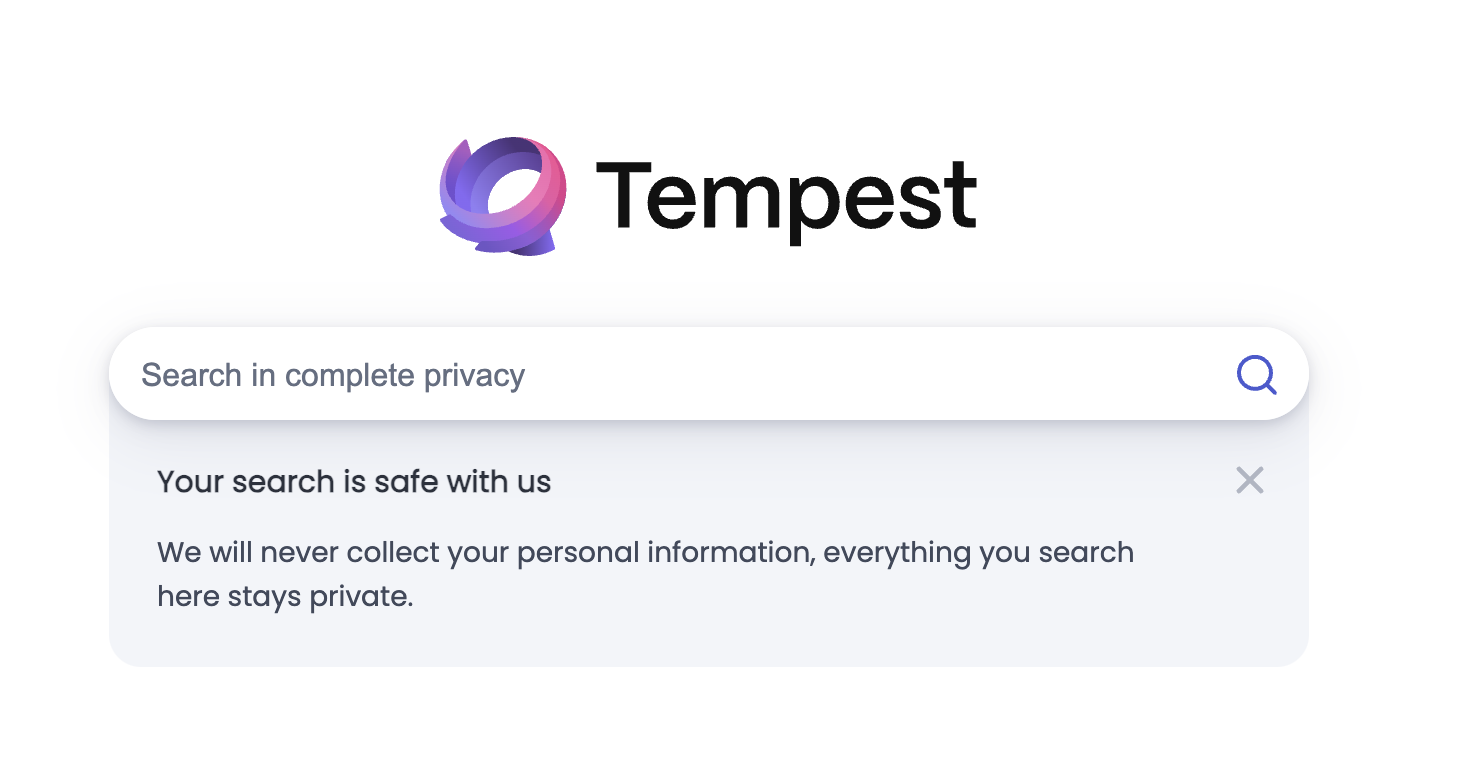
Box is a workplace collaboration software:

Moreover, custom software development companies are probably lumped together in the “Software Development” category.
If you need to categorize companies by specific company type, you must manually check each website… unless you use Clay.
With Clay, you can automate this manual research using a GPT prompt.
We’ll use the candidate’s LinkedIn company page as input, to determine the company’s specific category.
For example, check out this list of companies. Linkedin provides the first “Industry” column. The second column is our GPT prompt output:
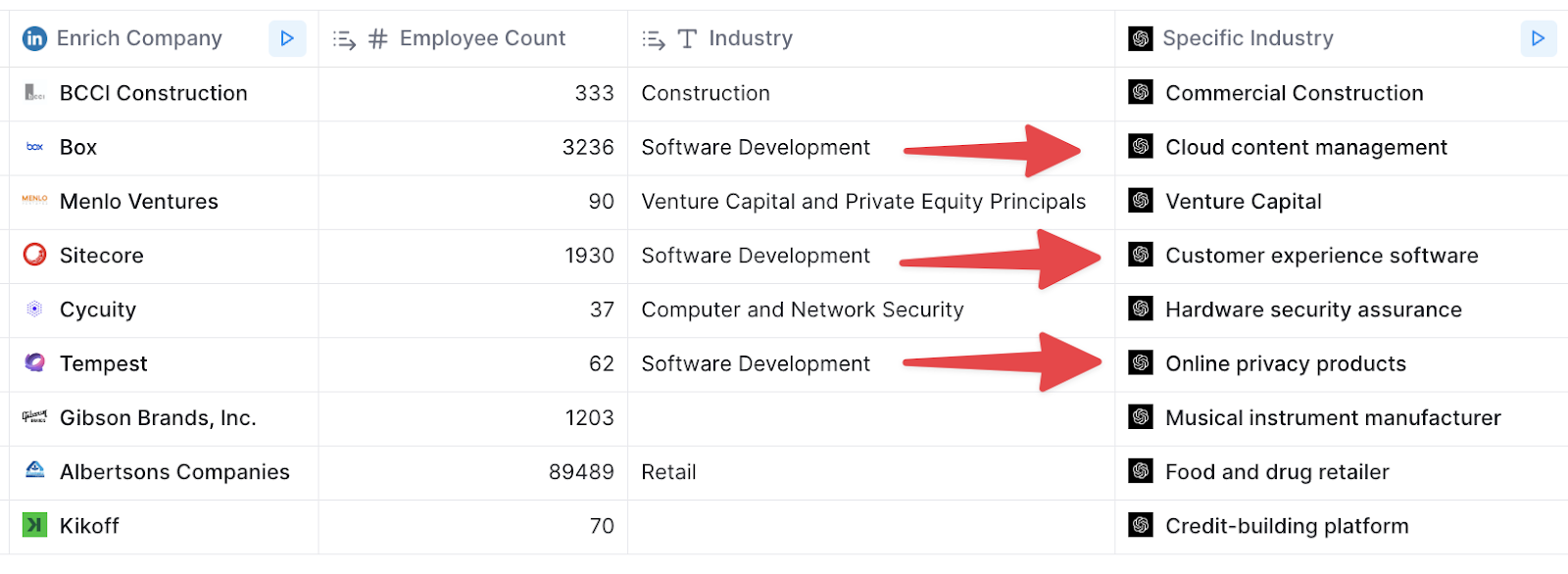
You can see how three companies, all categorized as “Software Development” had three separate specific company types.
If you wanted to be a bit more broad and qualify any candidate with prior “SaaS” experience, you could determine if any of these companies are SaaS companies instead of the specific industry.
For example:

Without manual research, we can automatically determine if a candidate has prior SaaS experience.
Pro Tip: AI can help calculate candidates’ time in current role
We also want to know how long the candidate’s been in their current role because we don’t want to message anyone who’s been in their seat for < 2 years at their current role.
“Time in seat” isn’t available by default. However, we can create an AI formula to calculate the total duration in their current seat, by subtracting the time since their start date from today’s date. Then, we’ll filter out any candidates in the seat for < 2 years.
Click on any column, then select “Insert Right” followed by “Formula” to insert a formula column in your Clay table.
Then, we’ll write the formula and generate our output.
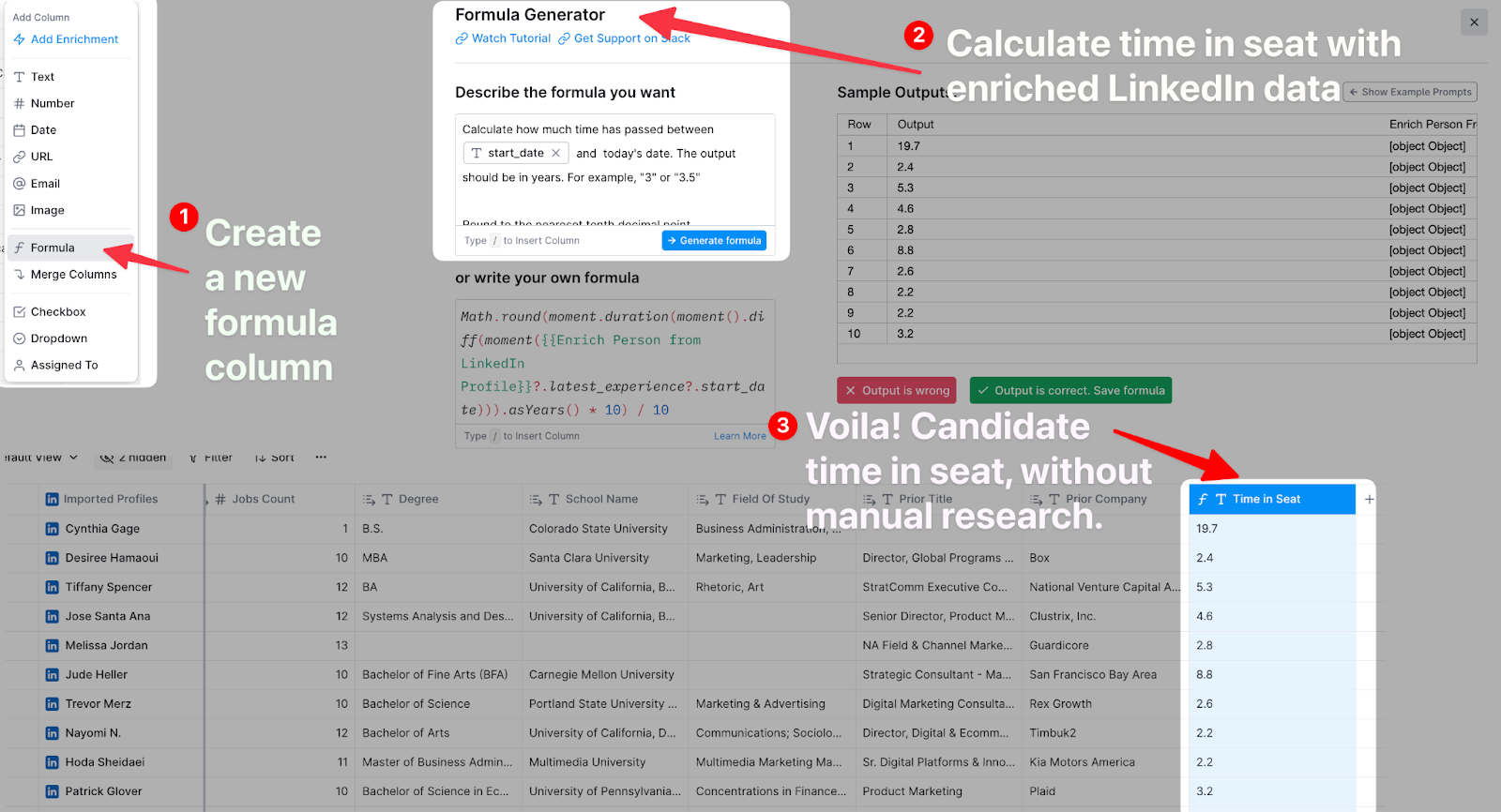
As you can see, this candidate’s time in seat (calculated in years) matches their LinkedIn profile data:
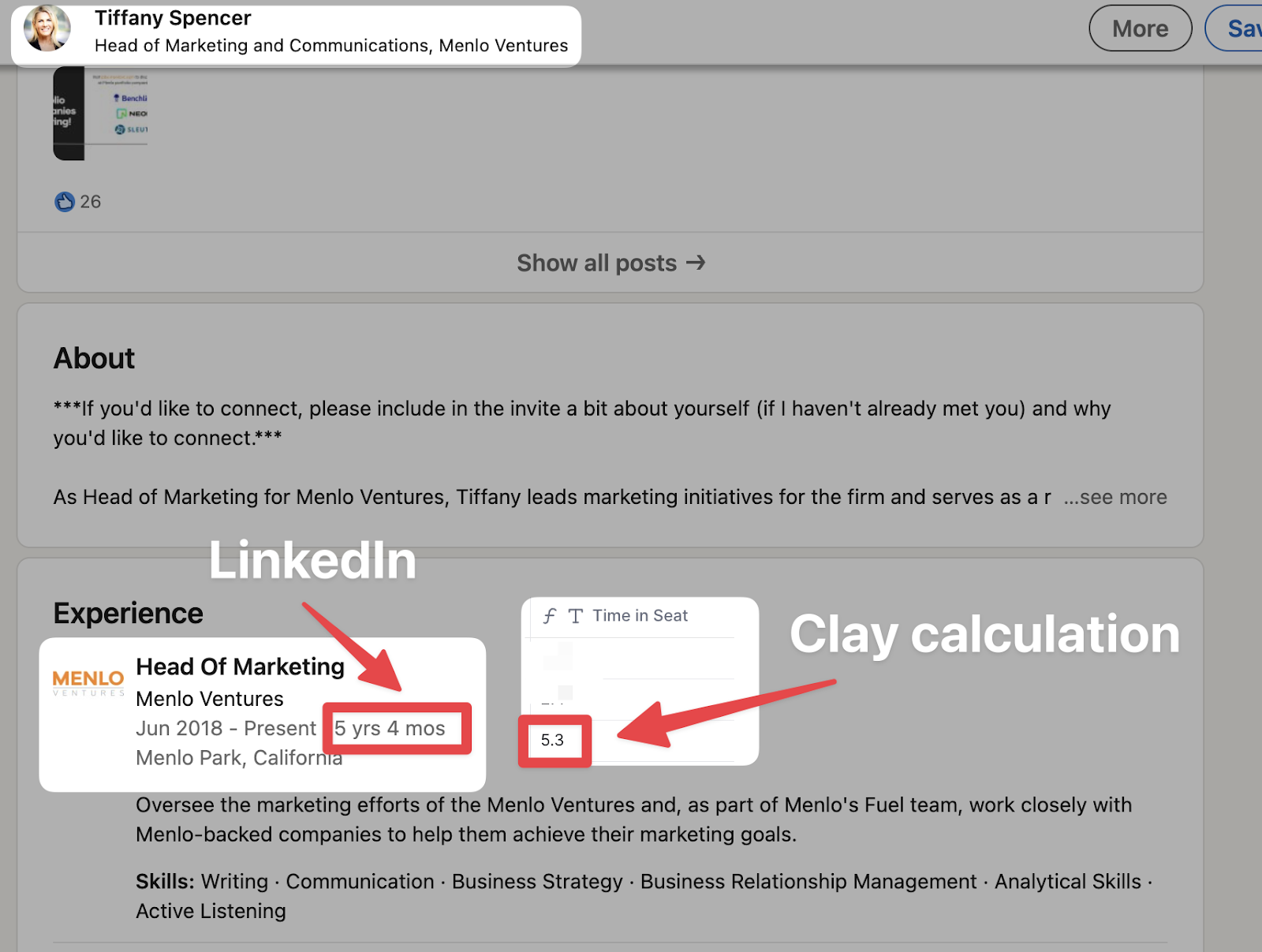
Lastly, we’ll filter to only show candidates in seat for at least two years:
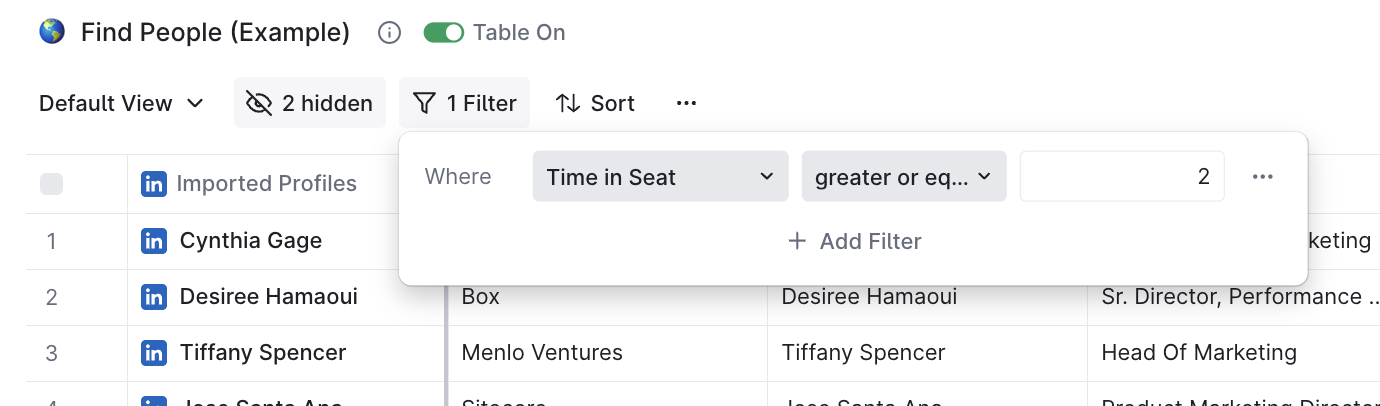
Now, without any manual research, we can automatically determine if a company has at least two years in their current seat.
Step Two: Automate your manual research with AI
Before you email a candidate, you need to know things like:
- Years at current company
- Time since the last role ended
- Current/Past company industry
- Current/past company job description
- School, Degree, Major
- Location
Without AI recruiting tools, you have two bad options to get this information. You can either spend tons of time manually checking each profile. OR you can skip the research since it takes so long, and blast messages to tons of people.
With AI, you can access all the above information without spending time on manual research. Extract the information above - and more - from candidate profiles into a Clay table, and use AI to determine whether a candidate fits your role based on any of those data points. Let’s show you how.
First: most jobs have specific requirements beyond what we’ve already shown above.
Let’s use this VP of Marketing position on Indeed as an example:
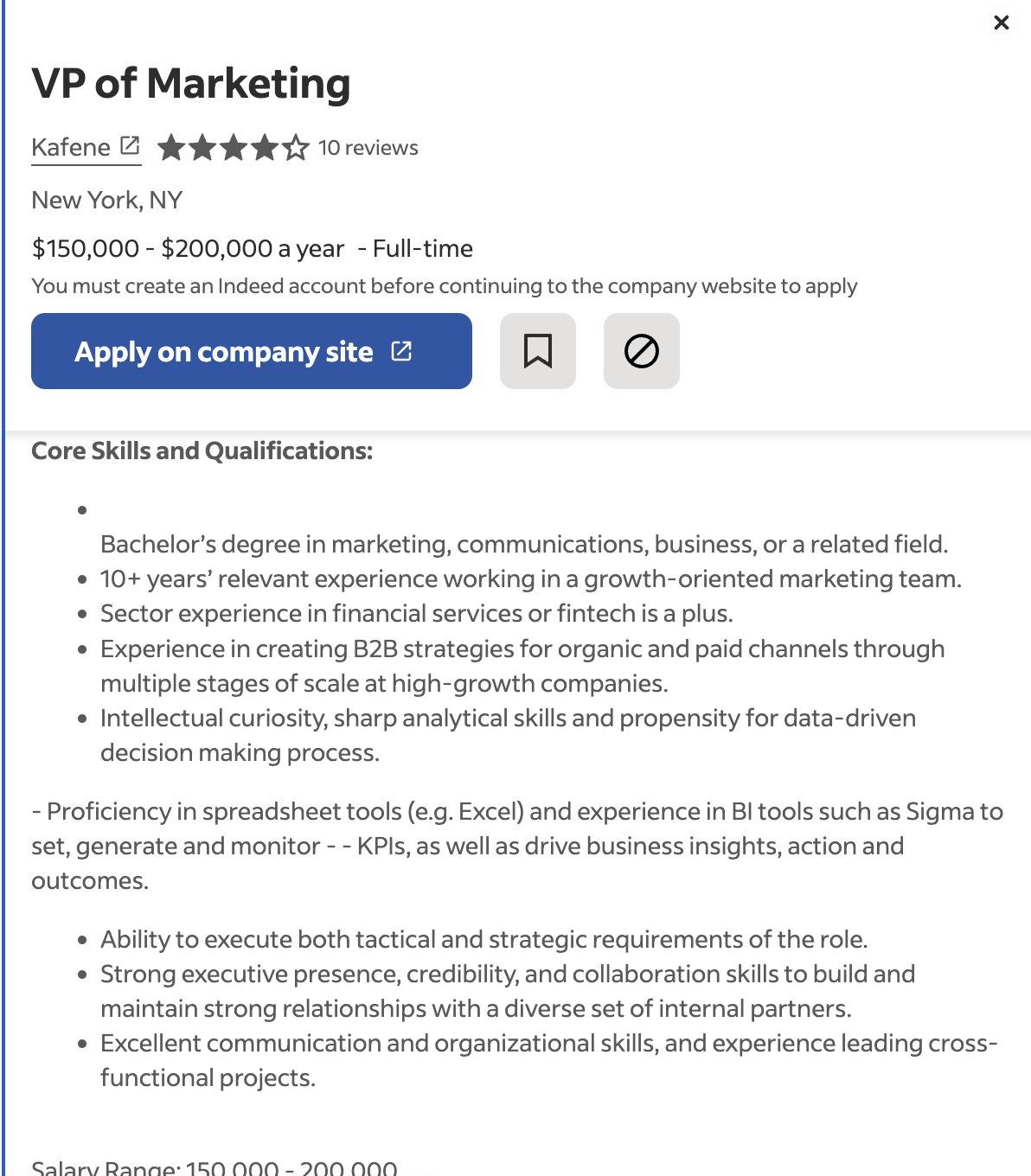
The role requires:
- At last 10 years of marketing experience
- At least 2 years of leadership experience
- At least a bachelor’s degree
The role also lists some “nice to have” experience:
- Worked in the finance industry
- Specific technologies (Excel, Sigma, HubSpot)
Lastly, the candidate ideally has experience relevant to the job’s core responsibilities:
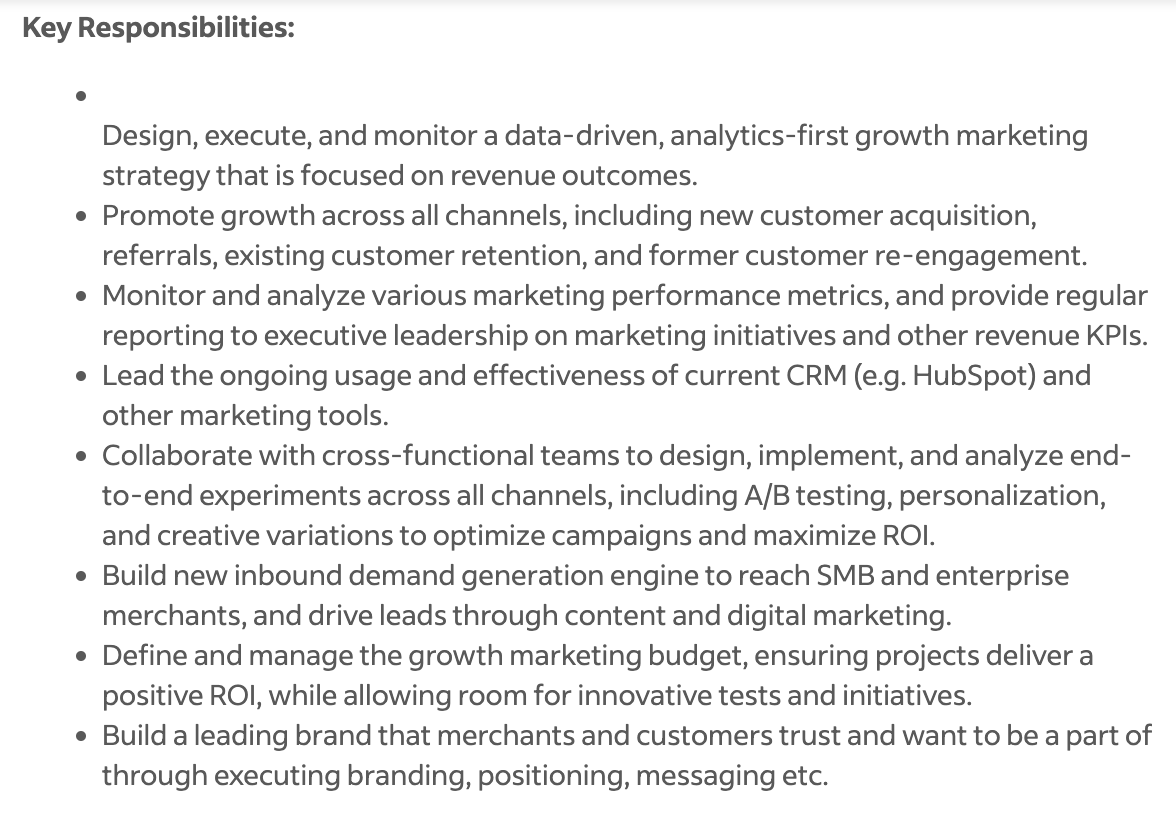
Before ChatGPT hit the market, sourcers had to manually research every candidate’s profile to answer these questions… or send a message to many unqualified candidates.
With Clay and ChatGPT, we can automate this research with a clever prompt.
NOTE: This is for advanced Clay users. If you’re a beginner, you may want to skip this step or reach out in our Slack community for help.
Job Requirements (Advanced)
GPT is only as powerful as its prompt and input data. The more specific you can be with your prompt, the better the output.
In our case, we’ll use GPT to ensure candidates satisfy our job requirements. Later, we can use GPT to see how well a candidate’s experience fits our job responsibilities.
We’re looking for experience in marketing, experience in leadership, experience with specific technology, and at least a bachelor’s degree.
Here’s the prompt I gave ChatGPT:
You are a recruiting sourcer looking at candidates' LinkedIn profiles to understand their past work experience and see if the candidate's background makes them a good fit for the job based on the job requirements (needs) and job "nice to haves" (wants). You will be given a list of job experience mandatory requirements, "nice to have " requirements, and tech stack experience requirements. Your job will be to scrape a LinkedIn profile, and see if the candidate meets the list of requirements. Here are the job experience mandatory requirements:
- Minimum education: bachelor's degree or greater
- Minimum years experience in marketing: 10 years
- Minimum years experience in a leadership role: 2 years
Here are the "nice to have" requirements:
- Background in finance
Here are the technologies the candidate will need to configure:
- HubSpot
- Excel
- Sigma
Candidate's LinkedIn profile:{Enrich Person from LinkedIn Profile}
You will return back your findings in JSON format.
You are now going to be given a list of questions. Each question has the JSON key in () afterwards.
- Does the candidate meet the minimum education requirement? (minimum_education_requirement) - What is the candidate's level of education? (education_level)
- Does the candidate have the minimum marketing experience? (minimum_marketing_experience)
- How many years of marketing experience does the candidate have? (marketing_experience_years) - Does the candidate meet the minimum number of years in a leadership position? (minimum_leadership_experience)
- How many years of leadership experience does the candidate have? (leadership_experience_years)
- Does the candidate have a background in finance? (finance_experience)
- If applicable, describe the candidate's finance background in 10 words or less (finance_experience_description)
- Does the candidate's profile explicitly mention any of the technologies they'll need to configure? If the profile does not explicitly mention any of the technology keywords, this answer is false. (technology_experience)
- If applicable, list the nice-to-have technologies mentioned in the candidate's profile, and describe where you found the technology explicitly mentioned. (technology_experience_names)
Notice the prompt is quite long. I also told GPT to return the answer in JSON format.
In a nutshell, JSON is compact and readable text formats for structuring data. If you don’t know what JSON is, this 90 second video will help.
We’re using it here to ensure a standard response is generated by GPT. Otherwise, the answers would be returned as long sentences, and it’d be impossible to consistently make use of the data.
Here’s what a GPT output looks like in JSON format:
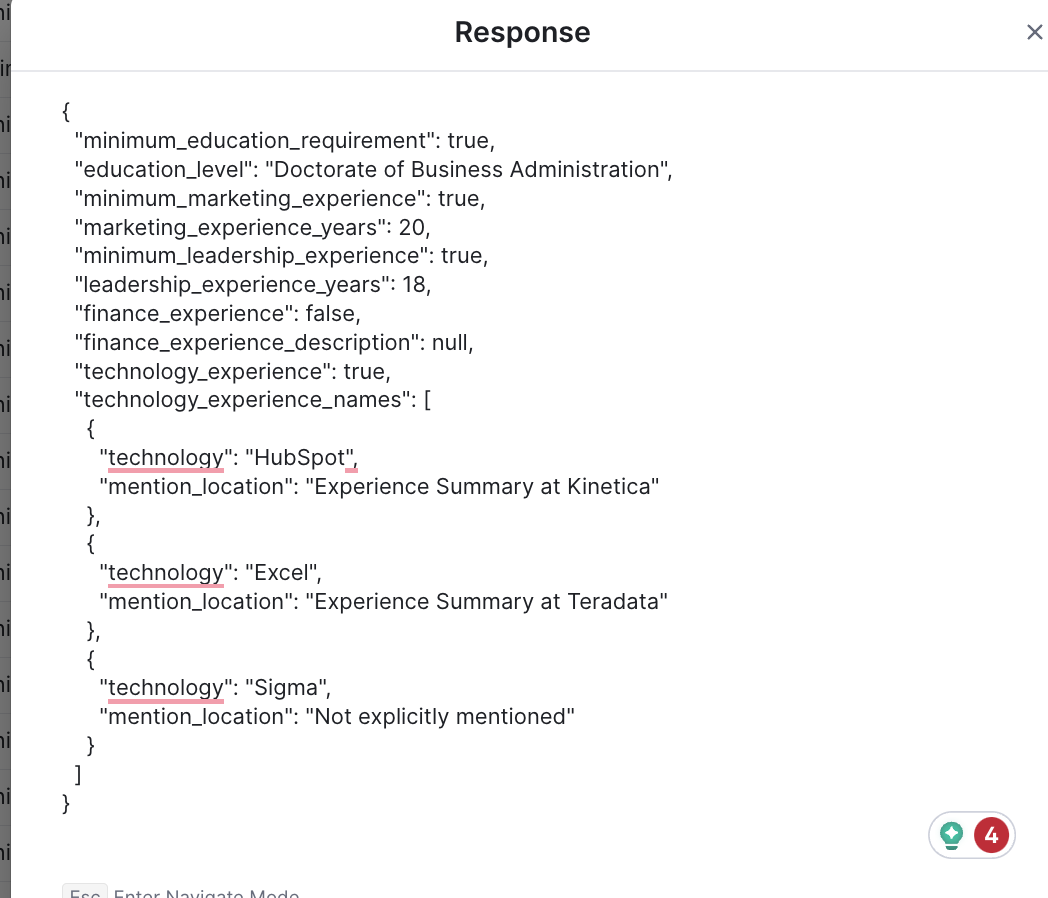
JSON makes it easy to extract data like “education level” or “marketing experience years”.
Here’s an example of candidate information without JSON format:
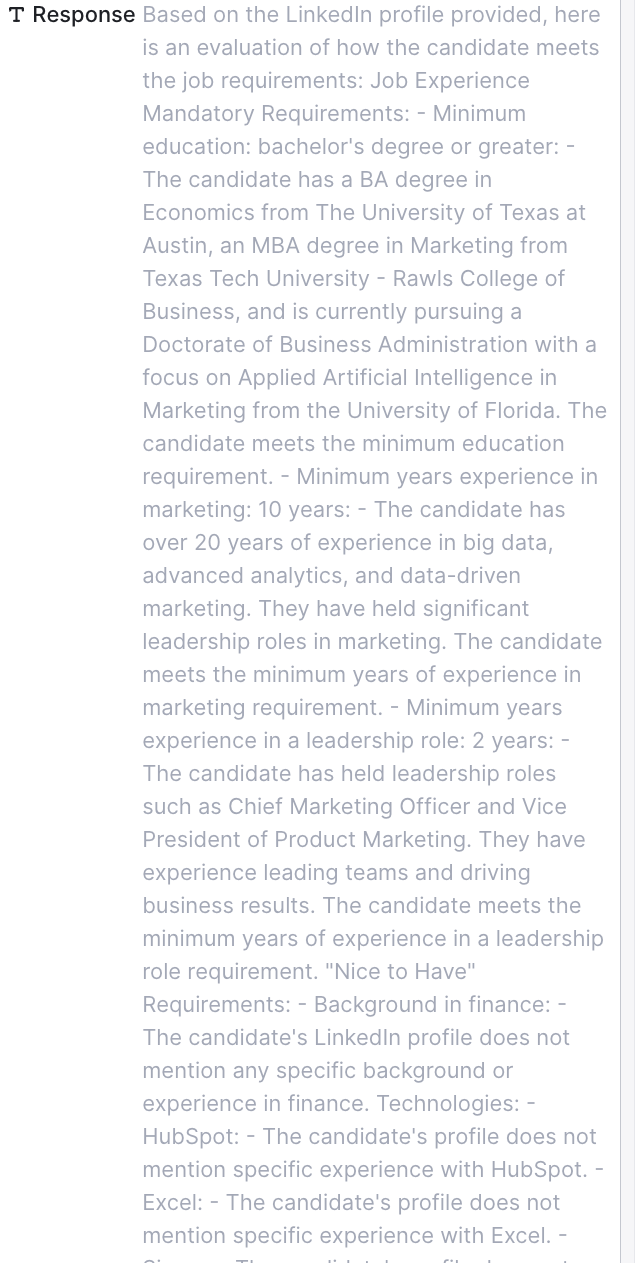
As you can see, it’d be nearly impossible to consistently glean the correct information from a bulky paragraph of text.
Once you understand JSON, it’s pretty easy to extract data and use it effectively for segmentation and personalized outreach messages.
Next, we will pull out the data from the GPT scrape to filter out candidates that don’t meet our requirements and use the scraped information in a personalized outreach message.
Extracting Data from the GPT Scrape
We can use more AI formulas to extract the information we want from GPT’s JSON output.
In this case, we want:
- Years of marketing experience
- Years of leadership experience
- Minimum education requirement met? (true false)
- Education level
- Finance experience
Let’s start with years of marketing experience.
JSON uses “keys” to store values.
For example, “marketing_experience_years” is the key, and “20” is the value. In layman’s terms, GPT determined this candidate has 20 years of marketing experience.
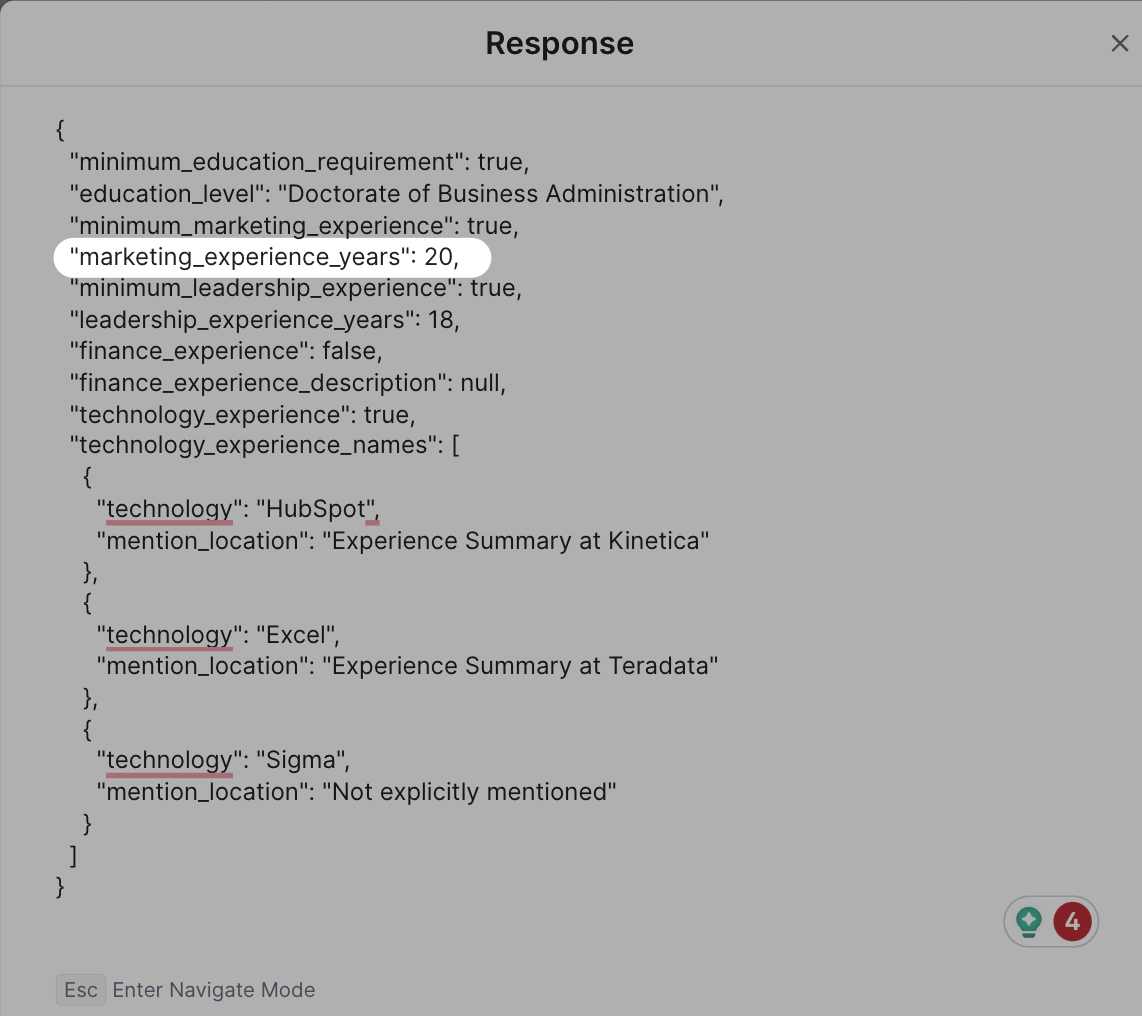
We’ll use an AI formula to extract the value (20) from the key (marketing_experience_years). This will give us a column in our Clay table populated with each candidate’s years of marketing experience.
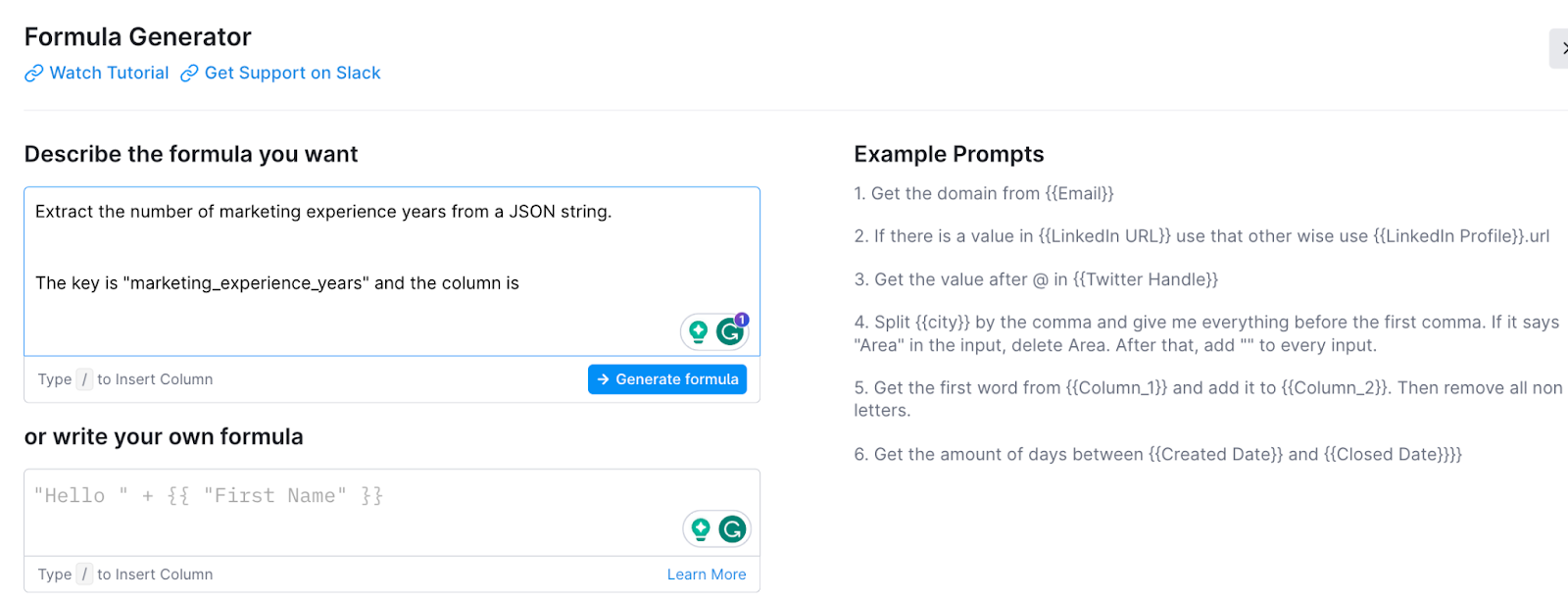
First, use the prompt above to tell the AI formula generator we’re extracting a value from a JSON string. Next, we’ll give our AI prompt the key (the placeholder for our value, in this case, marketing_experience_years).
Lastly, we need to tell the formula where to look for our key. It’s stored in the GPT response column.
We can use any data from our Clay table in an AI formula. Type “/” and then select the data you want.
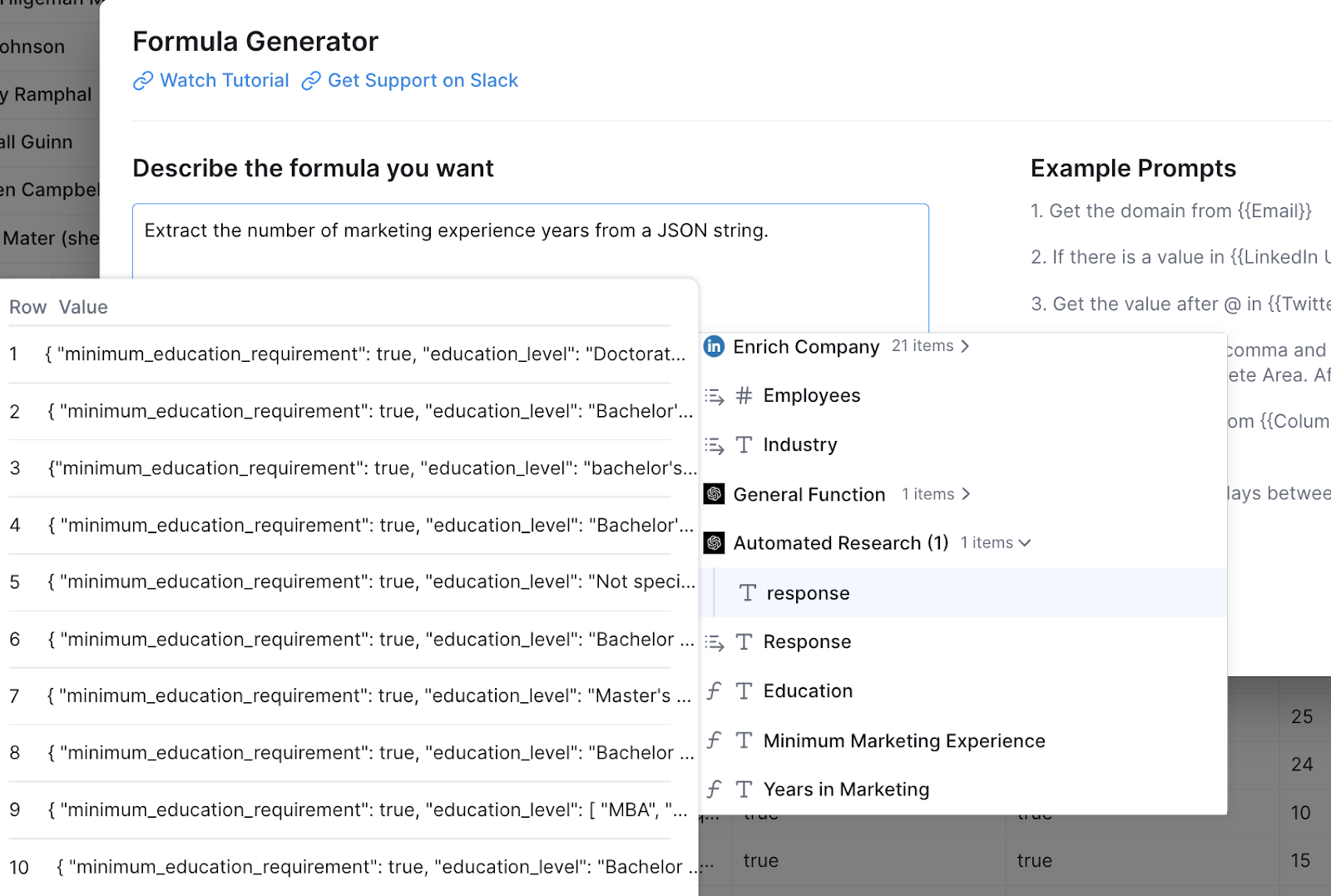
Click “Generate formula” to see a list of sample outputs, each containing your candidate’s marketing experience.
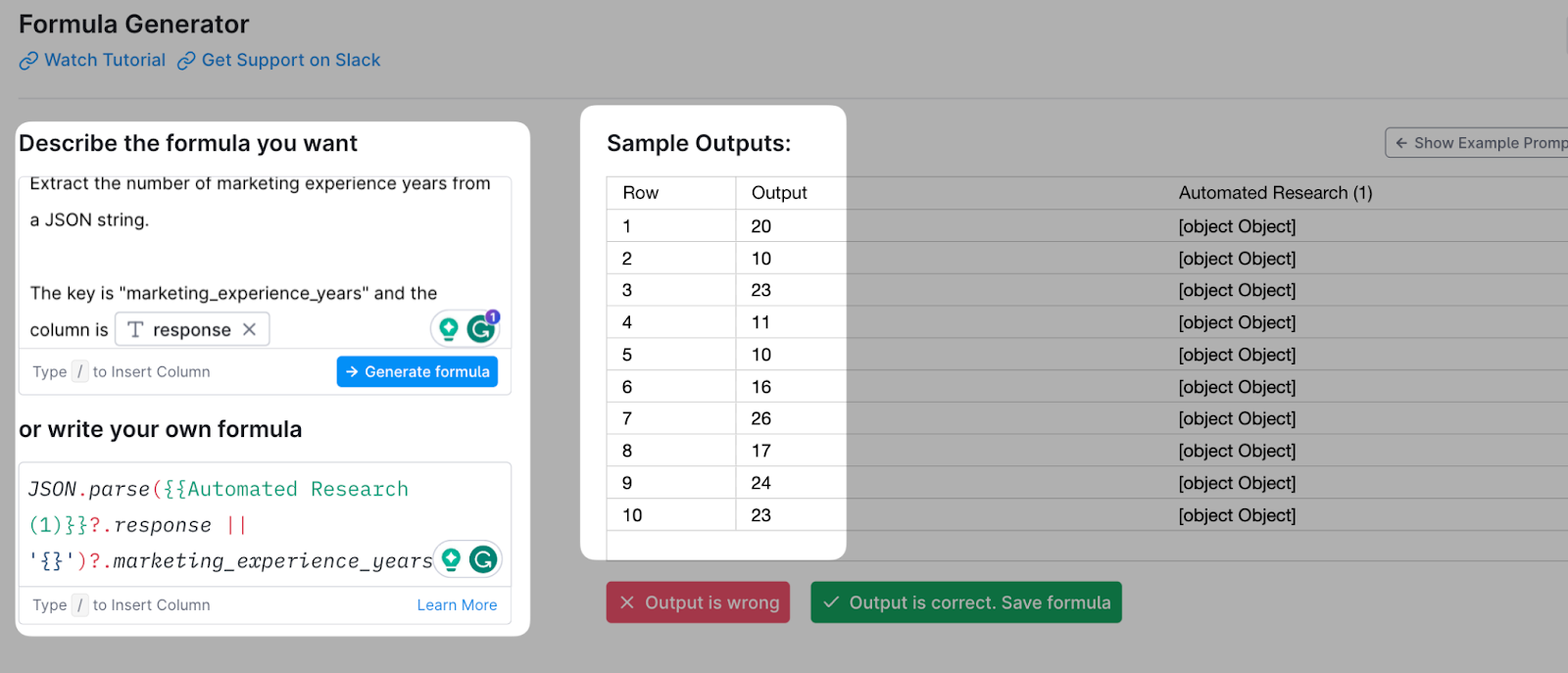
Click “Output is correct,” and you’ll have a table column of each candidate’s marketing experience, ready to filter and use in personalized outbound campaigns.
We repeated this step for years of leadership experience and education level. You can repeat the step above for any data you want from the GPT scrape.
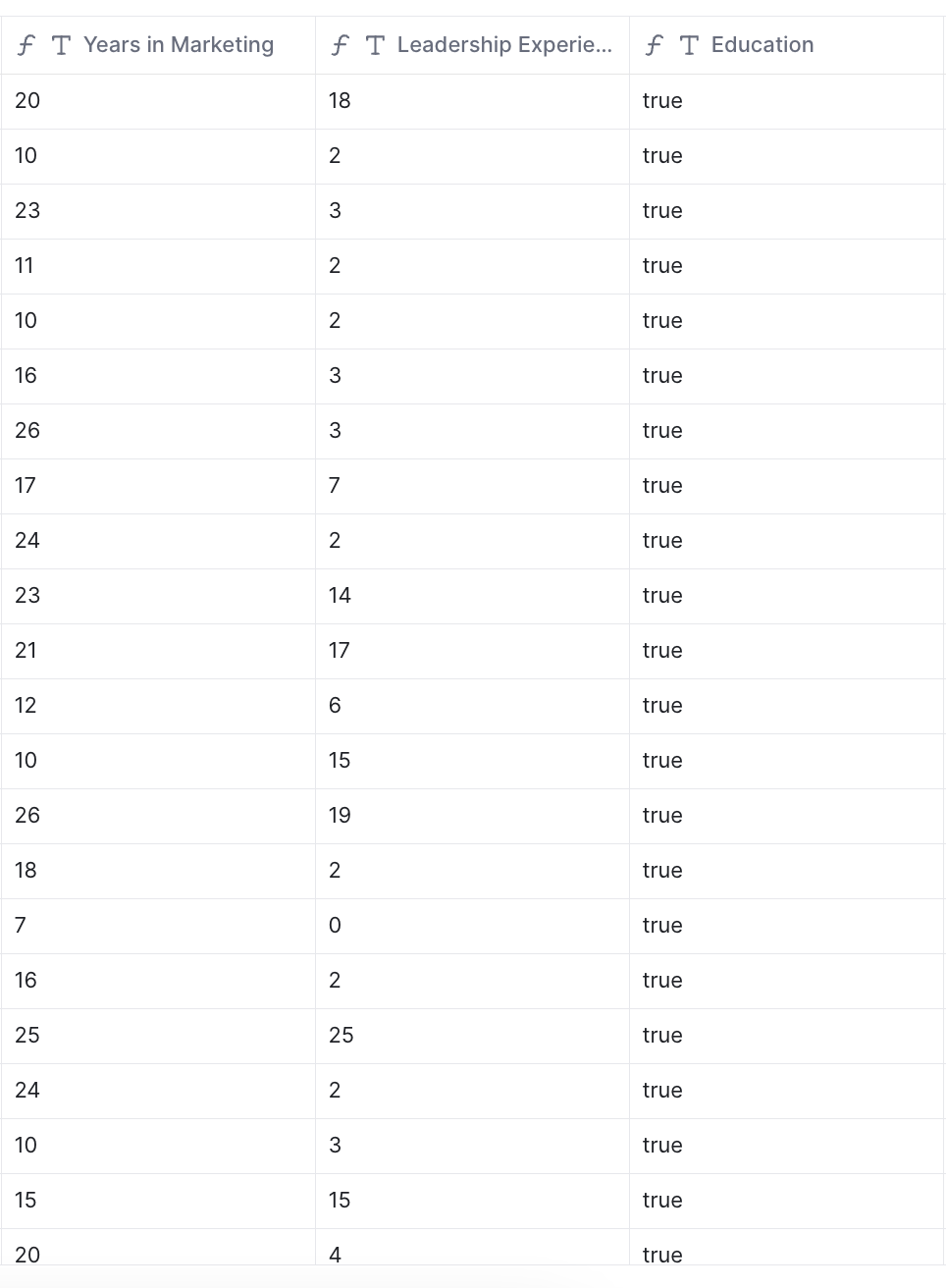
Lastly, we’ll filter candidates that meet our job requirements:

PS: By default, the formula column is a “Text” data type. If you want to filter a numerical value (like the number of years in marketing), change the data type to “Number.”
Click on the column, then click Edit Column, then change the data type to Number and save.
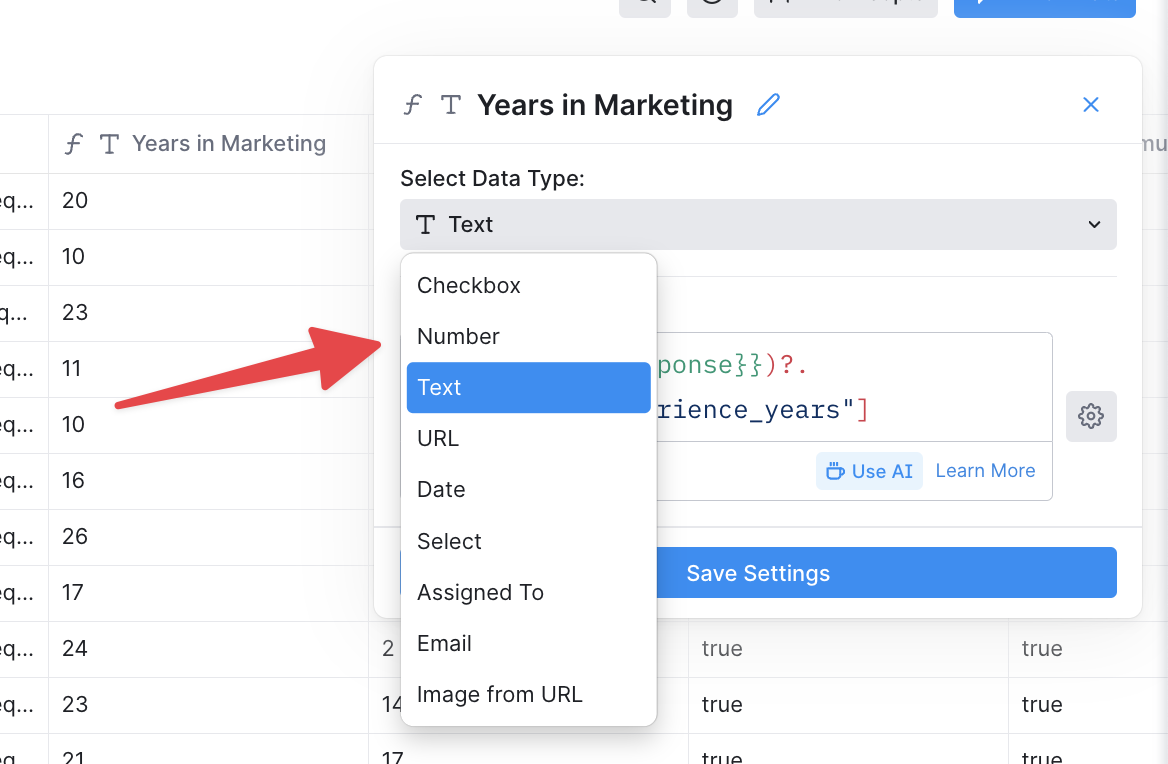
Now we’re left with a list of qualified candidates (marketing leaders in the San Francisco area with at least 10 years in marketing, 2 years of leadership experience, and at least a bachelor’s degree):
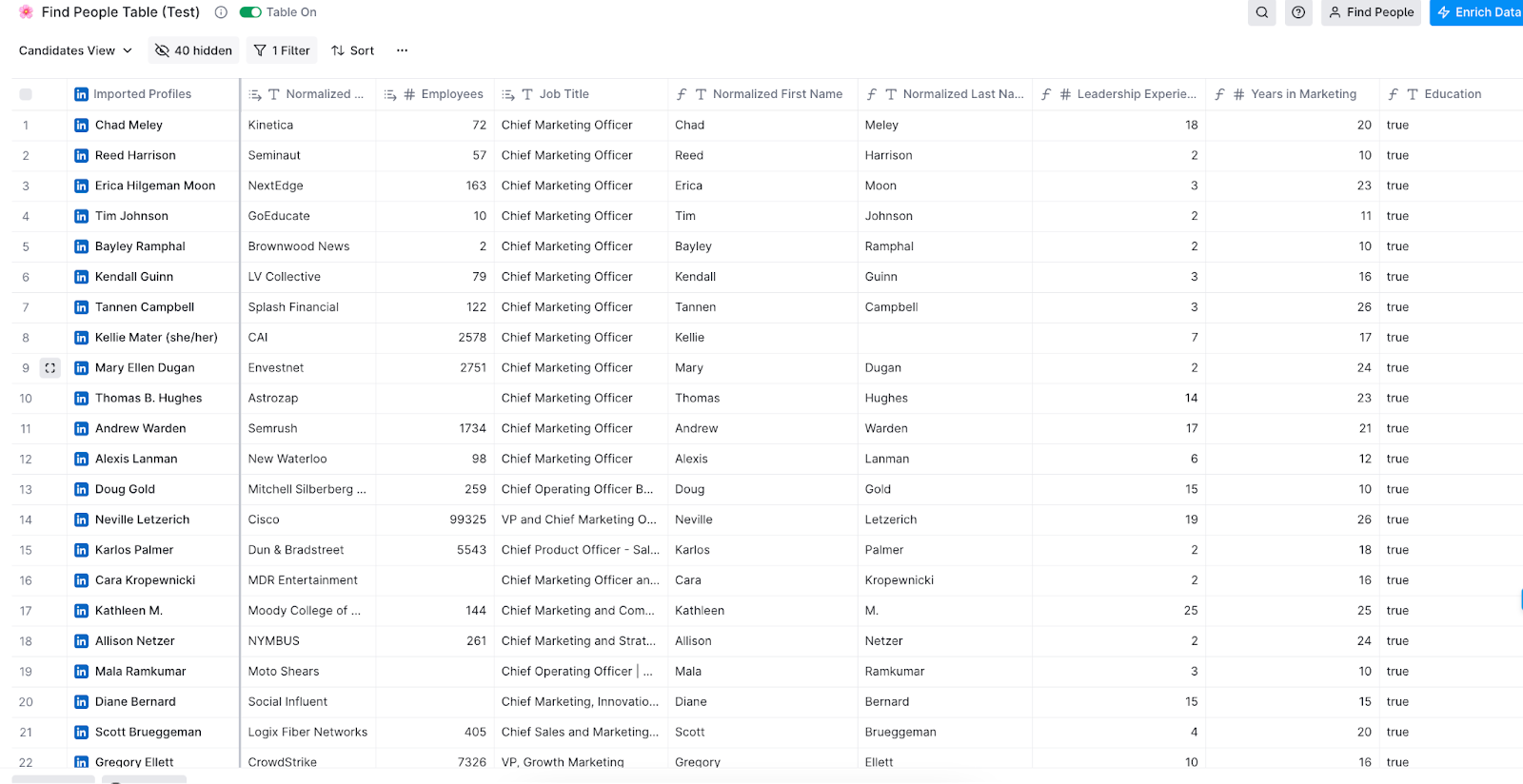
Write Qualified Candidates to New Table for Engagement
It’s time to find candidate emails. First, we’ll push our qualified candidates to a new table and find emails there, so we can separate our “Automated Research” workflow from our “Find Emails, Personalize, and Engage” workflow.
Duplicate the Clay table, and add the columns you want to populate in the new table.
We’ll use the following columns in our example:

- Company Name
- Job Title
- First Name
- Last Name
- Candidate Location
- LinkedIn Profile URL
- Company Website URL
- Company LinkedIn URL
- # Years at Current Company
- # Years of Marketing Experience
- # Years of Leadership Experience
Next, we’ll push our qualified candidates into a new table with the “Write to Other Table” integration.
Choose the new table, and map columns from your first table to the second table:
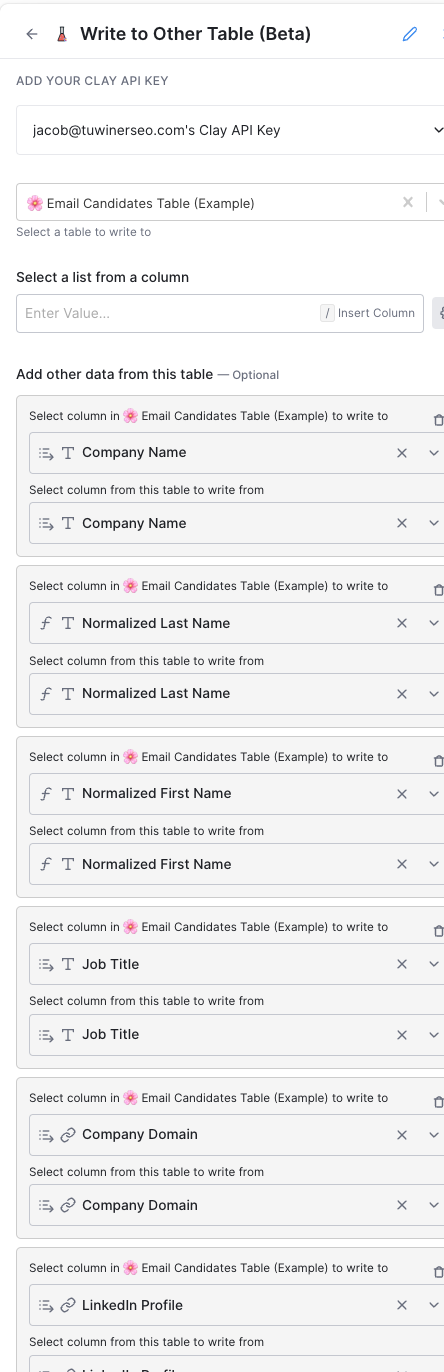
Run your rows, and you’ll see your data in the new table:
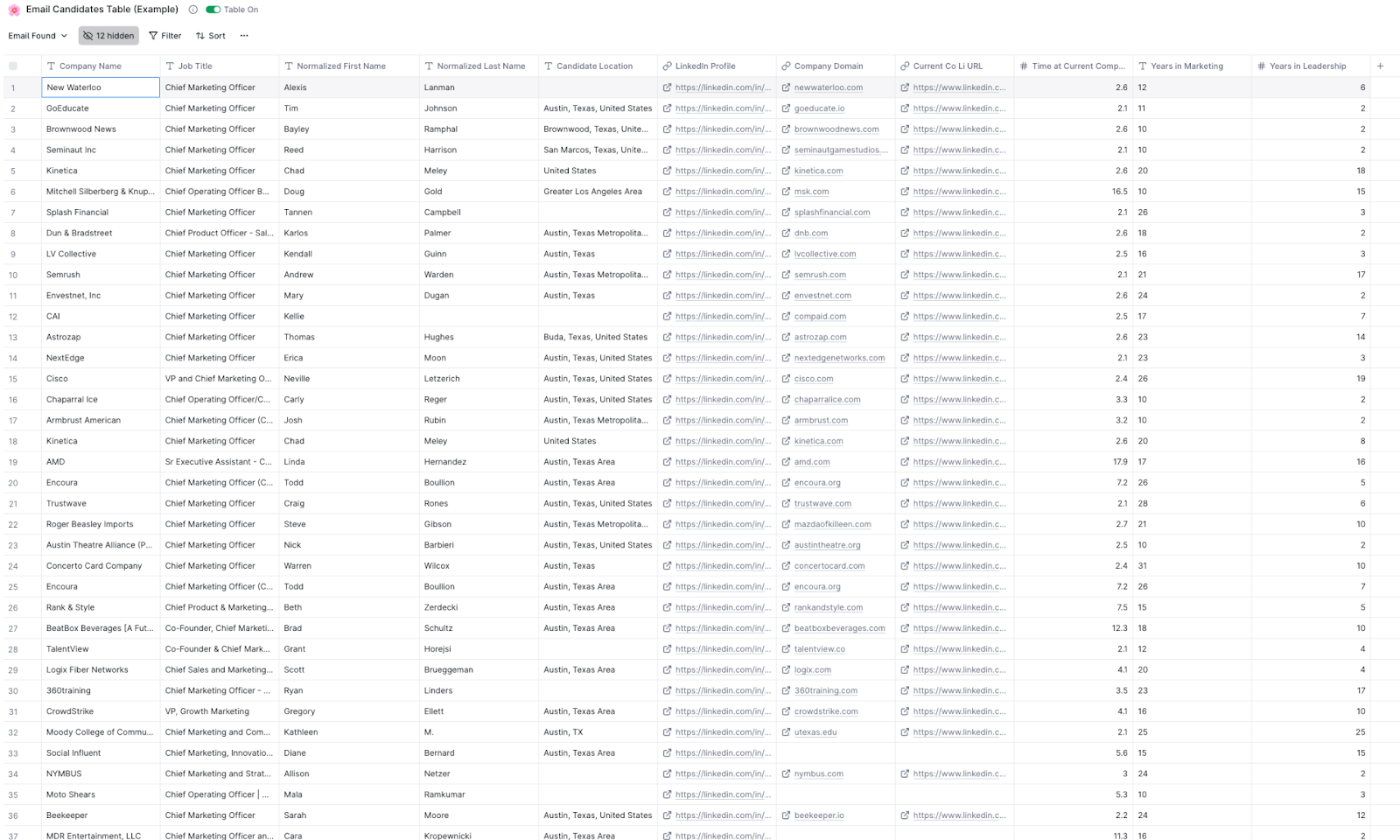
Find Candidates’ Personal Emails
We want to find personal emails, so candidates won’t worry about responding to a job offer from their employer’s email account.
We’ll use the “Find Personal Email” waterfall, which uses four data providers to find a personal email address. If one provider doesn’t have an email, Clay will automatically check the next provider until you find a match.
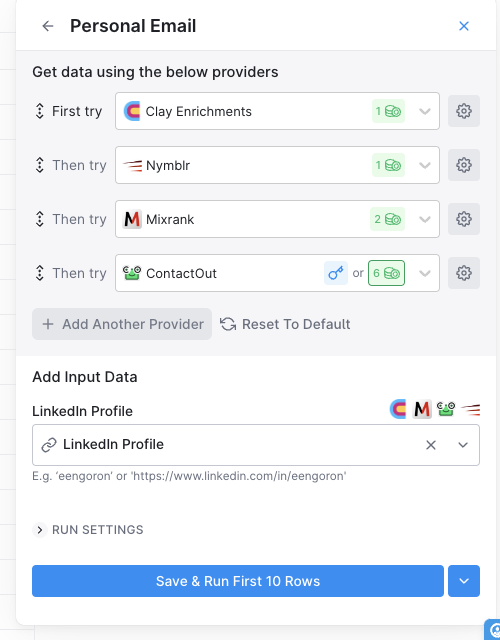
That is pretty good coverage! We found 33/37 candidate emails (89% coverage). You can also verify emails as well with NeverBounce or another provider.
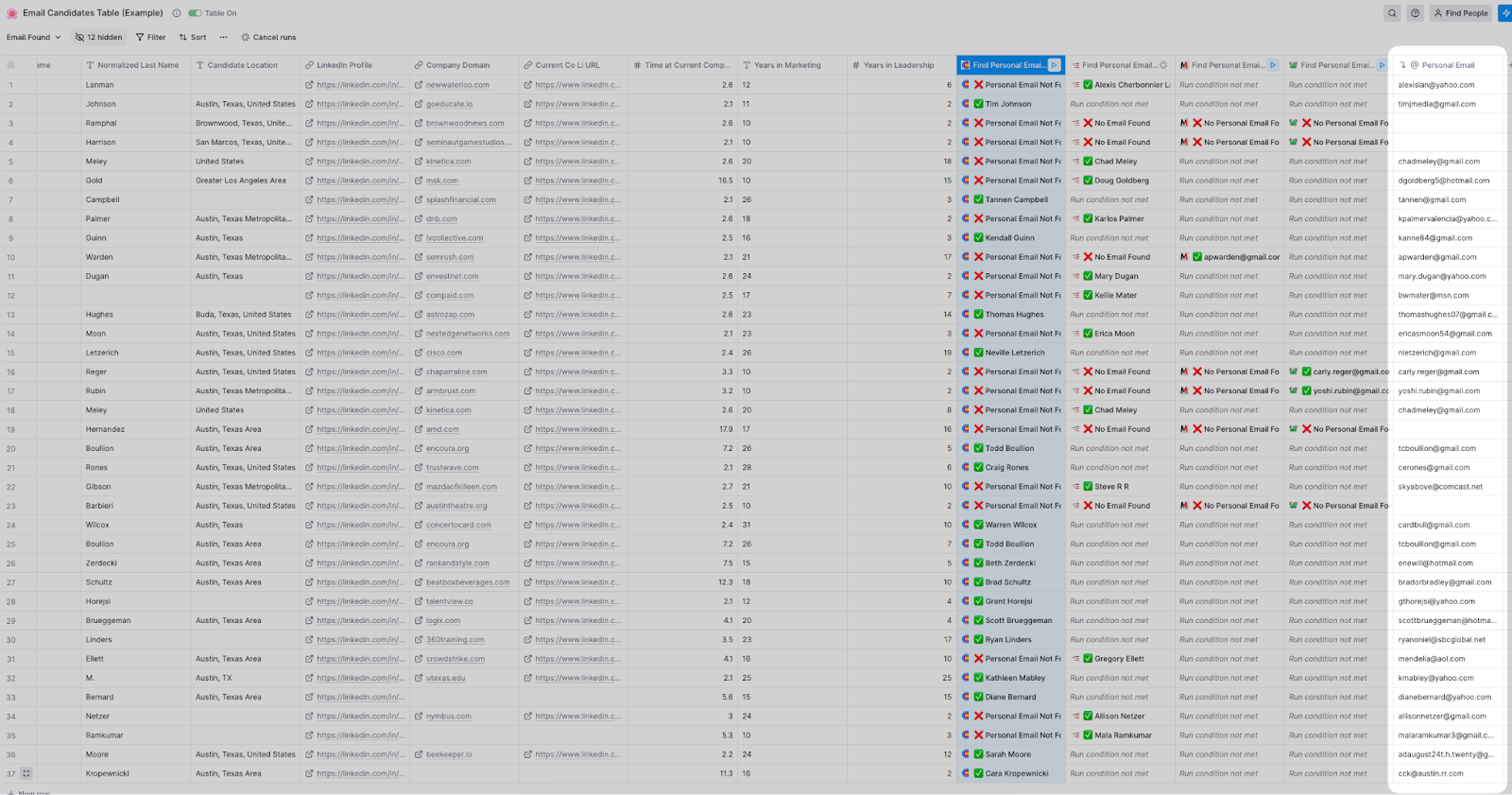
Let’s create a new table view showing only qualified candidates with verified emails.
Duplicate the view:
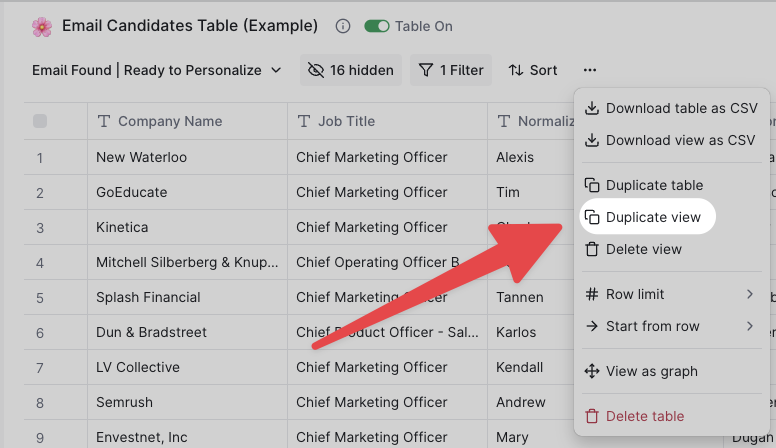
Then, filter out any rows without a “personal email” value:

Now your table shows all qualified candidates with verified personal email addresses:
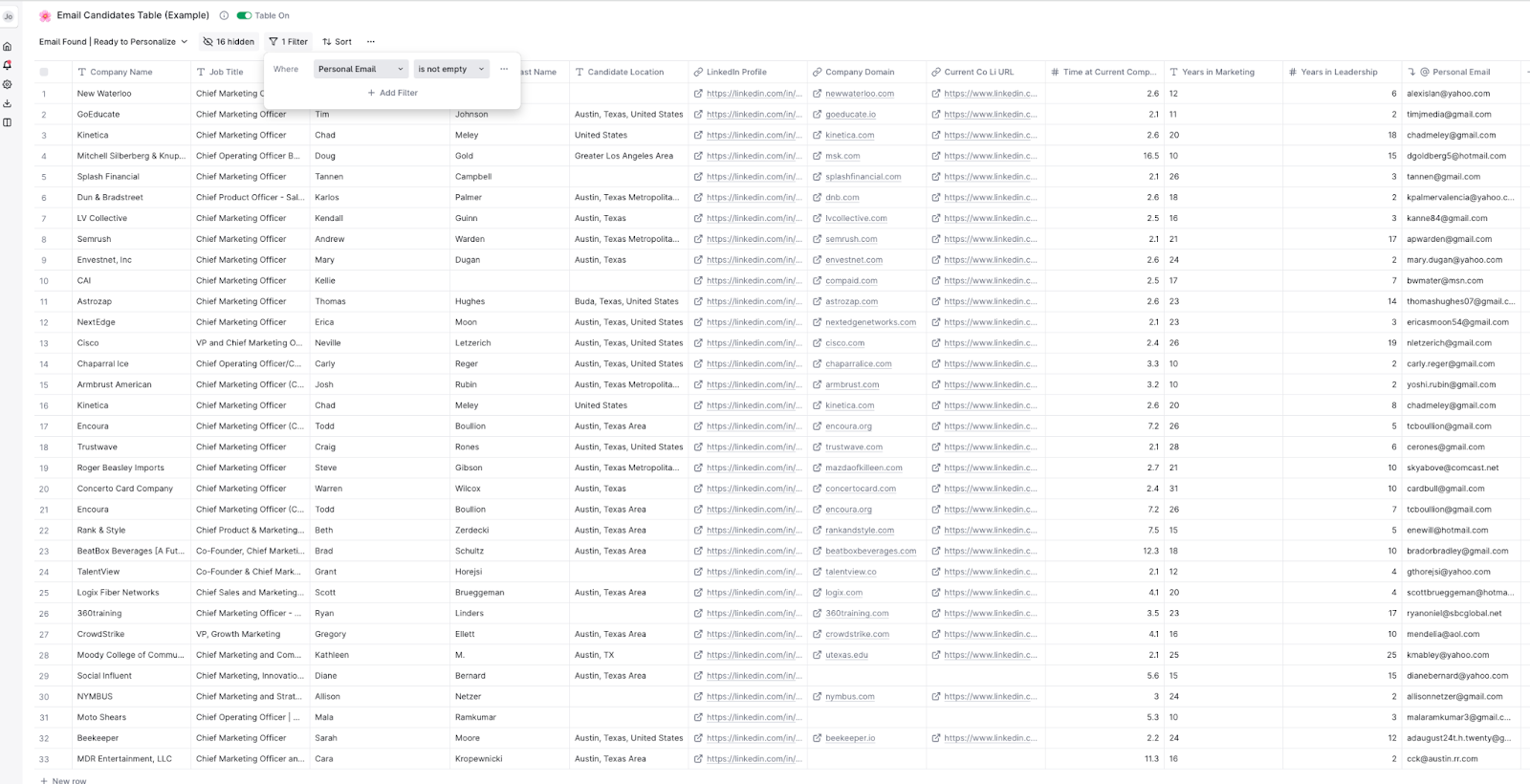
Pro Tip: Use AI to Normalize Company Name
Scraping LinkedIn and other data sources often returns company names with “LLC” or “Inc” or weird capitalization.
Sending an email with a raw company name that includes “LLC” is a dead giveaway that you didn’t write the email by hand. These small mistakes make a huge impact on your response rates because anything that looks like an automated email will often be ignored by a candidate.
Clay can “normalize company names” to remove any weird text from the data. Use the “Normalize Company Name” integration to turn raw data into “email ready” data.
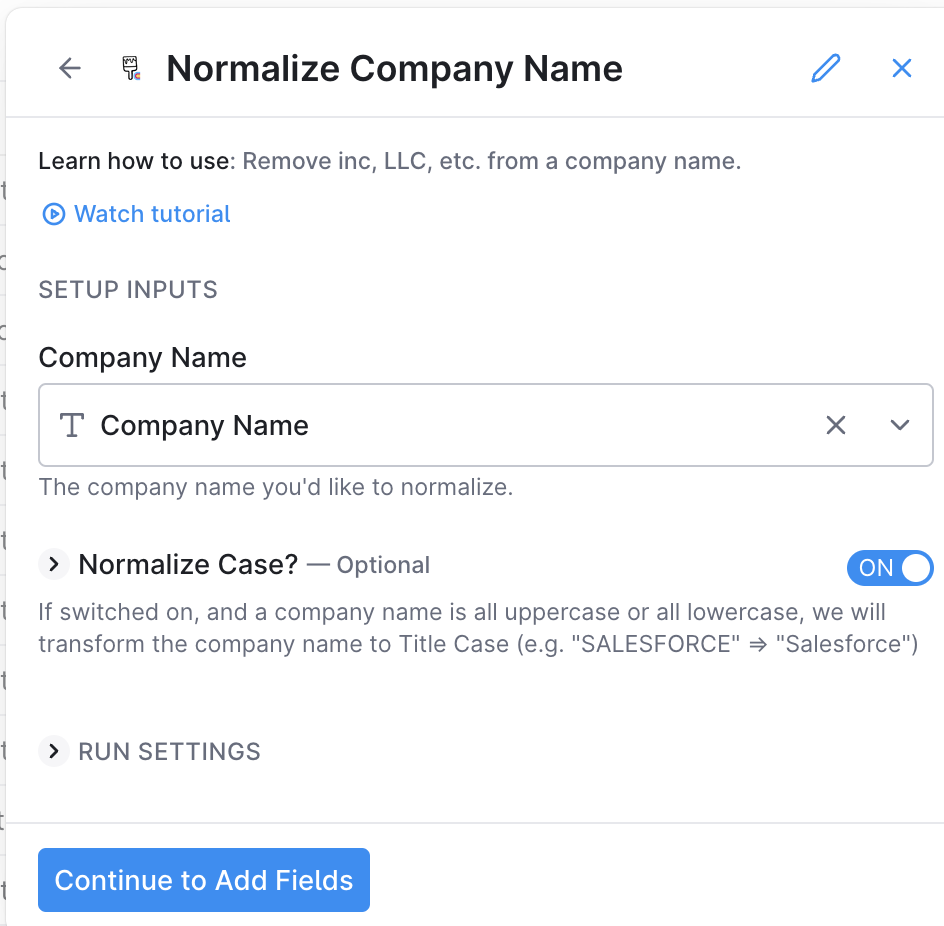
Here’s an example output:

Pro Tip: AI can help Remove Emojis from First Name
Relying on the candidate’s first name from their LinkedIn profile is a bad idea for two reasons:
- Candidates often add emojis to their first name
- Candidates often use their “full” first name, but usually “go by” a nickname
For example, let’s look at Felipe, Instantly’s partnership manager:
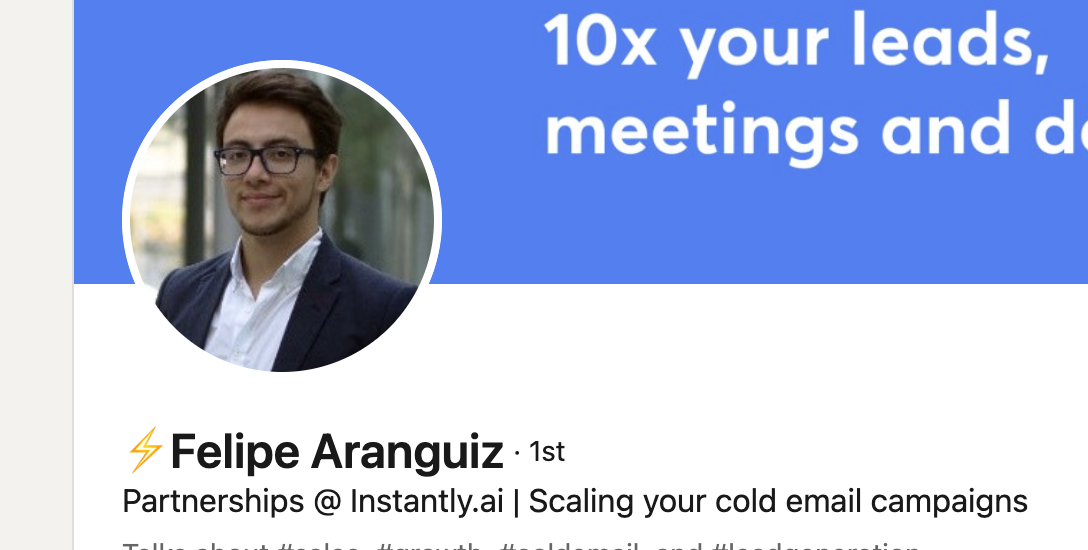
Felipe has a ⚡in his first name.
If we scraped his first name without formatting the data, we’d say “Hi ⚡️Felipe!”
He’d instantly (haha, get it?) know we sequenced him.
Instead, we’d want to format the first name to “Felipe”.
Let’s remove the emoji from the first name. Create a new column and use an AI formula to populate it with the candidate’s first name:
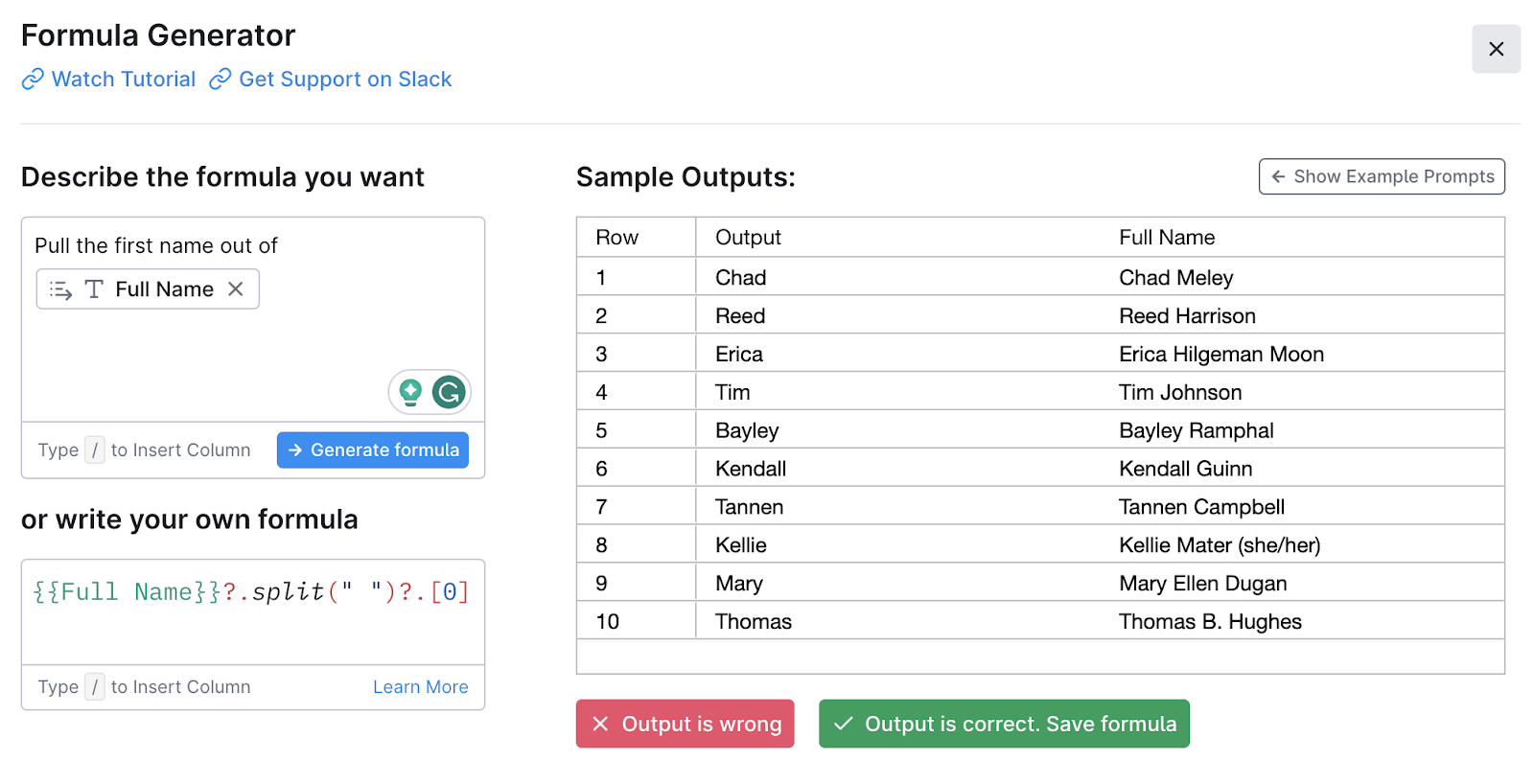
Repeat this step for the last name.
Then, pass the values into the “Normalize first and last names” integration:

As you can see, we removed the emoji from Felipe’s first name (and any other weird characters):

Pro Tip: Use AI to find preferred first names
Here’s another example:
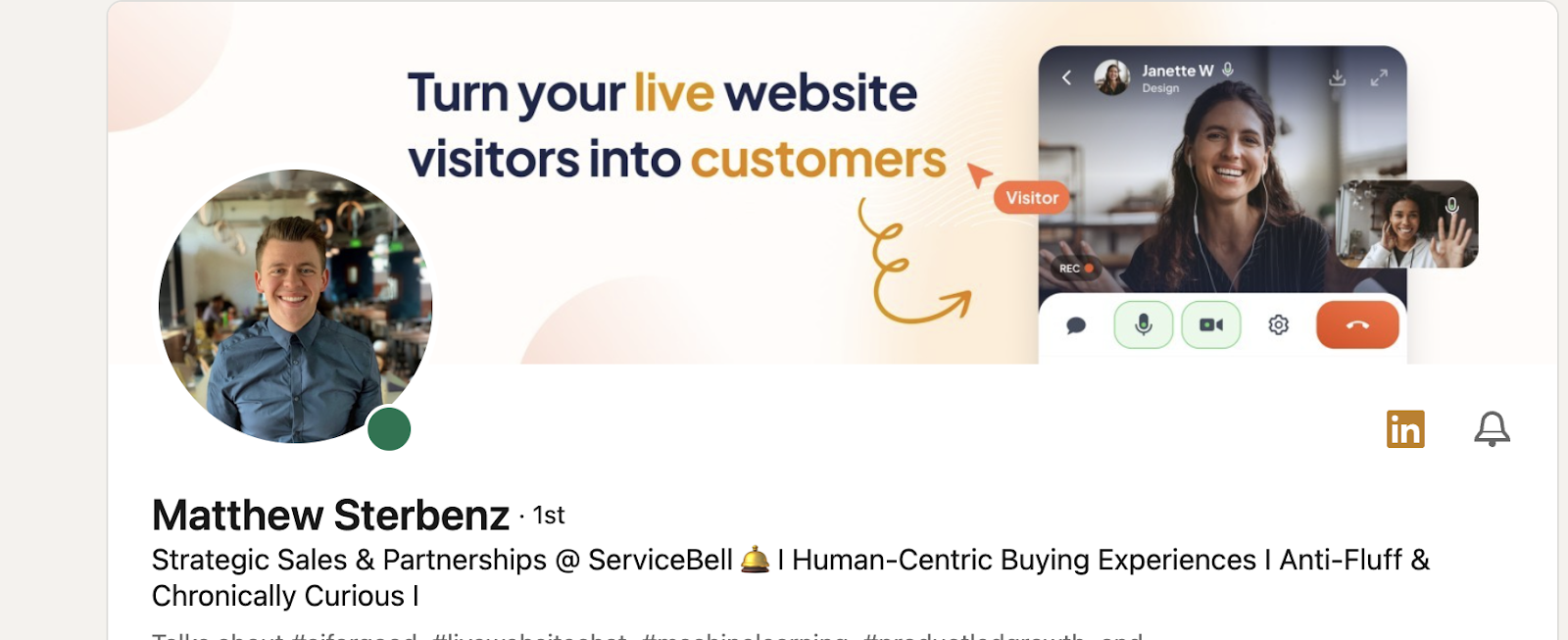
Matthew is a sales rep at ServiceBell.
His LinkedIn first name says “Matthew”, but if we look at his LinkedIn profile URL, it says “Matt”:

His email is “matt@servicebell.com”, not “matthew@servicebell.com”.
Lastly, we can see his peers call him “Matt” in his recommendations:
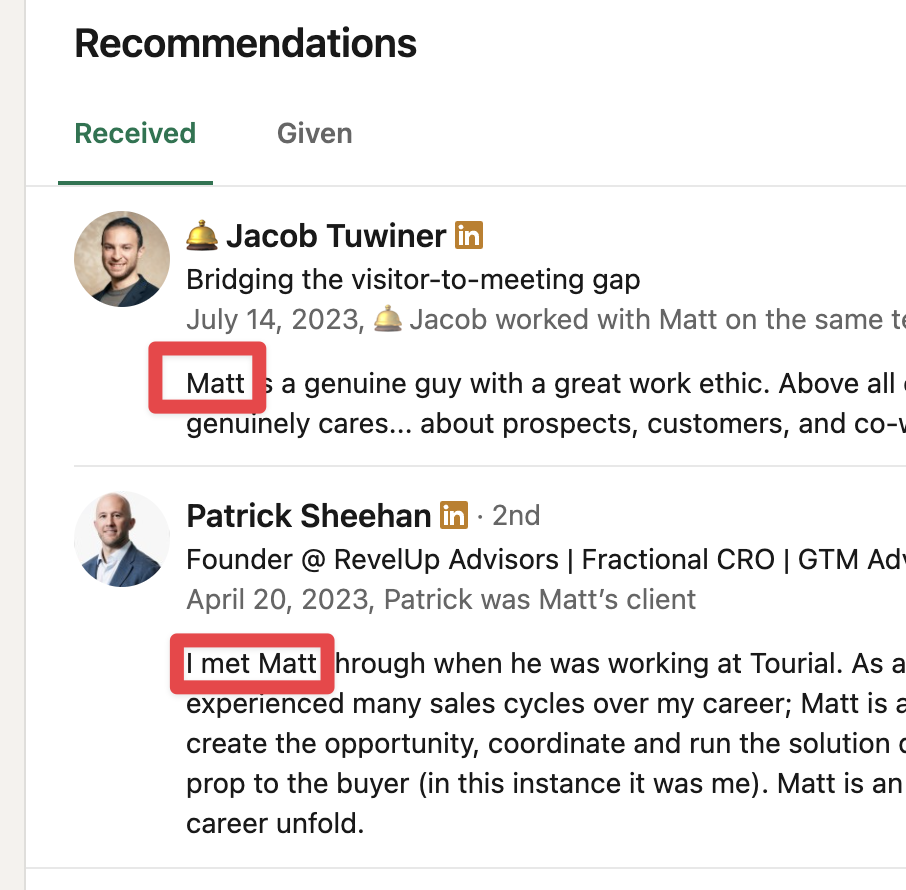
If we email him and say “Hi Matthew”, he’ll instantly know we didn’t write it ourselves (because we would have said “Hi Matt” instead.)
We can use Clay + ChatGPT to check the candidate’s LinkedIn URL and email to determine the candidate’s proper first name.
For example, this candidate calls himself “Gregory” in his LinkedIn first name, but his LinkedIn URL says otherwise.

We’ll use ChatGPT to check the candidate’s First Name, email, and LinkedIn URL, to determine the candidate’s proper first name:

Step Three: Use AI to personalize your recruiting emails
Now it’s time to personalize! We have all the raw data we need to get creative with GPT prompts.
Note: we’re personalizing after finding a verified email, so we don’t waste GPT credits on candidates without a verifiable email.
You could use GPT to write your entire cold email, but we will use GPT to insert custom variables in a pre-written email script.
Giving AI some guardrails help ensure consistency.
Here are some data points we’ll use for personalization:
- Candidate’s “general function”
- Years of experience in marketing and leadership
- Location
Candidate’s Job Function
Remember, we don’t want the candidate to feel like our email was sent to 100 other people.
Pasting the candidate’s job title into a cold email template is usually a dead giveaway that we dropped into a sequence.
Instead, we’ll use GPT to “massage” the data into a human, casual form so it comes across like we wrote it by hand.
For example, here’s a list of “general functions” using candidate job titles as the input:
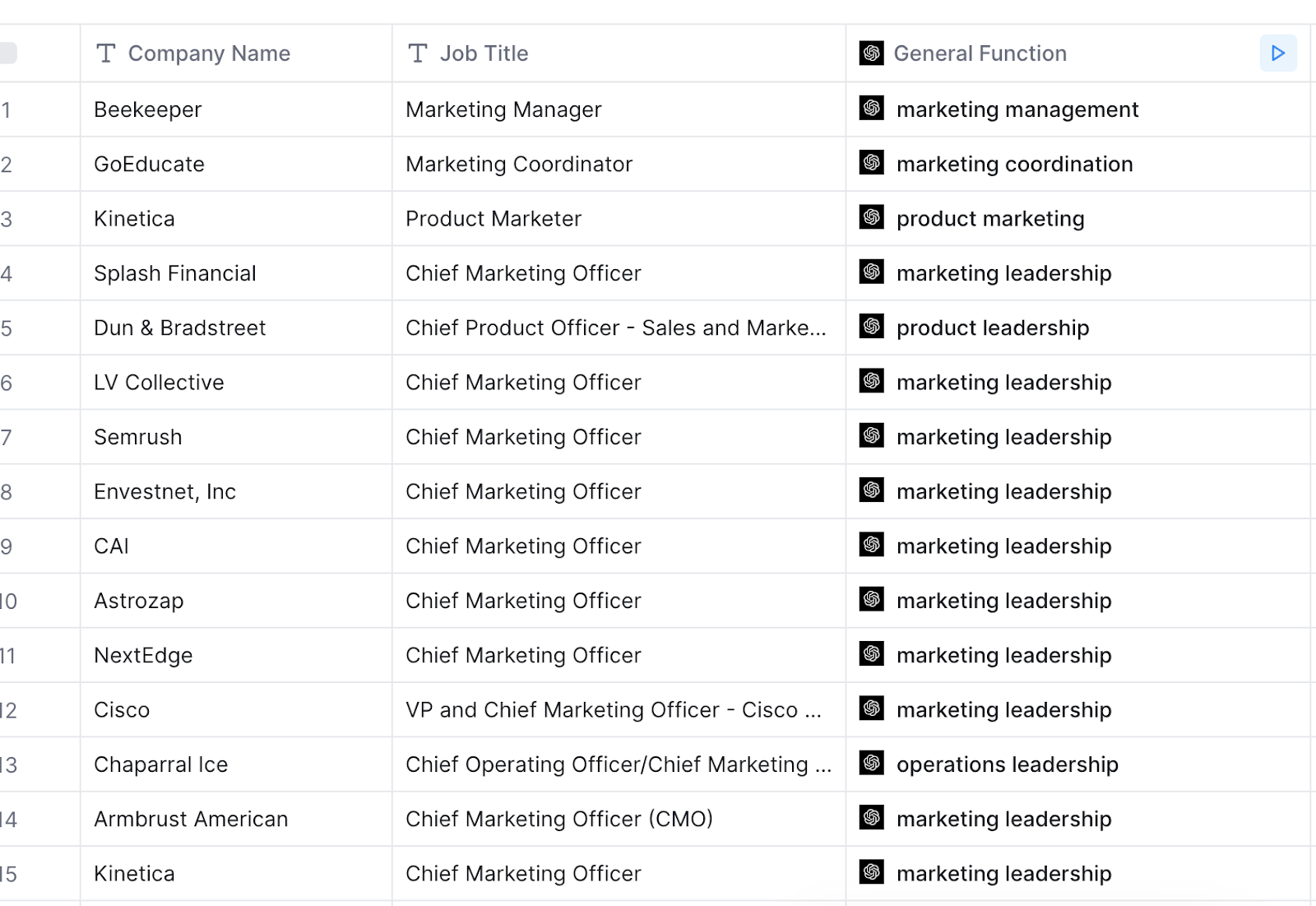
The general functions above would fit nicely in the following template:
“Hi {candidate name}, looks like you’ve been {general function} at {company name} for {years at company} years now.”
Populated with data from Clay, your opener would look like this:
“Hey Jacob, looks like you’ve been in marketing leadership at BeeKeeper for 2.5 years now.”
Here’s the GPT prompt we used:
Your job is to turn a job candidate's current job title into the job candidate's "general function". This is the candidate's job title: {Job Title}. A "general function" is a mix of their persona, and their senioriy level. Persona could be marketing, sales, customer success, operations, product, engineering, etc. Seniority could be leadership, management, or an individual contributor. For example, a "Chief Marketing Officer" job title's general function would be "marketing leadership". A "Marketing Manager" job title's general function would be "marketing management". A "Product Marketer" job title's general function would be "product marketing". The response will fit in the following script: "Hi {candidate first name}, looks like you've been in {general function} at {company} for {number of years} years now." Here's an example for a Chief Marketing Officer job title: "Hey John, looks like you've been in marketing leadership at Apple for 3.3 years now." Here's an example for a marketing manager job title: "Hey John, looks like you've been in marketing management at Apple for 3.3 years now." Here's an example for a product marketer (individual contributor) job title: "Hey John, looks like you've been in product marketing at Apple for 3.3 years now." If the candidate has multiple job functions in their title, determine the candidate's main job function, and only choose the main function. The main function is usually first in the title. For example, if the job title is "Chief Product Officer - Sales and Marketing Solutions", the general function is "product leadership". Keep the response under 5 words max. The response will usually be 2-3 words. The response should make sense in the script above. Your response is NOT the full sentence. It's only the text that will fit in {general function}. Your response should always make sense in the context of the script, but it is not the script itself. Never return your answer in quotations. Never return the title itself.
Normalize Location Name
You may want to confirm a candidate’s current location, especially if the job you’re filling requires in-person talent.
Mike Cohen’s script also had a cheeky “Still in {area}?” question in the opening line to add some personalization.
The raw data from LinkedIn is not ready for a cold email:
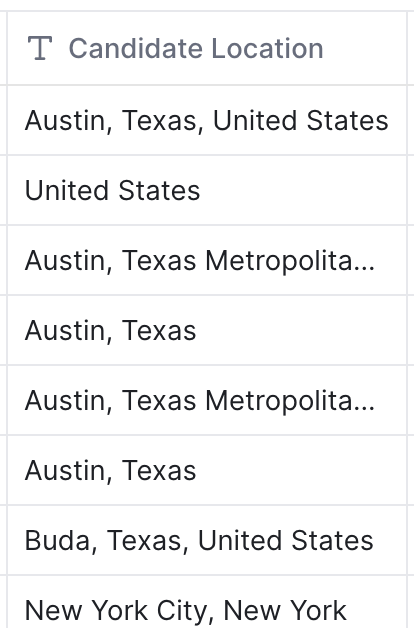
We’ll use a GPT prompt to normalize the location names, so we can use them in a personalized cold email (without looking like a robot).
Check out the results:
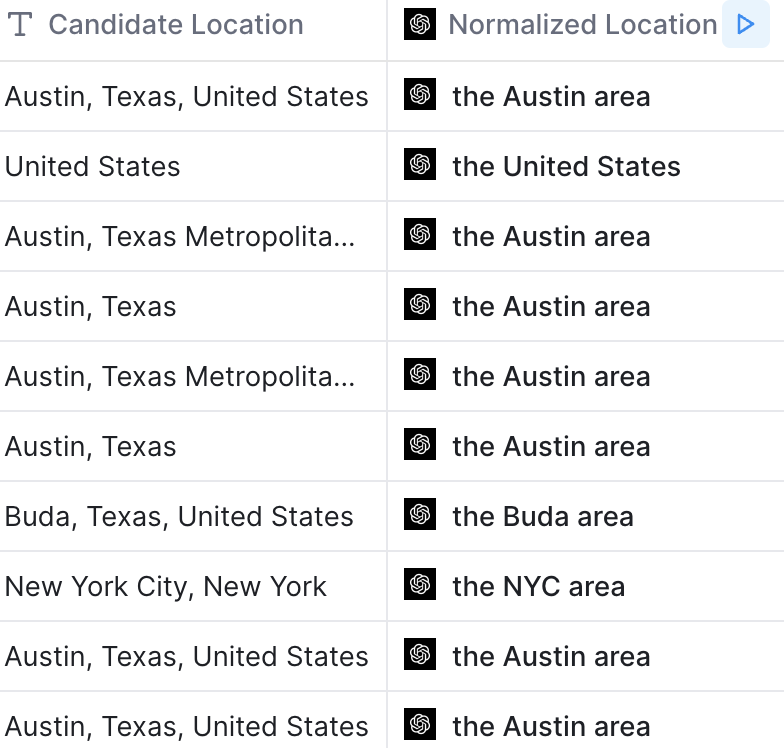
Building on our script example from before, you can now say:
“Hey Jacob, looks like you’ve been in marketing leadership at BeeKeeper for 2.5 years now. Still in the NYC area?”
Here’s the GPT prompt we used:
Your job is to normalize a candidate's location for use in a cold email script. You'll be given a candidate location. The candidate location usually contains the city, state, and country, but inputs will be different every time. This is the candidate location: {Candidate Location}
We want to normalize the text to fit in the following email script: "Still in {location}?" Usually, {location} output will be the name of the city or metropolitan area where the person lives. If you have a city or metropolitan area, return the name of the city or the metropolitan. For example, if the location is "New York City, New York", return "the NYC area" If the location is "Austin, Texas, United States", return "the Austin area" If the location is "United States", return "the United States." If the location is "Texas", return "Texas". Keep your response short, always under 5 words.
A Full AI Recruiting Email Sequence Template
Now we have all of the data we need to write a killer personalized cold email, using Mike Cohen’s email template that got a 31% message to meeting booked conversion rate!
Email 1: Initial outreach
Here’s the killer first email template Mike shared with us:
“Hi {first name},
Looks like you’ve been {general function} at {normalized company name} for {years in role}. I also noticed from your LinkedIn profile you’re living in {area}. Are you still with {company name} right now?
We currently have a unique opportunity for an experienced {job title you’re filling} here at {company name you’re hiring for}, one of the top {industry} companies in the world. You will be leading marketing, sales, and rev ops for new product execution and GTM strategies.
I’m offering two days and times for a quick phone call either this Thursday or Friday. I look forward to connecting soon.”
If you want to get creative with the email, you can add a personalized PS line to the end using the other data we gleaned from the profile:
“PS given your {years in marketing} and {years of leadership experience}, I think you’d be a perfect fit!”
Email 2: Stable Personality Type
The second email is meant for the “Stable” personality type on the DISC personality scale.
Stable personalities like lots of “we” language.
Here’s Mike’s script:
Hey {{first name]} - following up on my message {{previousStepDay}} about the VP of RevOps role.
What type of company are we?
Here’s an article from {{news network}} about {{topic}}:
{{link}}
And last, a few reason we should at least chat:
- {{Reason 1}}
- {{Reason 2}}
- {{Reason 3}}
Let me know if you have any availability early next week!
Email 3: Personalized Meme
Mike uses SourceWhale’s sequencing tool and data from Clay to generate personalized memes in his third email:
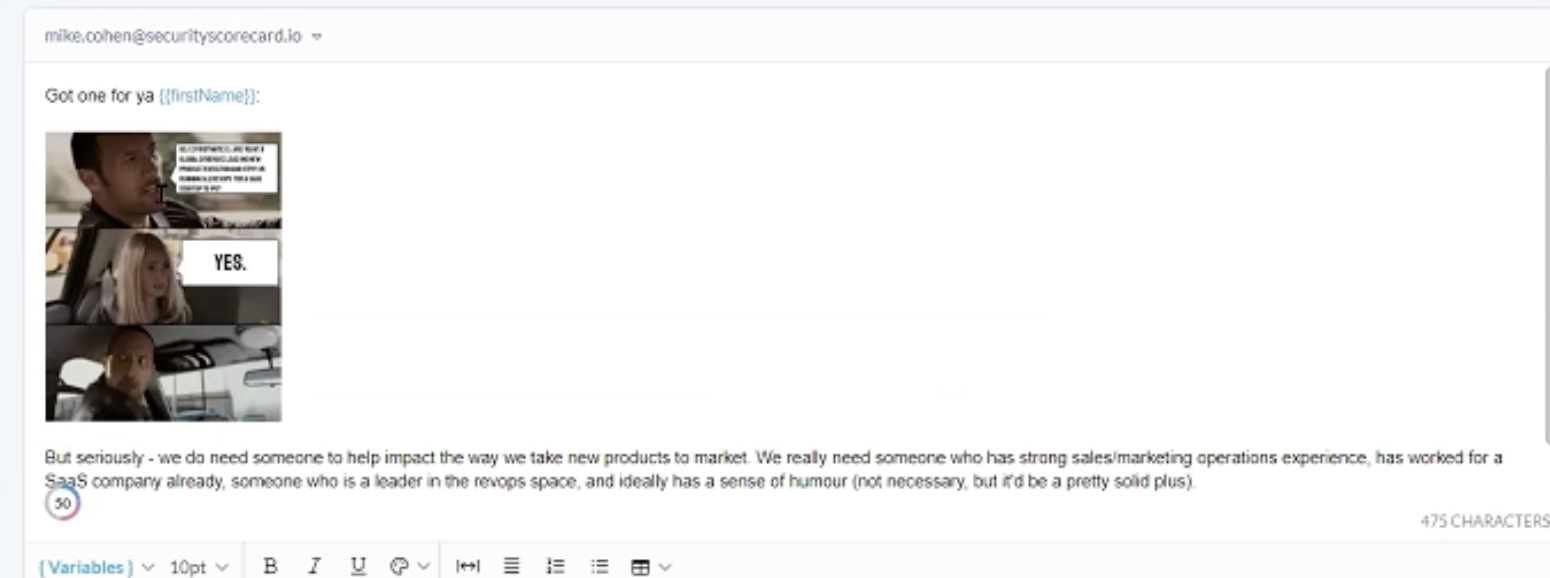
Fourth Email: Short and Sweet
The fourth email is short and sweet for people who don’t want to read:
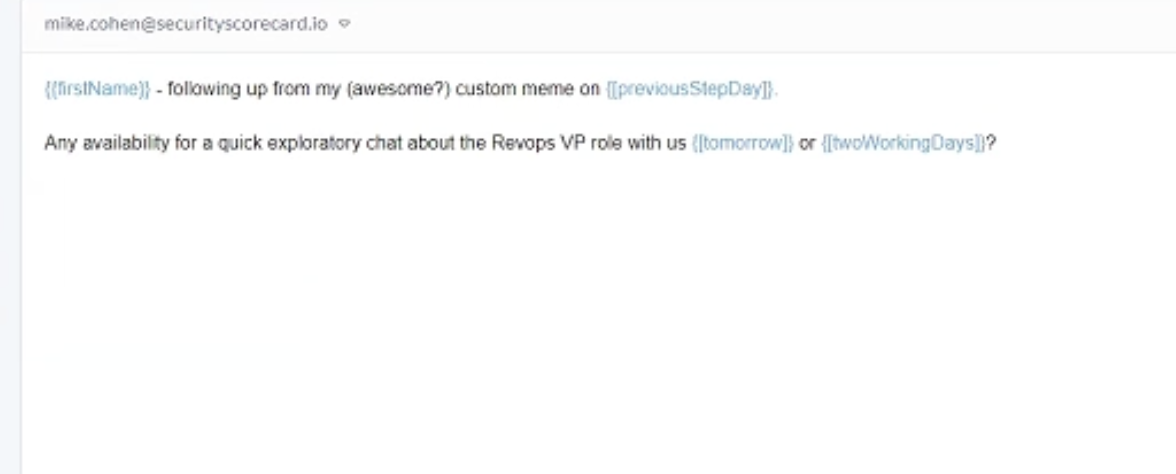
Fifth Email:
Mike ends his sequence with a picture of his dogs, for high “I” personalities.
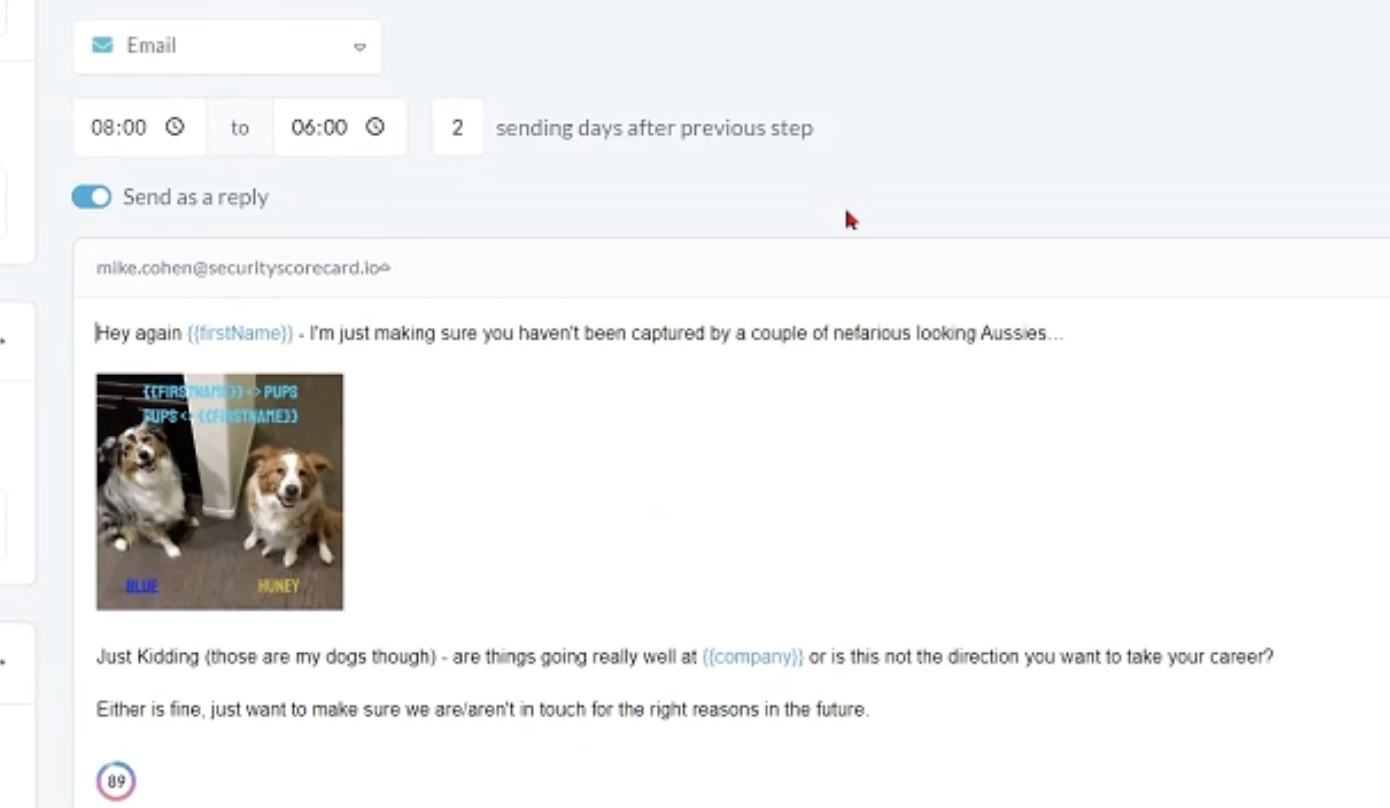
Results
The proof is in the pudding as they say… or in this case, the sequence statistics.
Using these AI recruiting strategies, here are Mike’s results for one of his sourcing clients.
One campaign:
- 102 candidates messaged
- 59.8% response rate
- 38 booked intro calls (37%)
Global client results:
- 561 candidates messaged
- 269 responses (48% response rate)
- 173 booked intro calls (31% messaged candidates booked. 64% of replies are interested)
Not too shabby, eh? Try our AI recruiting workflows out for yourself and let us know your results in the comments below 👇🏻
Using AI recruiting strategies can transform your recruiting process from slow, manual, and ineffective to blazingly fast—and better. Today, recruiting can feel like a lose-lose. People either spend unsustainable amounts of time on manual candidate research OR send generic messages with terrible response rates.
AI recruiting tools and workflows can shatter that tradeoff. Today, for the first time, you can research and write hyper-personalized messages that land candidates—without any manual work.
In this guide, we’re going to show you how, drawing upon the success of Mike Cohen—a.k.a the “Batman” of recruiting.
Mike routinely books calls with 33% of the candidates he emails with little to no manual work. We’ll walk through Mike’s entire end-to-end AI recruiting process, including the exact scripts and strategies he uses.
This guide includes:
- What’s wrong with the recruiting status quo
- How to use artificial intelligence to automate your manual research
- How to use artificial intelligence to personalize emails
- How to build Mike’s AI recruiting strategy in Clay, complete with email scripts and GPT prompts
The future of recruiting is intelligent and personalized at scale. Let’s dive in!
Results first: What can AI recruiting do for you?
TLDR: AI recruiting strategies will save you research time, make your emails much more personalized, and land more candidates.
AI gets beautiful when you combine your automated research with clever GPT prompts to create insanely relevant cold email copy. Using this strategy, we’ve sliced our time sending emails by more than 50%—with better results.
Here’s an AI generated email we sent with a 13% positive reply rate:
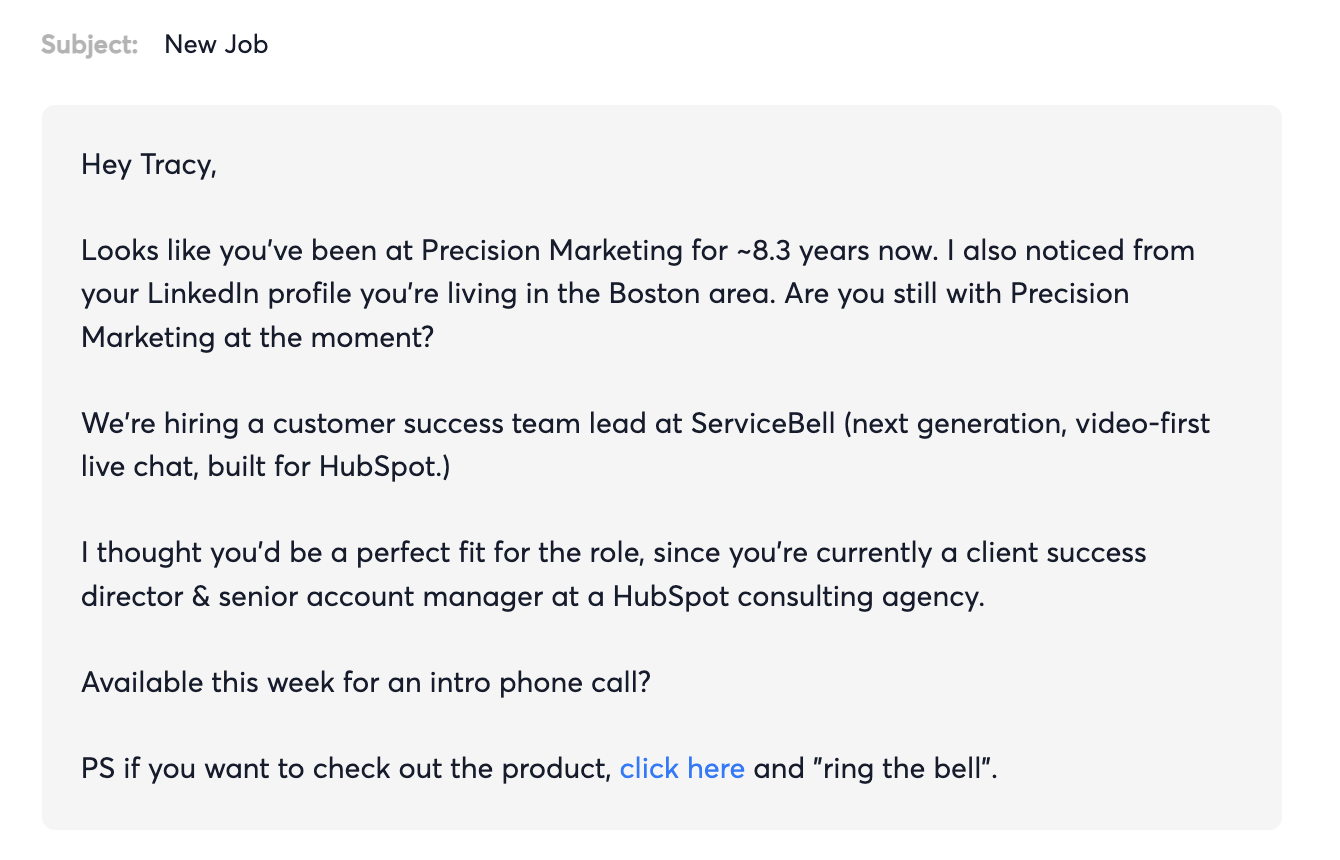
The secret to solid cold outreach is 1:1 personalization. Your candidates need to feel like the message in their inbox was written just for them.
There’s no way someone receives that email and thinks it was sent to 100 other people.
To write it, we just scraped the candidate’s title, company, location, and time in their role, and massaged the data with GPT
On the other hand, here’s one of the “best” recruiting cold email templates you’ll find on Google:

It’s totally generic, doesn’t look personalized at all, and will likely be ignored by quality candidates.
Why recruiting today is broken
There are two main parts of recruiting: first, sourcing & initial outreach, and second, continuing interactions and interviews with quality candidates.
We’ll focus our guide on sourcing, which is the first step of recruiting. Sourcing focuses exclusively on identifying and engaging the best candidates through research and outreach.
Recruiting teams today struggle with two critical issues.
No metrics or tracking
Sourcing is a nightmare to measure and track. Unless you’re terrific at spreadsheets, sourcers have zero visibility into any of the work their team is doing until the message is sent.
Because teams can’t track what outreach strategies and channels are working, they often fly blind—unable to measure performance or improve their own efficiency. And that means they use broken processes to message unqualified or uninterested candidates.
Most candidate sourcing teams, e.g., don’t know how many:
- Searches ran
- Profiles returned
- Profiles were checked
- Potential candidates were identified
- Potential candidates were messaged
- Suitable candidates replied
- Intro calls were booked
Generic, ineffective messaging
Sourcers rarely have time for detailed research on potential candidates' backgrounds, skills, and motivations. That means they lump candidates into massive, generic outreach campaigns—with messages that sound robotic and spammy.
It makes sense. Every email you’ve seen in your inbox and instantly deleted all have one thing in common: it was generic. The email wasn’t written for you. And if the sender didn’t take the time to learn about you, why would you take the time to learn about them?
Potential candidates must feel like they are getting the message from you. If candidates feel they’re getting the message because they have a specific title and some buzzwords on their profile, and the message was sent to 200 other people, they won’t respond.
Writing personalized messages to the most suitable candidates used to require tons of time and effort—until AI recruiting tools came along.
How AI Recruiting strategies work
Using AI in your recruiting workflows can help you send hyper-personalized messages at scale—and track your success with real metrics.
AI recruiting strategies can help you:
- Filter for tons of qualified candidates
- Automate all your manual research — including everything about someone education, work history, specializations, and more
- Write personalized sourcing emails at scale (with a 30% message to book rate)
Let’s go through each step to get you started with the use of AI in your recruiting process.
Step One: Automatically Find Qualified Candidates with AI
Your AI recruiting journey starts with sourcing a curated list of people to reach out to.
There are two ways to do this. Consider the below:
- Search A returned 300 people, but we only messaged 25 people.
- Search B only returned 80 people, but 35 were worth messaging
Search B is far superior—saving everyone time and money.
Filter for the right list, so your team can spend more time messaging good candidates instead of sifting through poor profiles.
Find Candidates from LinkedIn
Clay has access to LinkedIn’s entire database…which means you can run your boolean searches from Clay without spending tons of money on LinkedIn Sales Navigator.
You also don’t have to manually extract candidate data from Sales Navigator after searching or use a scraping tool. Clay gives you the data in spreadsheet form off the bat.
Sign up for Clay and click New Table > People > Find People from LinkedIn.
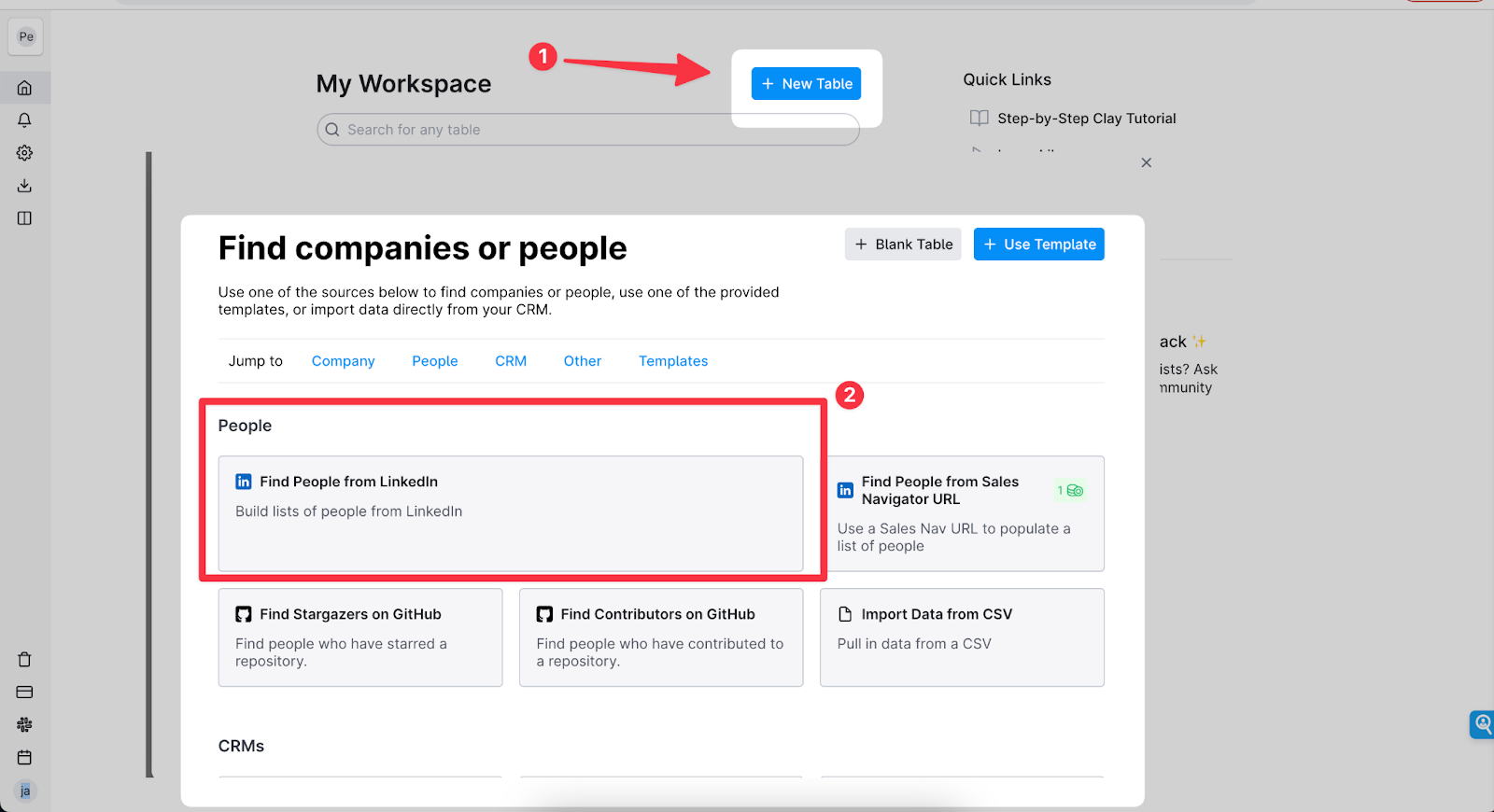
Next, enter your search criteria. In our example, we’re looking for marketing leaders with at least two years at their current company, in the San Francisco area.

You can filter by minimum or maximum months in a specific role, number of jobs, keywords in their job descriptions, keywords in their about section, and more.
Next, we’ll import our results to a New Table:
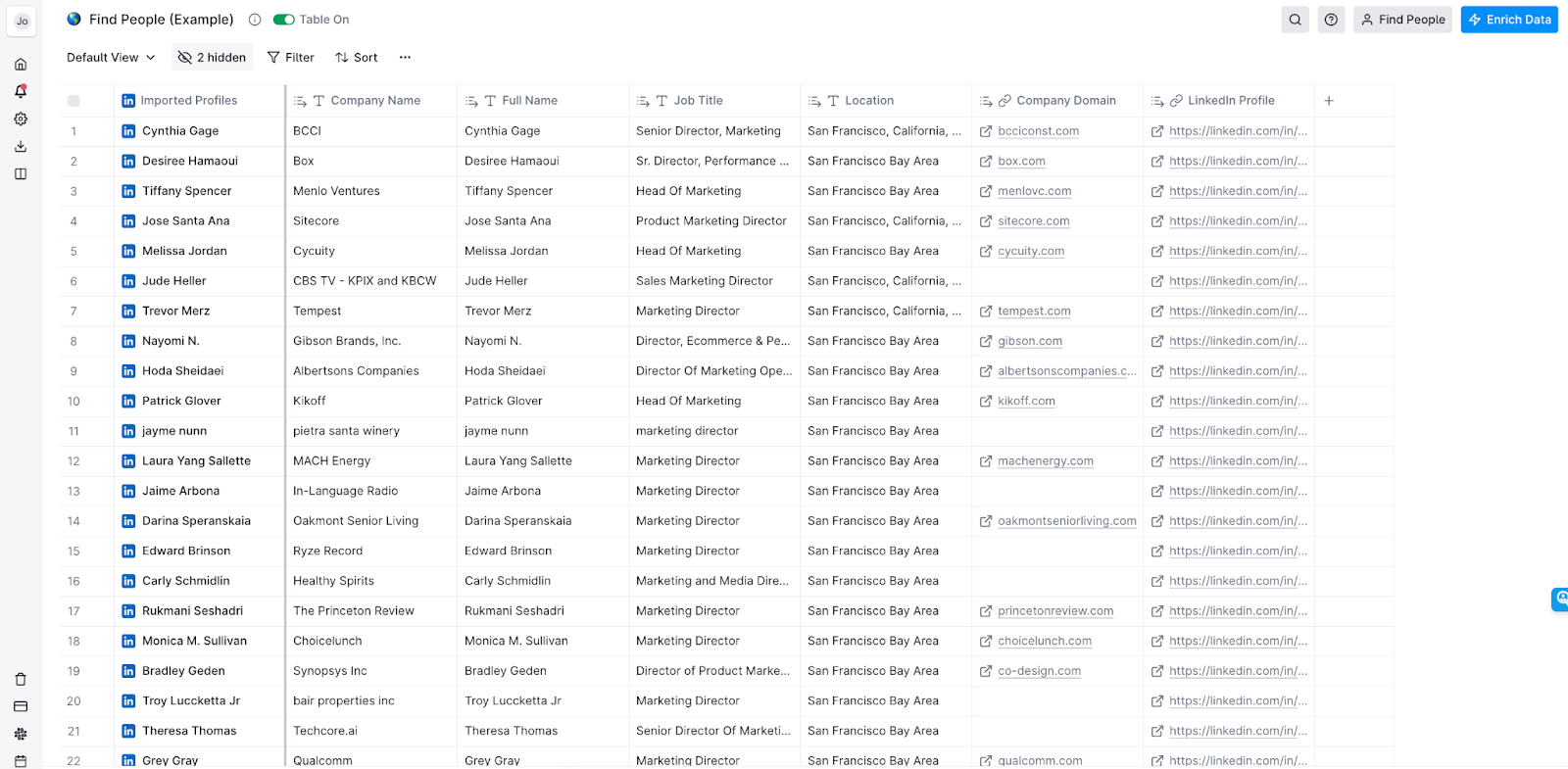
Extract Candidate Profile Data
With all of your candidates now in a Clay table, it’s time for further qualification.
Our basic find people search gives us a candidate’s company, full name, job title, location, company website, and LinkedIn profile URL.
More candidate data is available on LinkedIn, like education, current company industry and employee count, prior experience data, etc.
You can use the “Enrich Person from LinkedIn Profile” integration to unlock the extra data in your Clay table:
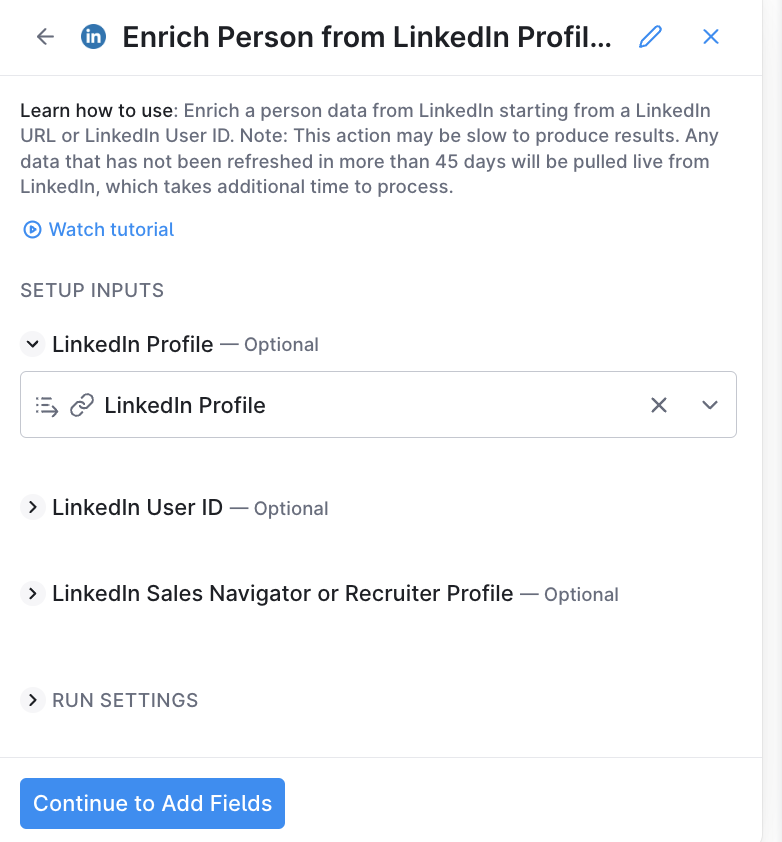
Now you have access to all of their LinkedIn data in Clay:

In this example, we want to know more about the candidate’s prior experience and education, so we’re going to pull that data out into our Clay table as individual columns.
Click on the “Enrich Person from LinkedIn” cell to open the data menu on the right, and select “Add as column” to create a new column:
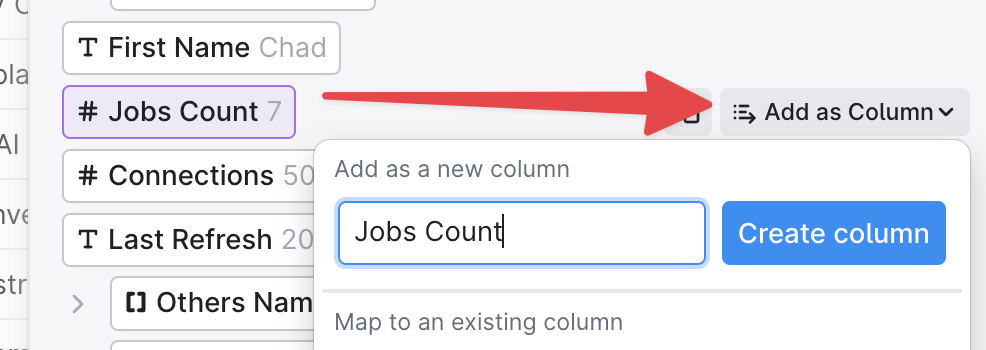
Create new columns for all the information you want in the table.
Here’s our example:
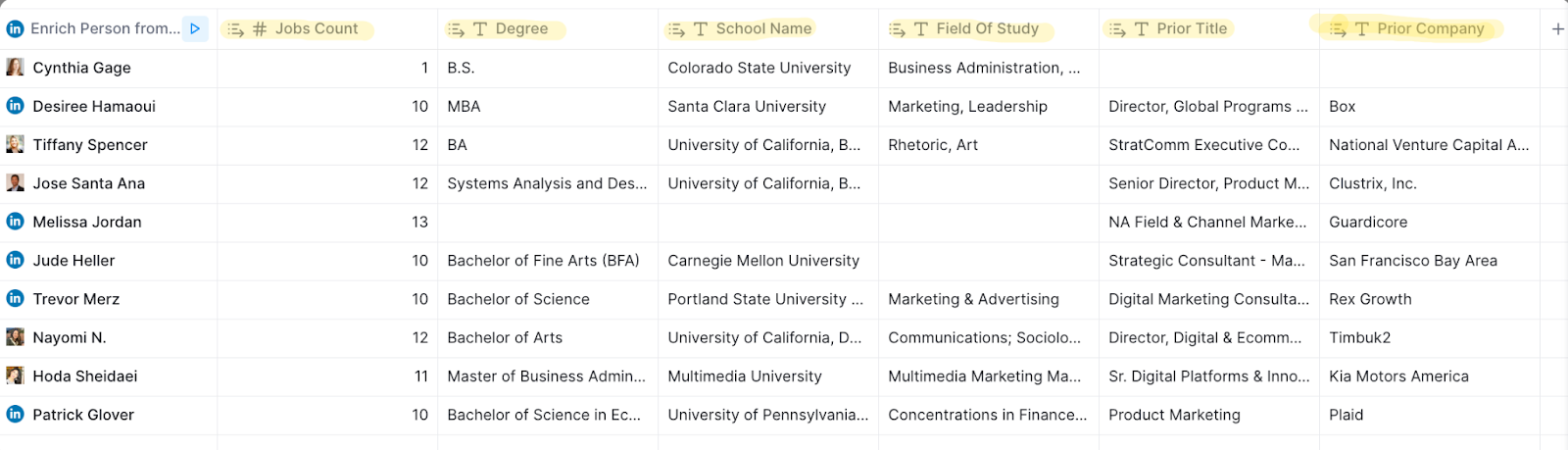
Extract Candidate Company Data
It’s often helpful to know information about your candidate’s current employer, like employee count and industry.
We can grab that information with the “Enrich Company” LinkedIn integration:
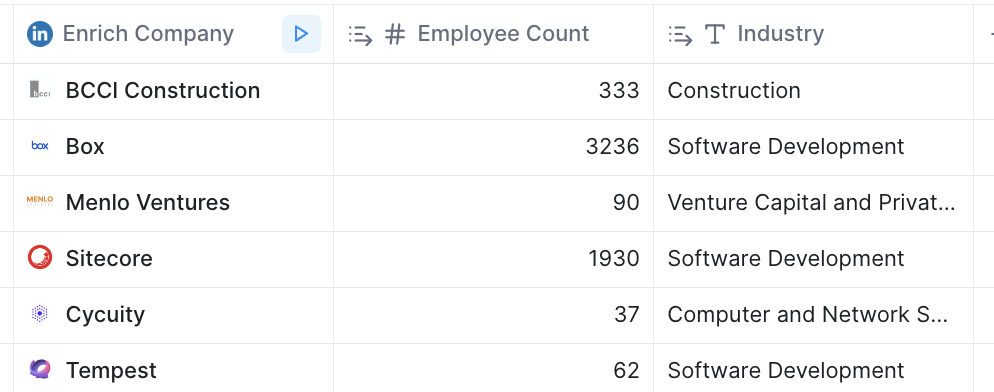
Pro Tip: AI can help determine each specific company’s industry
If you’ve used LinkedIn before, you know their industry categories are pretty broad.
In the example image above, “Tempest” and “Box” are both categorized as “Software Development” companies.
But Tempest is a “secure browser”:
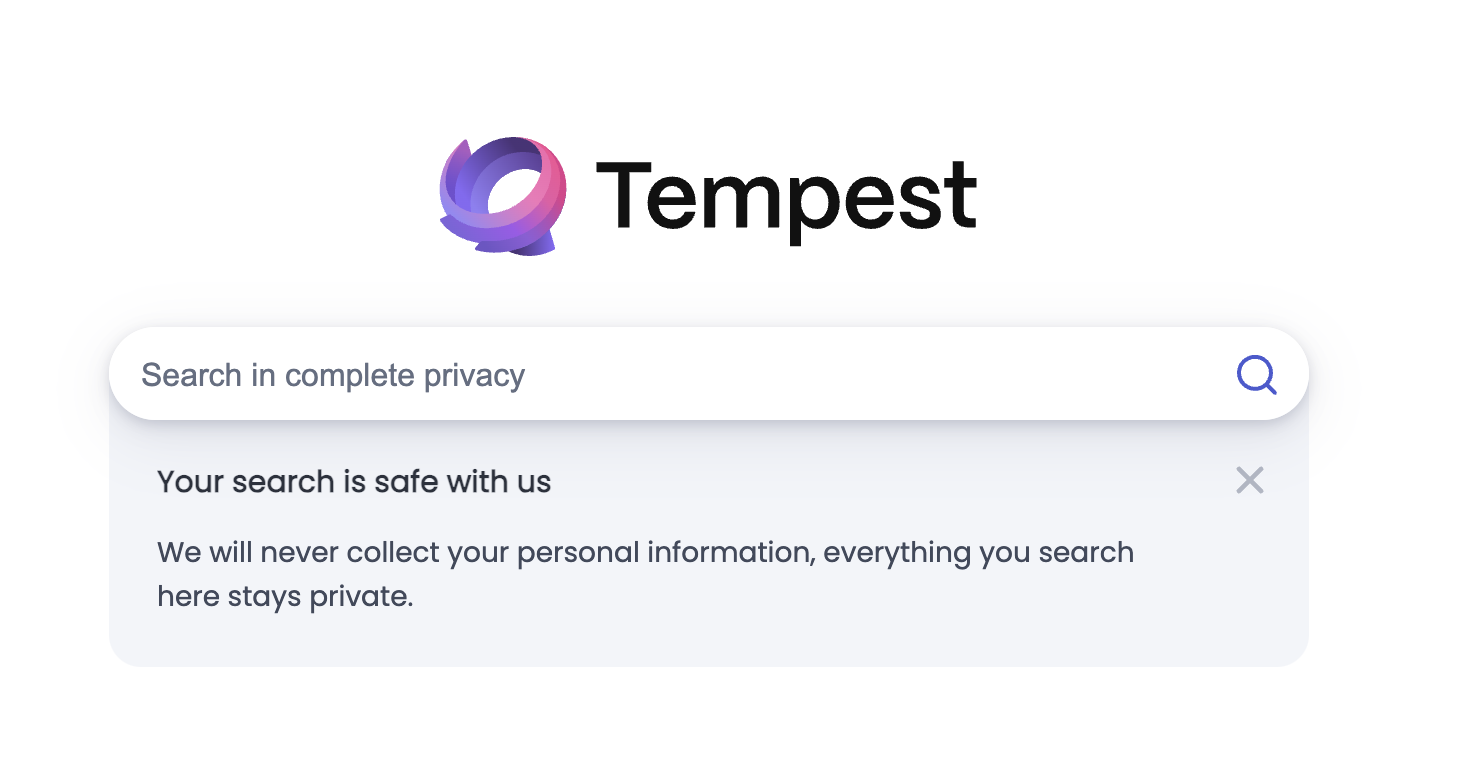
Box is a workplace collaboration software:

Moreover, custom software development companies are probably lumped together in the “Software Development” category.
If you need to categorize companies by specific company type, you must manually check each website… unless you use Clay.
With Clay, you can automate this manual research using a GPT prompt.
We’ll use the candidate’s LinkedIn company page as input, to determine the company’s specific category.
For example, check out this list of companies. Linkedin provides the first “Industry” column. The second column is our GPT prompt output:
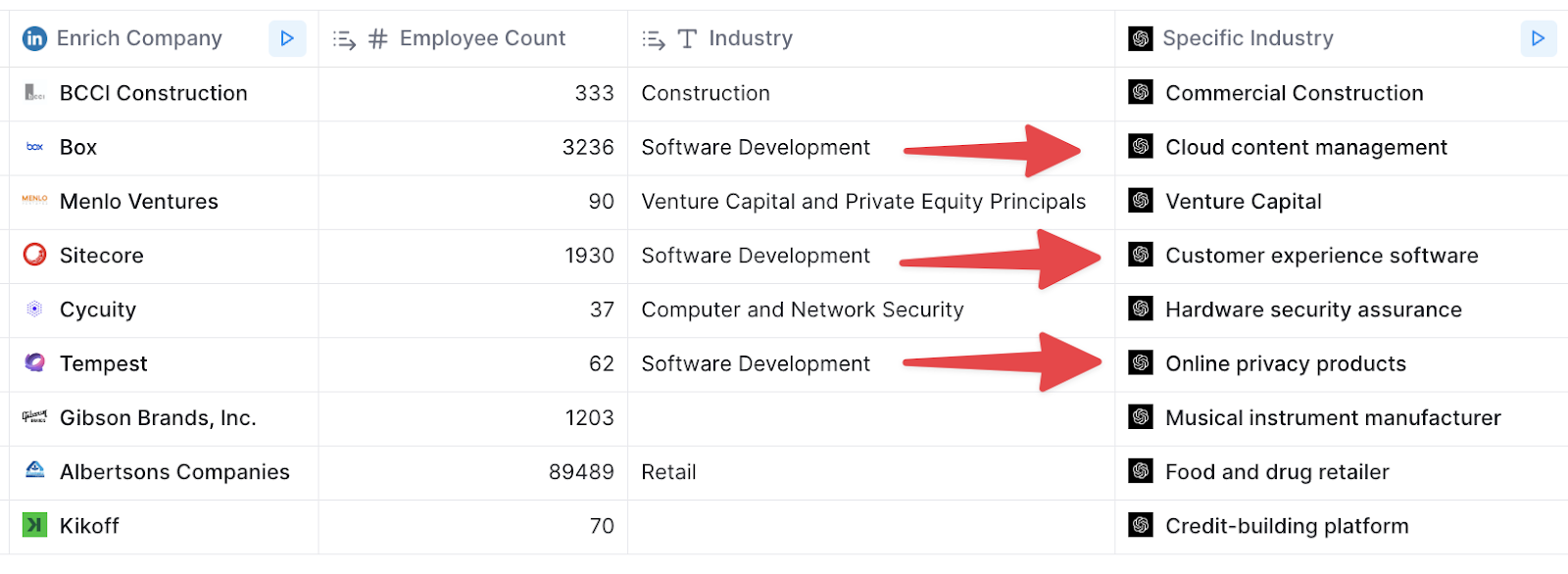
You can see how three companies, all categorized as “Software Development” had three separate specific company types.
If you wanted to be a bit more broad and qualify any candidate with prior “SaaS” experience, you could determine if any of these companies are SaaS companies instead of the specific industry.
For example:

Without manual research, we can automatically determine if a candidate has prior SaaS experience.
Pro Tip: AI can help calculate candidates’ time in current role
We also want to know how long the candidate’s been in their current role because we don’t want to message anyone who’s been in their seat for < 2 years at their current role.
“Time in seat” isn’t available by default. However, we can create an AI formula to calculate the total duration in their current seat, by subtracting the time since their start date from today’s date. Then, we’ll filter out any candidates in the seat for < 2 years.
Click on any column, then select “Insert Right” followed by “Formula” to insert a formula column in your Clay table.
Then, we’ll write the formula and generate our output.
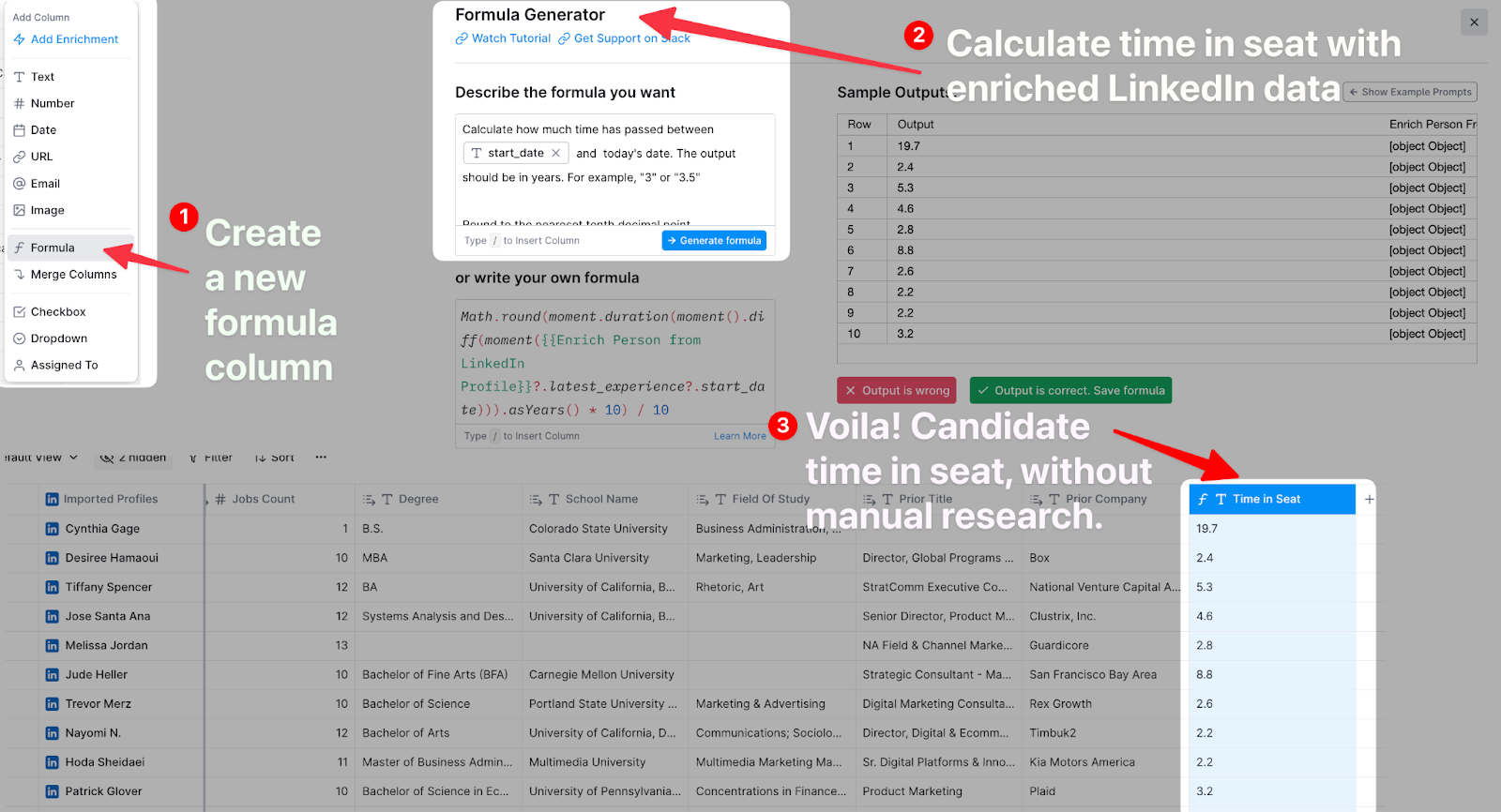
As you can see, this candidate’s time in seat (calculated in years) matches their LinkedIn profile data:
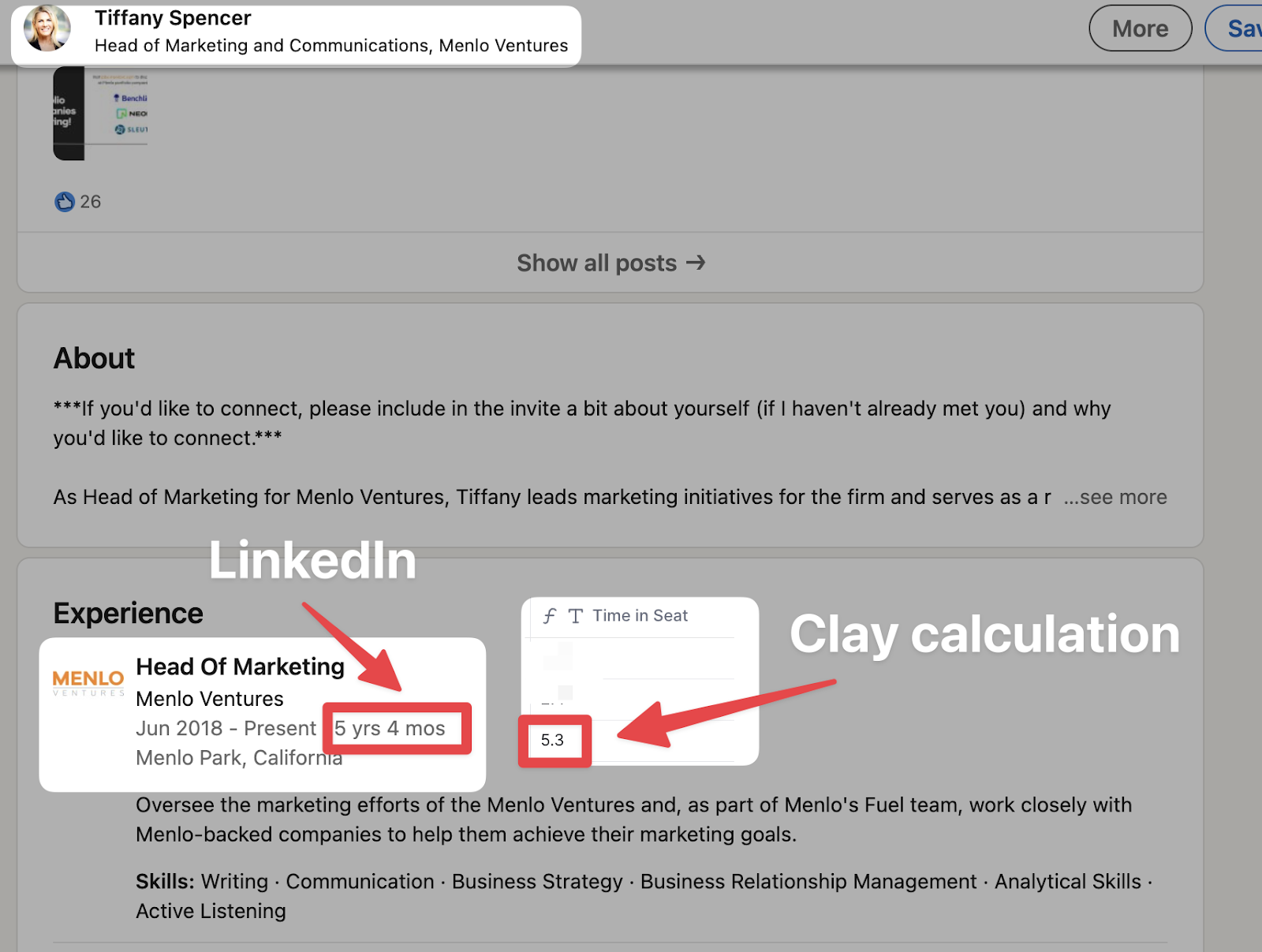
Lastly, we’ll filter to only show candidates in seat for at least two years:
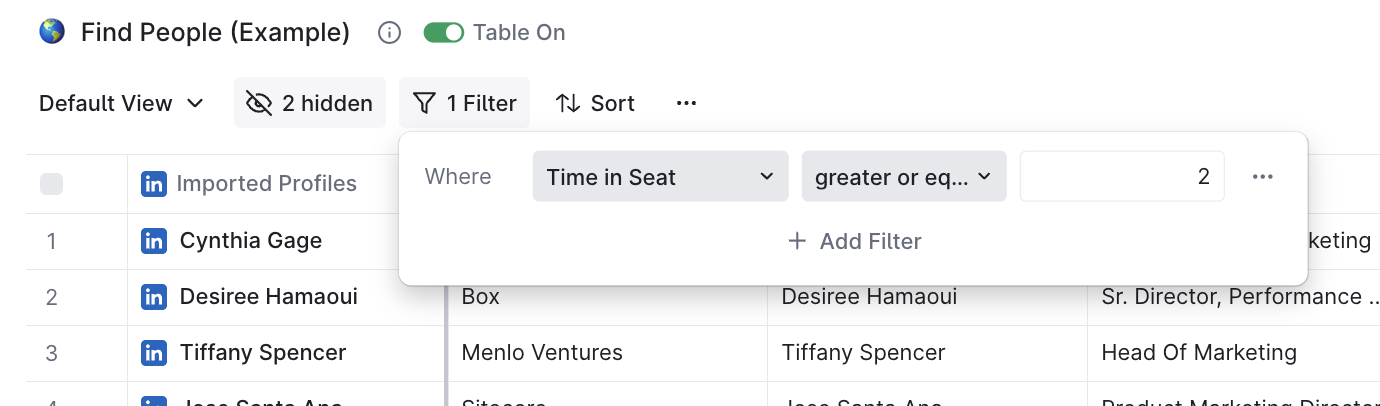
Now, without any manual research, we can automatically determine if a company has at least two years in their current seat.
Step Two: Automate your manual research with AI
Before you email a candidate, you need to know things like:
- Years at current company
- Time since the last role ended
- Current/Past company industry
- Current/past company job description
- School, Degree, Major
- Location
Without AI recruiting tools, you have two bad options to get this information. You can either spend tons of time manually checking each profile. OR you can skip the research since it takes so long, and blast messages to tons of people.
With AI, you can access all the above information without spending time on manual research. Extract the information above - and more - from candidate profiles into a Clay table, and use AI to determine whether a candidate fits your role based on any of those data points. Let’s show you how.
First: most jobs have specific requirements beyond what we’ve already shown above.
Let’s use this VP of Marketing position on Indeed as an example:
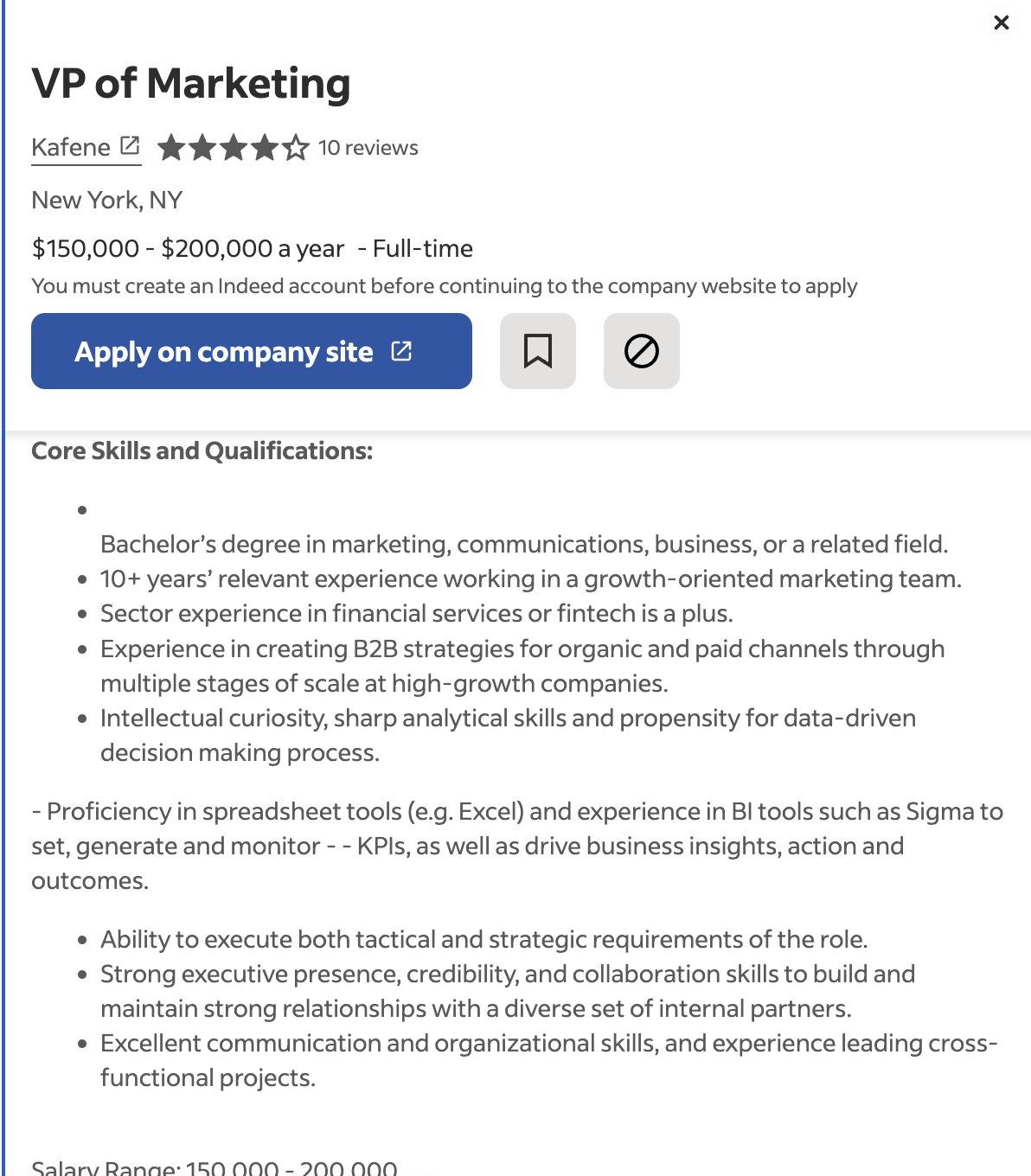
The role requires:
- At last 10 years of marketing experience
- At least 2 years of leadership experience
- At least a bachelor’s degree
The role also lists some “nice to have” experience:
- Worked in the finance industry
- Specific technologies (Excel, Sigma, HubSpot)
Lastly, the candidate ideally has experience relevant to the job’s core responsibilities:
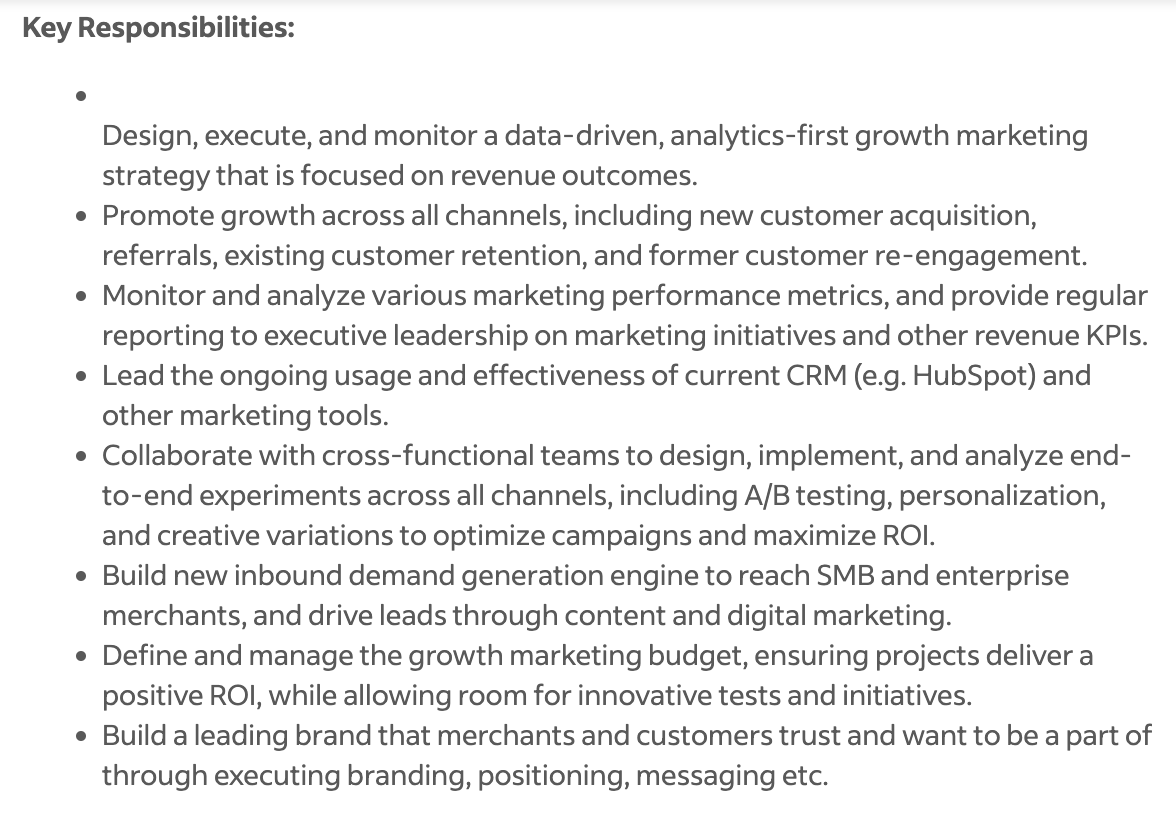
Before ChatGPT hit the market, sourcers had to manually research every candidate’s profile to answer these questions… or send a message to many unqualified candidates.
With Clay and ChatGPT, we can automate this research with a clever prompt.
NOTE: This is for advanced Clay users. If you’re a beginner, you may want to skip this step or reach out in our Slack community for help.
Job Requirements (Advanced)
GPT is only as powerful as its prompt and input data. The more specific you can be with your prompt, the better the output.
In our case, we’ll use GPT to ensure candidates satisfy our job requirements. Later, we can use GPT to see how well a candidate’s experience fits our job responsibilities.
We’re looking for experience in marketing, experience in leadership, experience with specific technology, and at least a bachelor’s degree.
Here’s the prompt I gave ChatGPT:
You are a recruiting sourcer looking at candidates' LinkedIn profiles to understand their past work experience and see if the candidate's background makes them a good fit for the job based on the job requirements (needs) and job "nice to haves" (wants). You will be given a list of job experience mandatory requirements, "nice to have " requirements, and tech stack experience requirements. Your job will be to scrape a LinkedIn profile, and see if the candidate meets the list of requirements. Here are the job experience mandatory requirements:
- Minimum education: bachelor's degree or greater
- Minimum years experience in marketing: 10 years
- Minimum years experience in a leadership role: 2 years
Here are the "nice to have" requirements:
- Background in finance
Here are the technologies the candidate will need to configure:
- HubSpot
- Excel
- Sigma
Candidate's LinkedIn profile:{Enrich Person from LinkedIn Profile}
You will return back your findings in JSON format.
You are now going to be given a list of questions. Each question has the JSON key in () afterwards.
- Does the candidate meet the minimum education requirement? (minimum_education_requirement) - What is the candidate's level of education? (education_level)
- Does the candidate have the minimum marketing experience? (minimum_marketing_experience)
- How many years of marketing experience does the candidate have? (marketing_experience_years) - Does the candidate meet the minimum number of years in a leadership position? (minimum_leadership_experience)
- How many years of leadership experience does the candidate have? (leadership_experience_years)
- Does the candidate have a background in finance? (finance_experience)
- If applicable, describe the candidate's finance background in 10 words or less (finance_experience_description)
- Does the candidate's profile explicitly mention any of the technologies they'll need to configure? If the profile does not explicitly mention any of the technology keywords, this answer is false. (technology_experience)
- If applicable, list the nice-to-have technologies mentioned in the candidate's profile, and describe where you found the technology explicitly mentioned. (technology_experience_names)
Notice the prompt is quite long. I also told GPT to return the answer in JSON format.
In a nutshell, JSON is compact and readable text formats for structuring data. If you don’t know what JSON is, this 90 second video will help.
We’re using it here to ensure a standard response is generated by GPT. Otherwise, the answers would be returned as long sentences, and it’d be impossible to consistently make use of the data.
Here’s what a GPT output looks like in JSON format:
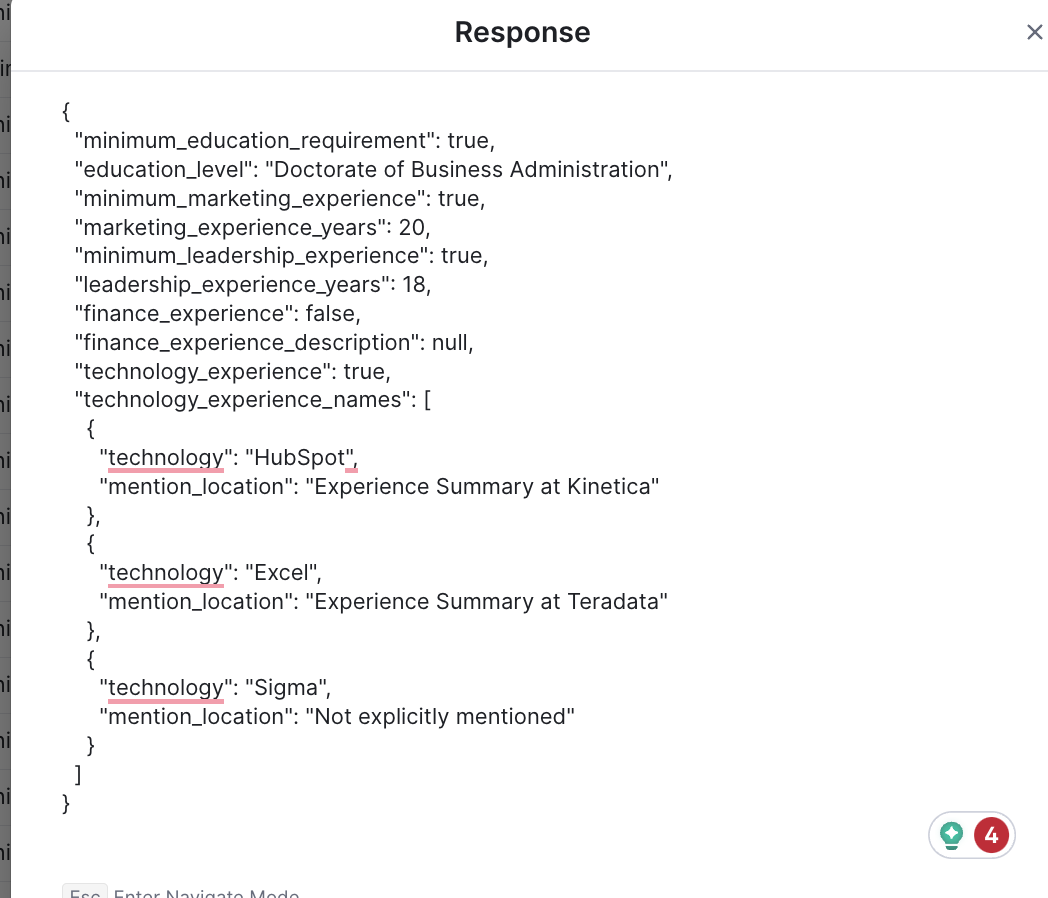
JSON makes it easy to extract data like “education level” or “marketing experience years”.
Here’s an example of candidate information without JSON format:
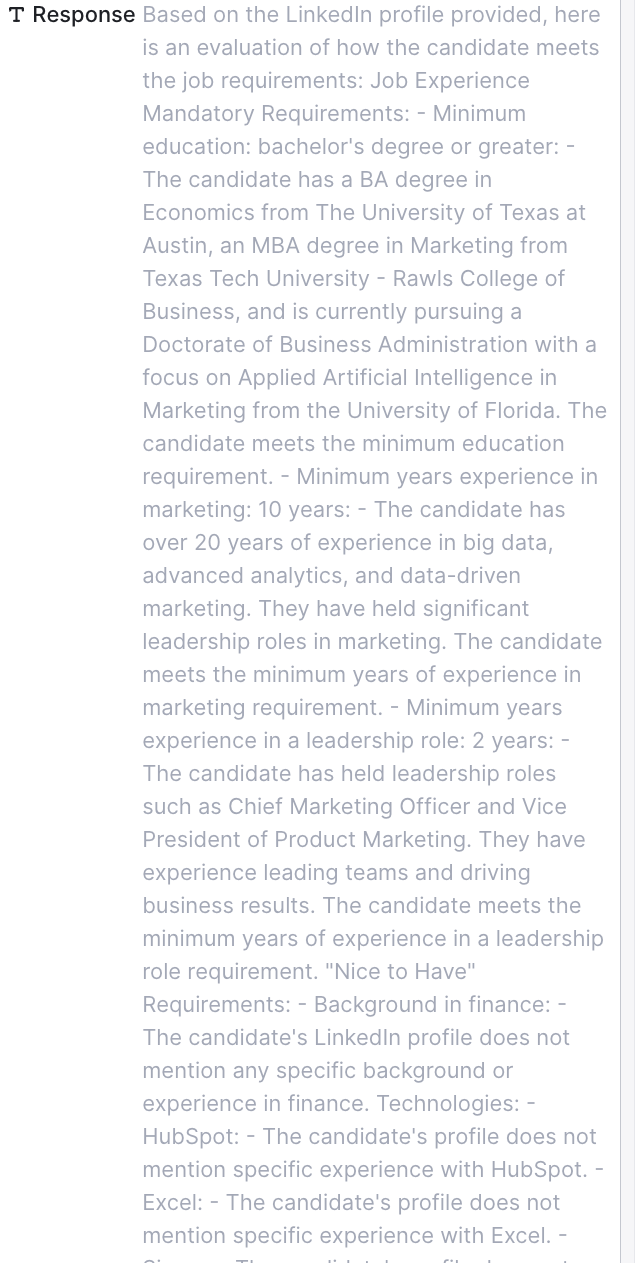
As you can see, it’d be nearly impossible to consistently glean the correct information from a bulky paragraph of text.
Once you understand JSON, it’s pretty easy to extract data and use it effectively for segmentation and personalized outreach messages.
Next, we will pull out the data from the GPT scrape to filter out candidates that don’t meet our requirements and use the scraped information in a personalized outreach message.
Extracting Data from the GPT Scrape
We can use more AI formulas to extract the information we want from GPT’s JSON output.
In this case, we want:
- Years of marketing experience
- Years of leadership experience
- Minimum education requirement met? (true false)
- Education level
- Finance experience
Let’s start with years of marketing experience.
JSON uses “keys” to store values.
For example, “marketing_experience_years” is the key, and “20” is the value. In layman’s terms, GPT determined this candidate has 20 years of marketing experience.
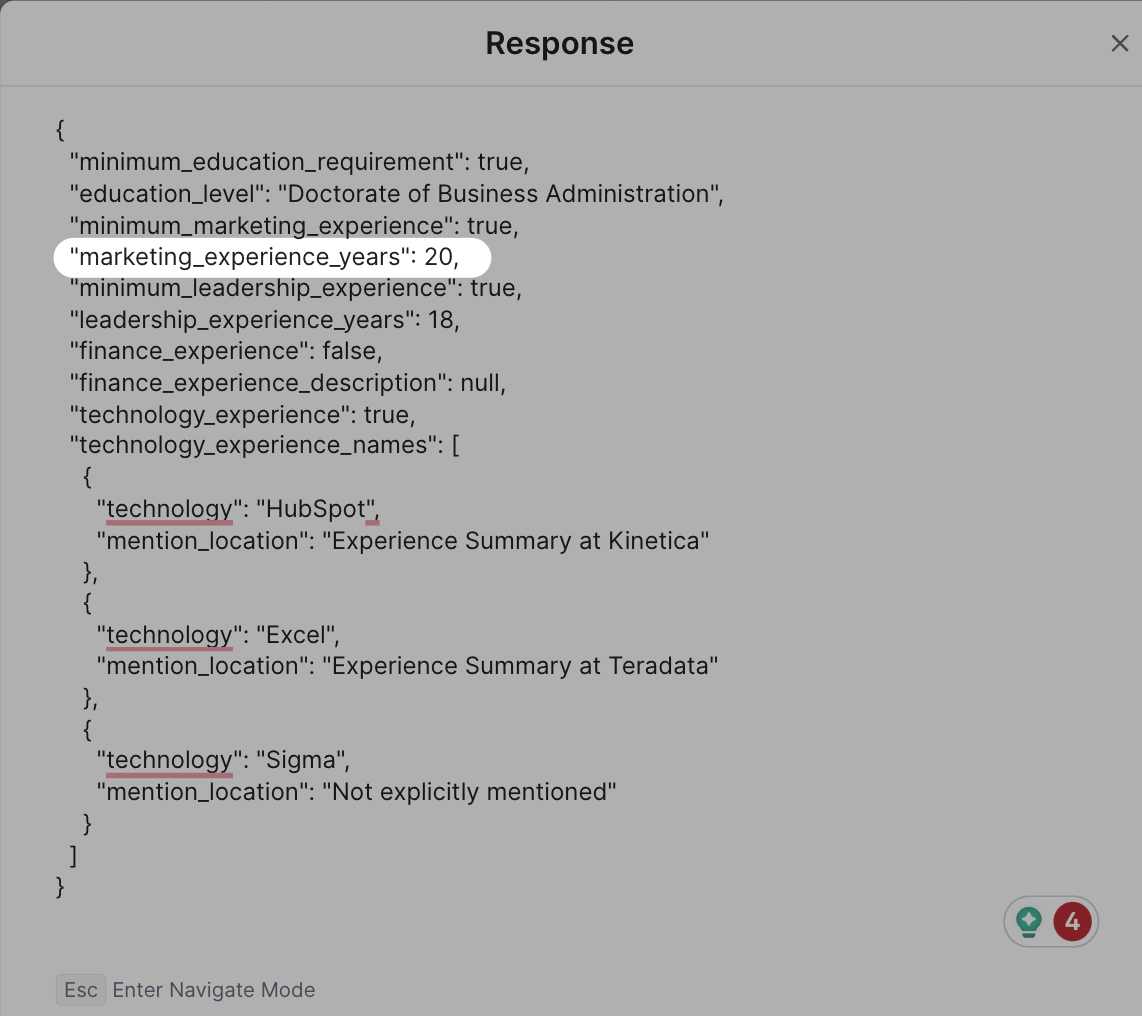
We’ll use an AI formula to extract the value (20) from the key (marketing_experience_years). This will give us a column in our Clay table populated with each candidate’s years of marketing experience.
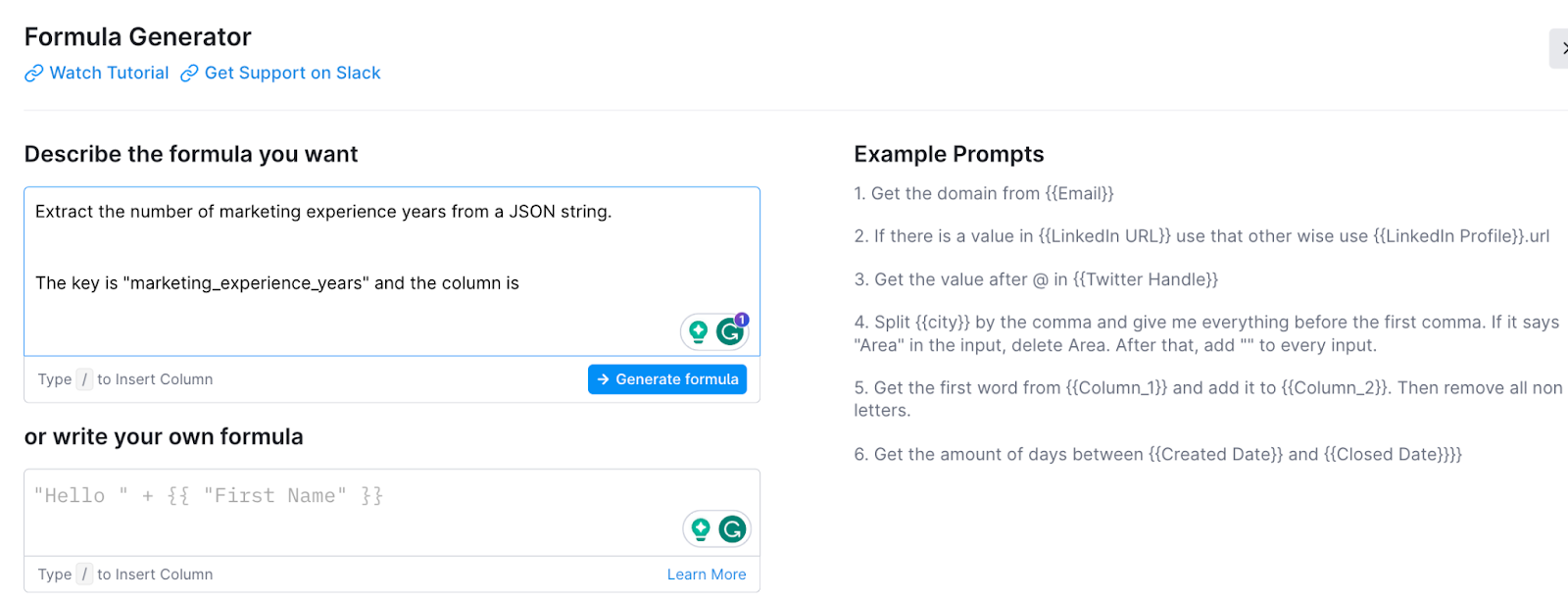
First, use the prompt above to tell the AI formula generator we’re extracting a value from a JSON string. Next, we’ll give our AI prompt the key (the placeholder for our value, in this case, marketing_experience_years).
Lastly, we need to tell the formula where to look for our key. It’s stored in the GPT response column.
We can use any data from our Clay table in an AI formula. Type “/” and then select the data you want.
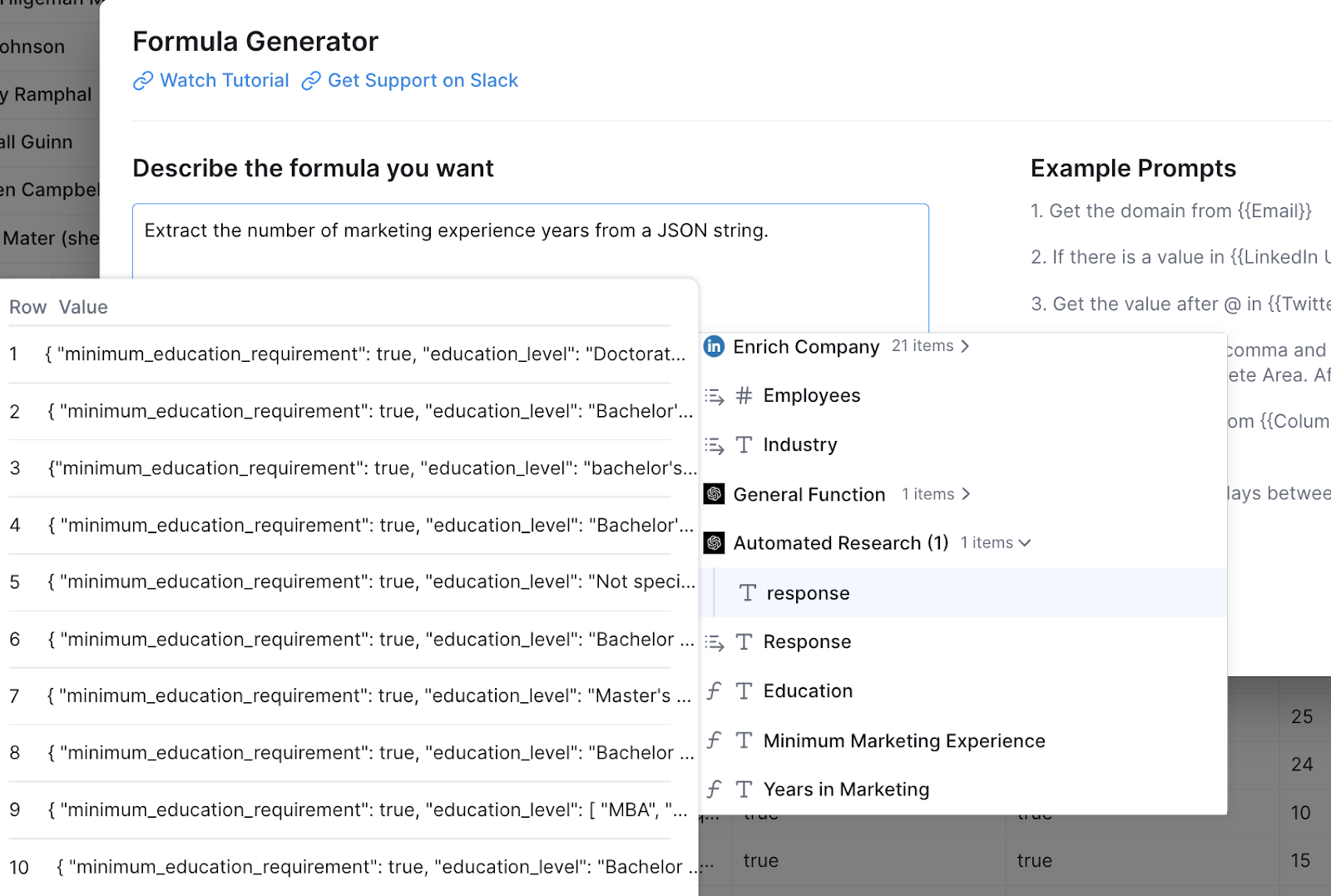
Click “Generate formula” to see a list of sample outputs, each containing your candidate’s marketing experience.
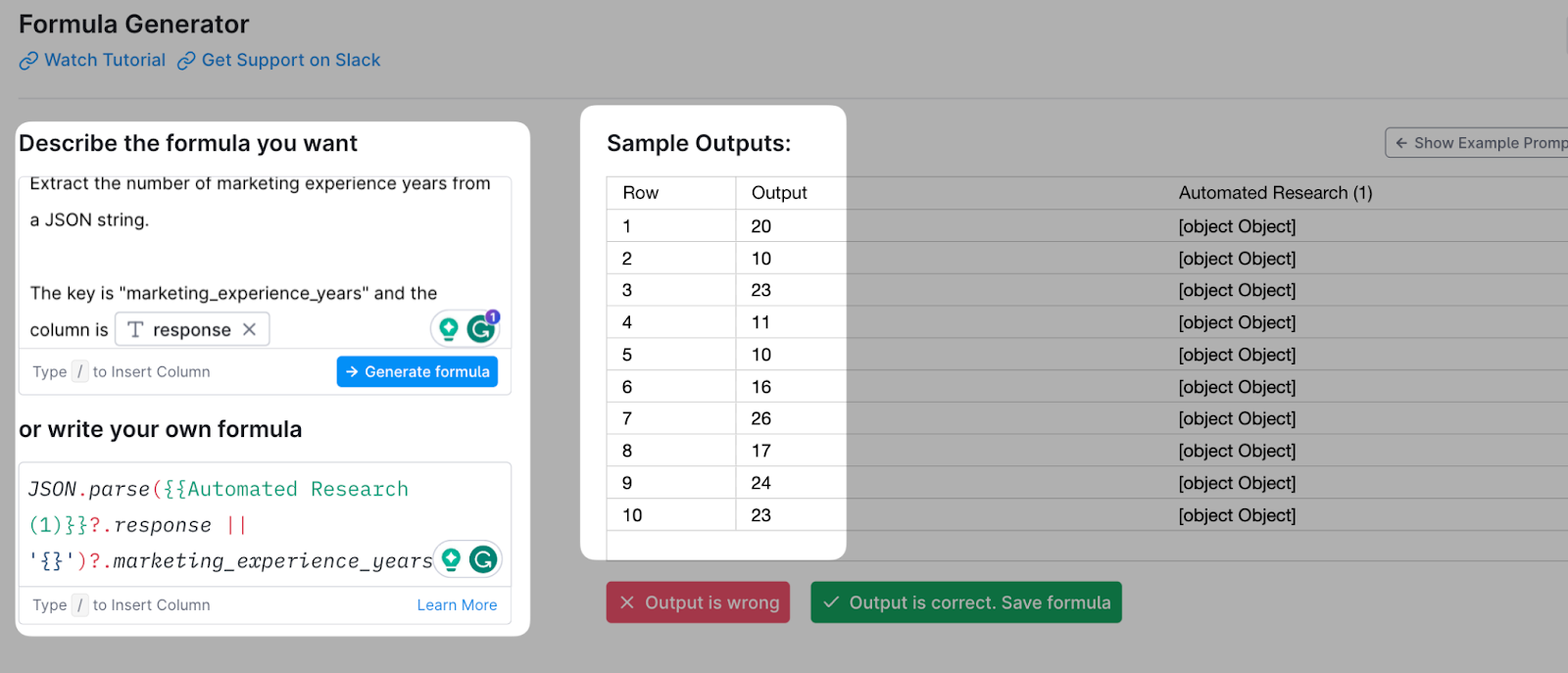
Click “Output is correct,” and you’ll have a table column of each candidate’s marketing experience, ready to filter and use in personalized outbound campaigns.
We repeated this step for years of leadership experience and education level. You can repeat the step above for any data you want from the GPT scrape.
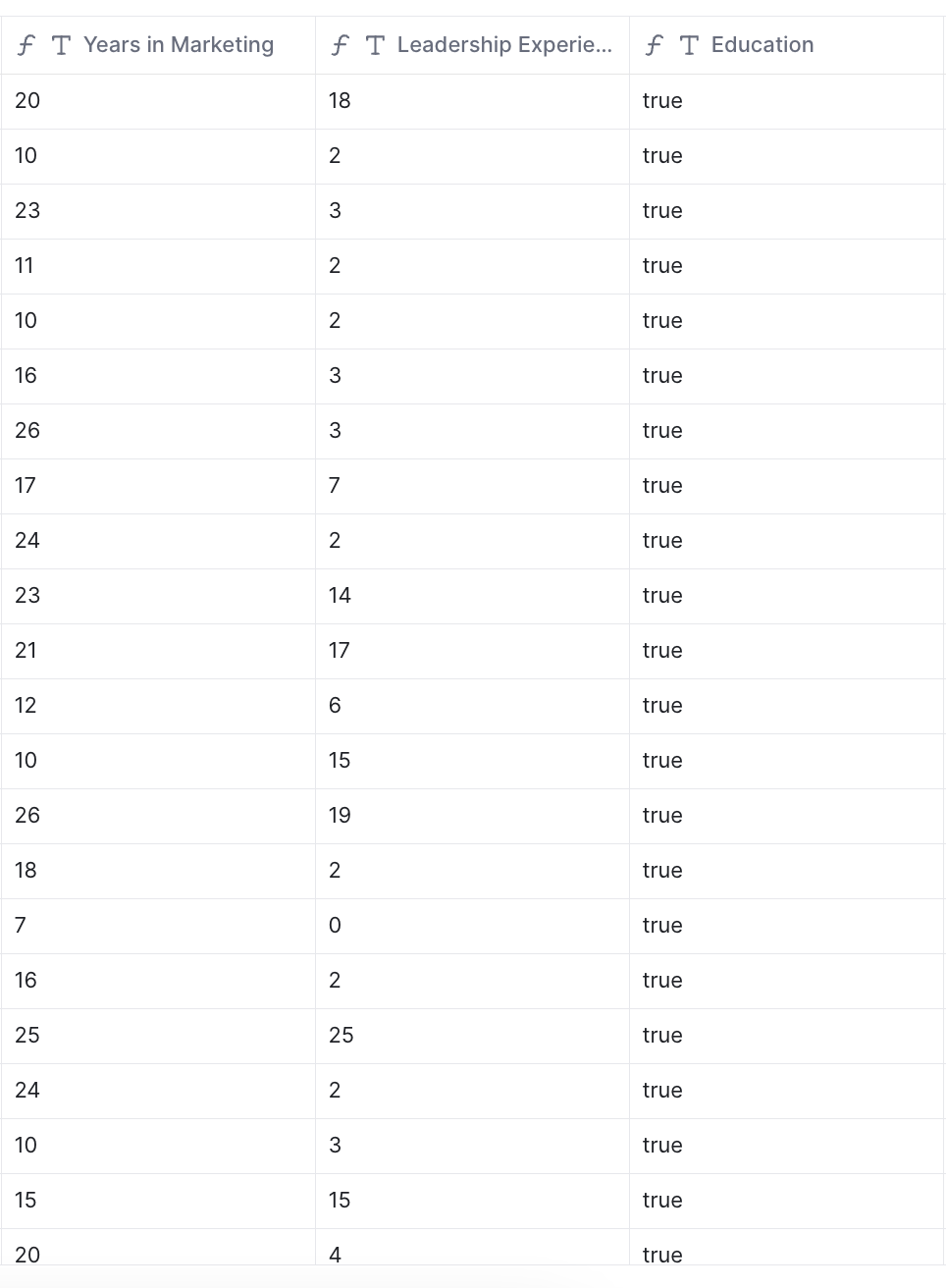
Lastly, we’ll filter candidates that meet our job requirements:

PS: By default, the formula column is a “Text” data type. If you want to filter a numerical value (like the number of years in marketing), change the data type to “Number.”
Click on the column, then click Edit Column, then change the data type to Number and save.
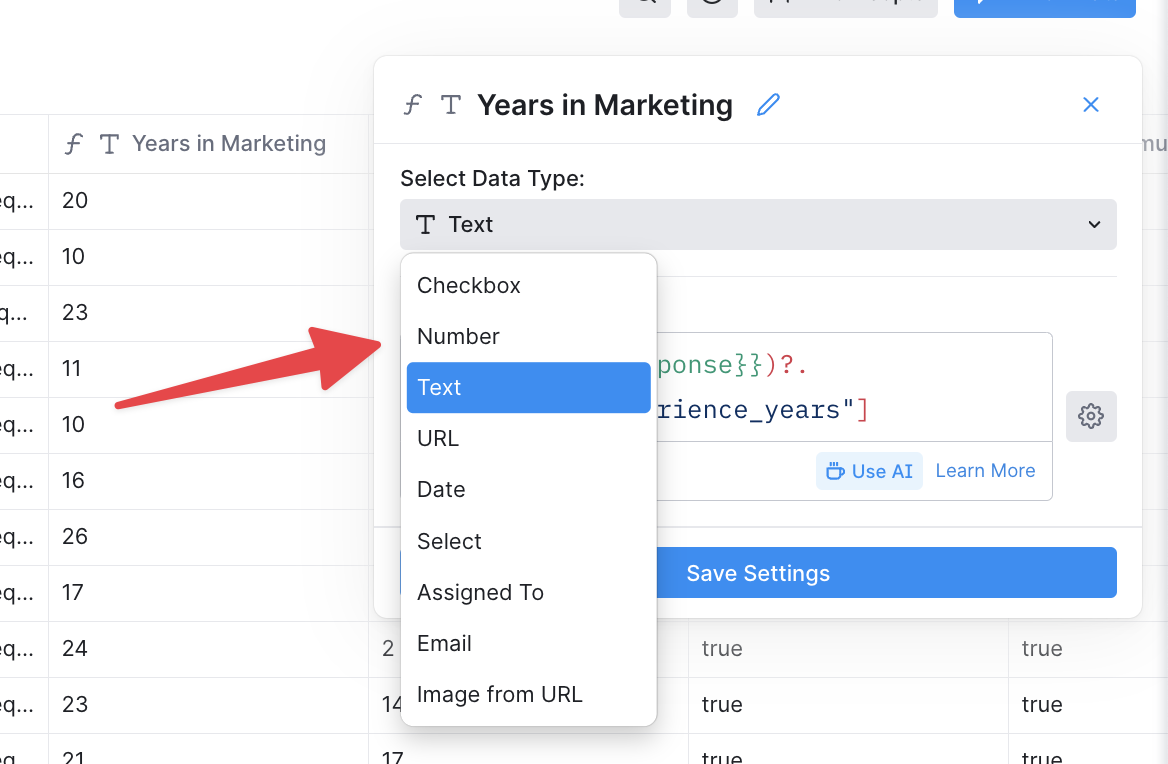
Now we’re left with a list of qualified candidates (marketing leaders in the San Francisco area with at least 10 years in marketing, 2 years of leadership experience, and at least a bachelor’s degree):
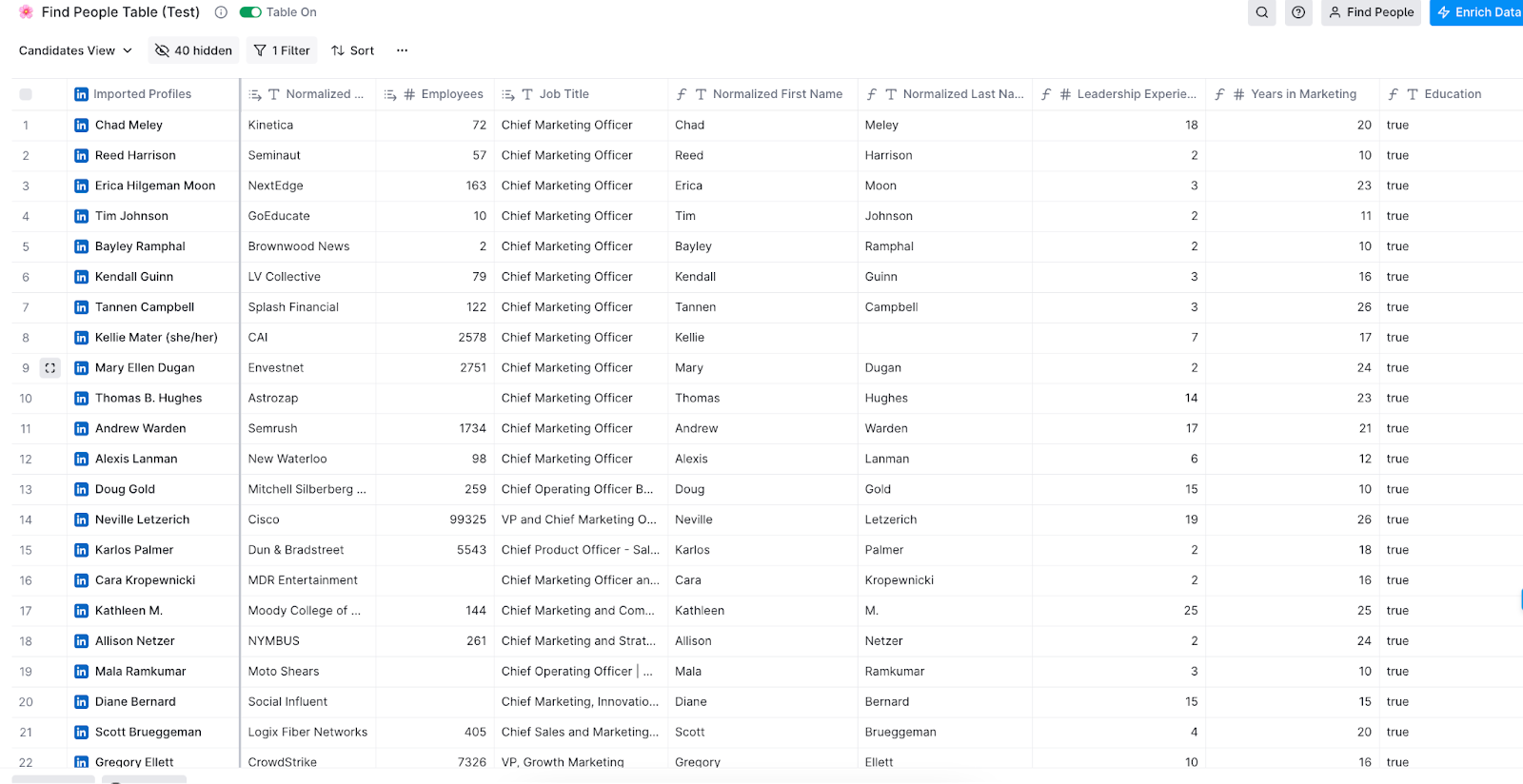
Write Qualified Candidates to New Table for Engagement
It’s time to find candidate emails. First, we’ll push our qualified candidates to a new table and find emails there, so we can separate our “Automated Research” workflow from our “Find Emails, Personalize, and Engage” workflow.
Duplicate the Clay table, and add the columns you want to populate in the new table.
We’ll use the following columns in our example:

- Company Name
- Job Title
- First Name
- Last Name
- Candidate Location
- LinkedIn Profile URL
- Company Website URL
- Company LinkedIn URL
- # Years at Current Company
- # Years of Marketing Experience
- # Years of Leadership Experience
Next, we’ll push our qualified candidates into a new table with the “Write to Other Table” integration.
Choose the new table, and map columns from your first table to the second table:
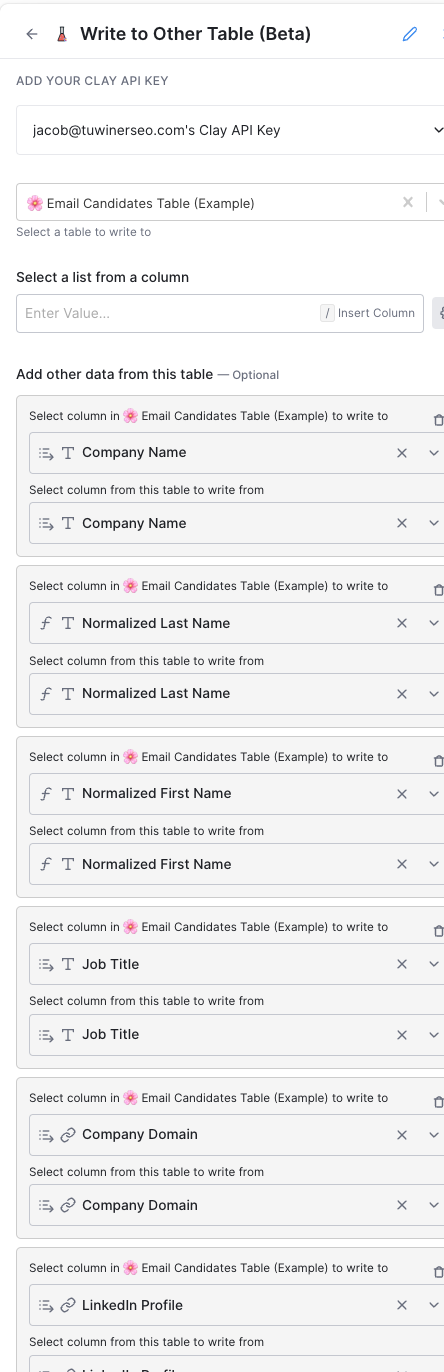
Run your rows, and you’ll see your data in the new table:
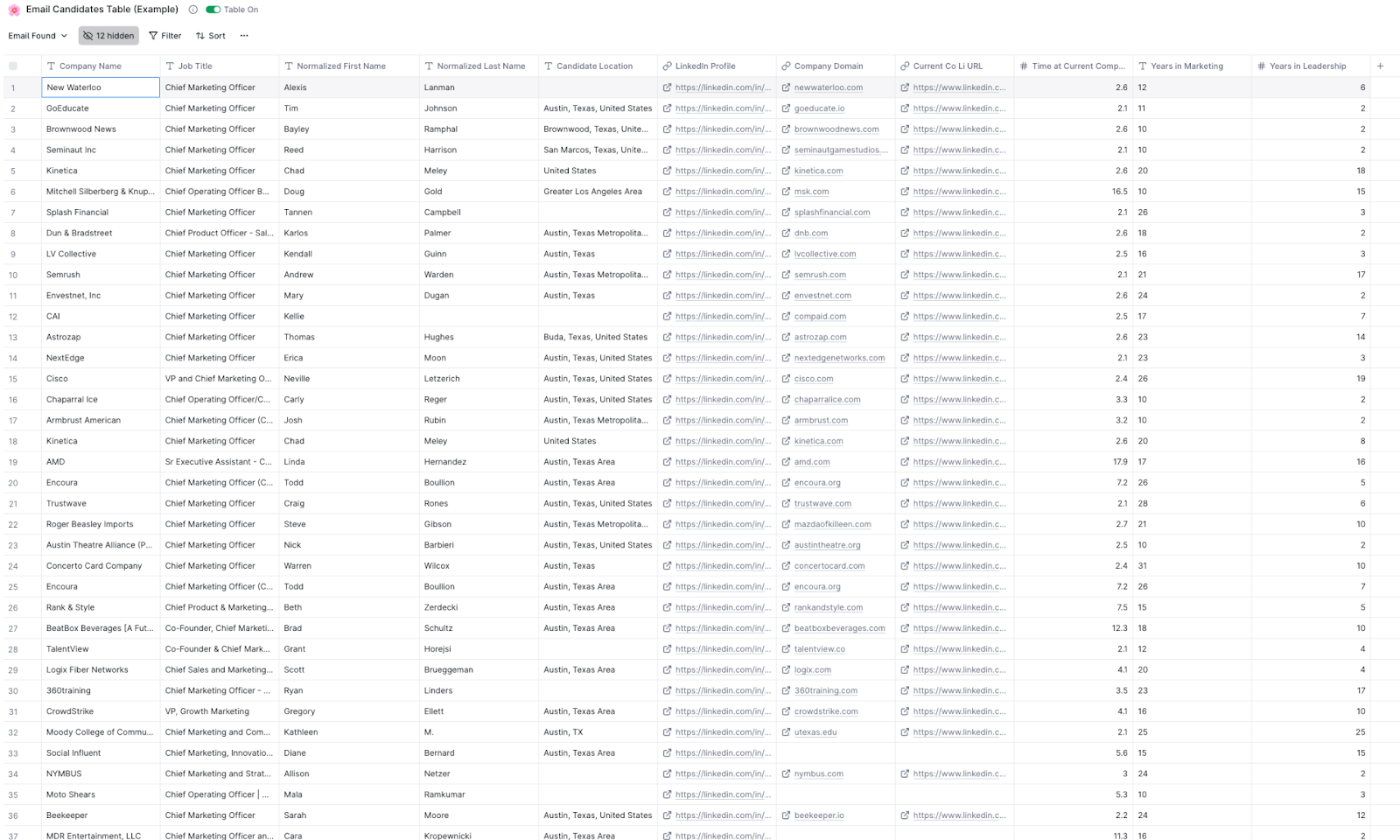
Find Candidates’ Personal Emails
We want to find personal emails, so candidates won’t worry about responding to a job offer from their employer’s email account.
We’ll use the “Find Personal Email” waterfall, which uses four data providers to find a personal email address. If one provider doesn’t have an email, Clay will automatically check the next provider until you find a match.
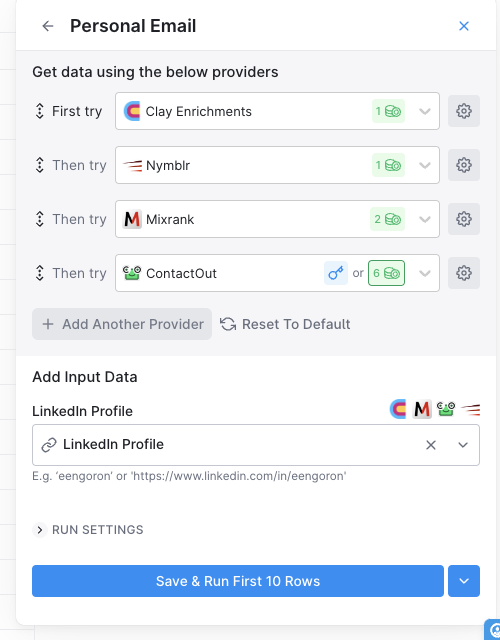
That is pretty good coverage! We found 33/37 candidate emails (89% coverage). You can also verify emails as well with NeverBounce or another provider.
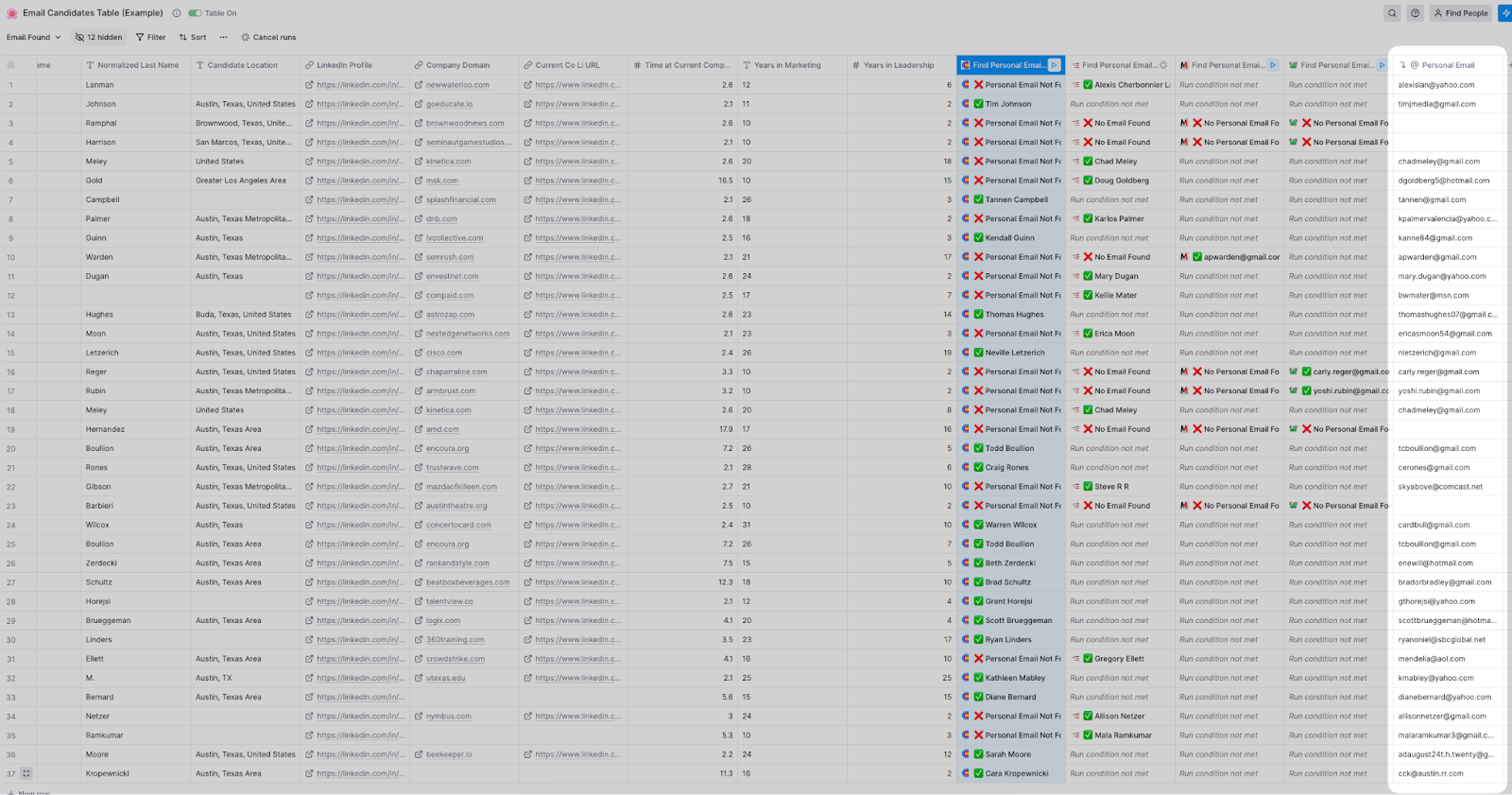
Let’s create a new table view showing only qualified candidates with verified emails.
Duplicate the view:
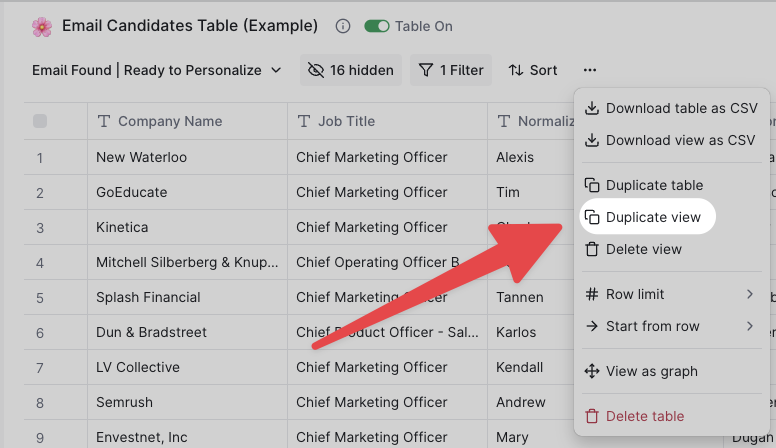
Then, filter out any rows without a “personal email” value:

Now your table shows all qualified candidates with verified personal email addresses:
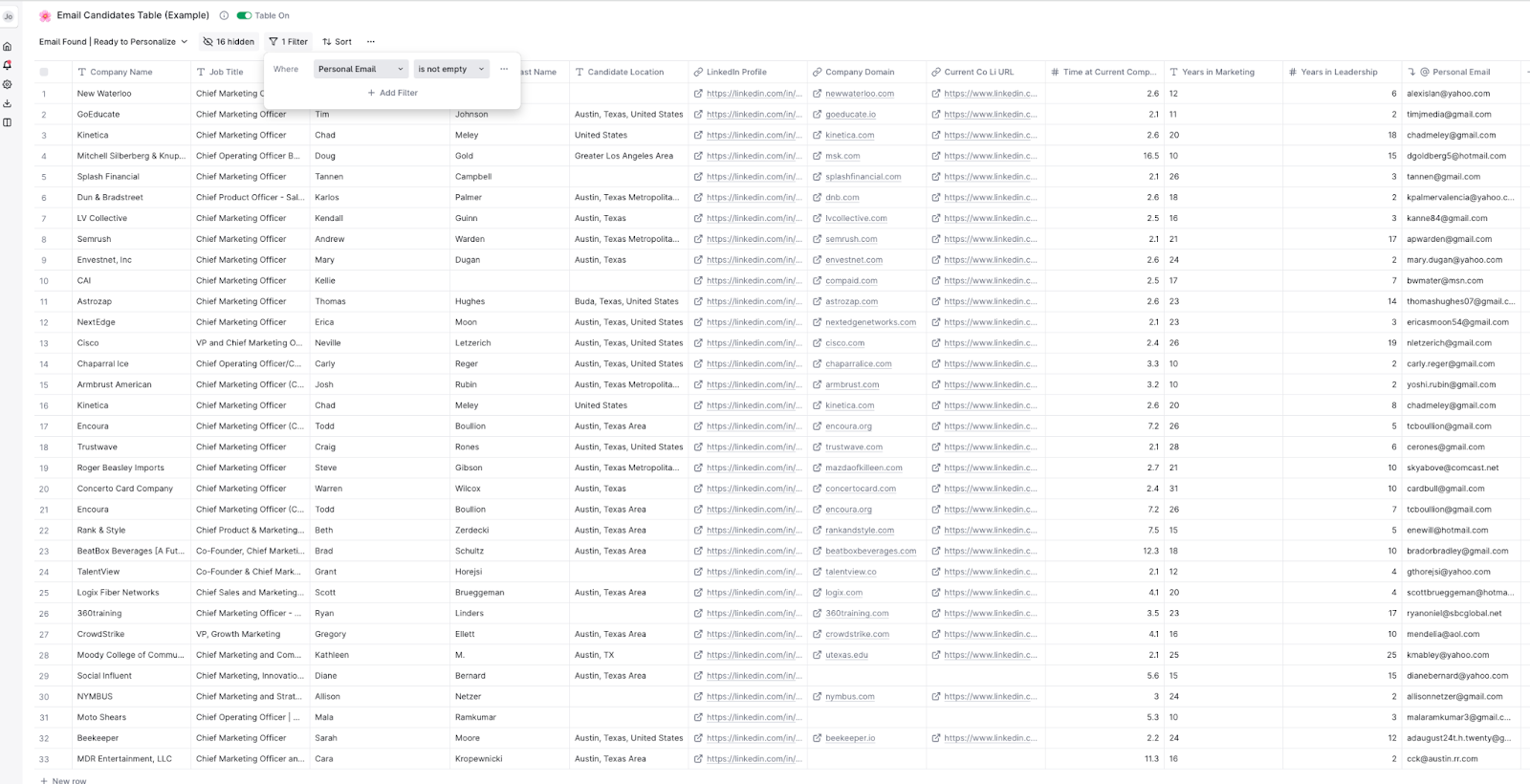
Pro Tip: Use AI to Normalize Company Name
Scraping LinkedIn and other data sources often returns company names with “LLC” or “Inc” or weird capitalization.
Sending an email with a raw company name that includes “LLC” is a dead giveaway that you didn’t write the email by hand. These small mistakes make a huge impact on your response rates because anything that looks like an automated email will often be ignored by a candidate.
Clay can “normalize company names” to remove any weird text from the data. Use the “Normalize Company Name” integration to turn raw data into “email ready” data.
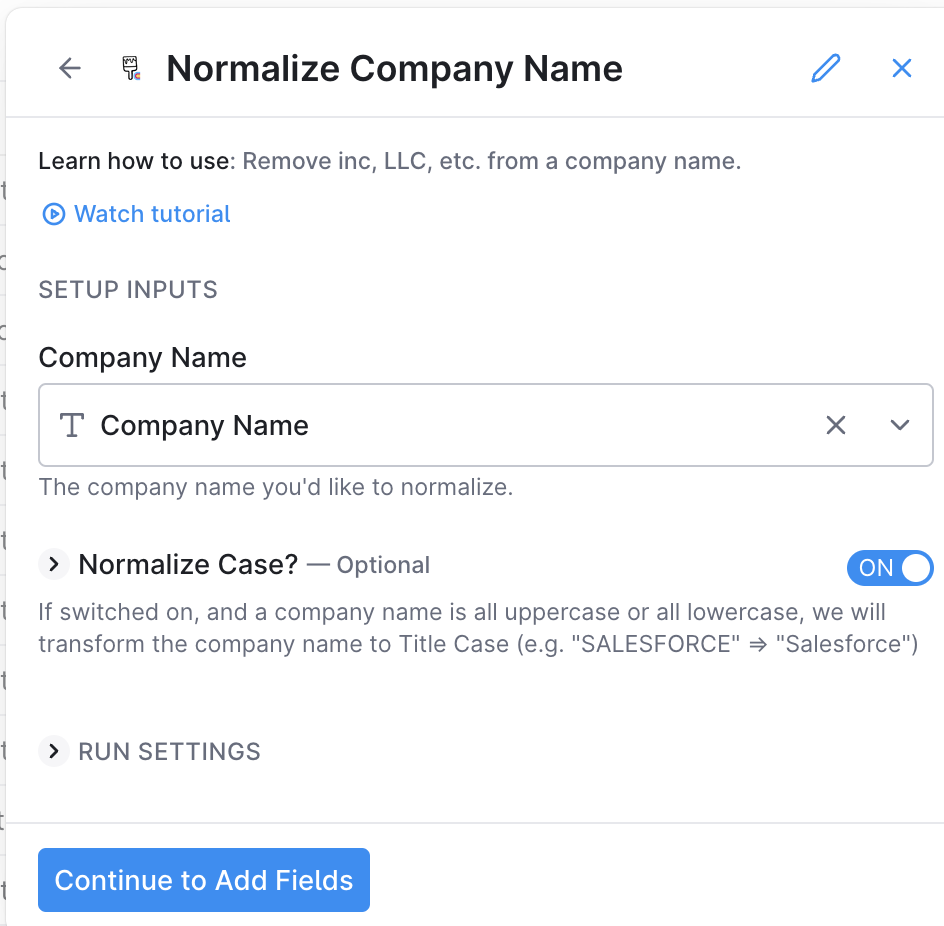
Here’s an example output:

Pro Tip: AI can help Remove Emojis from First Name
Relying on the candidate’s first name from their LinkedIn profile is a bad idea for two reasons:
- Candidates often add emojis to their first name
- Candidates often use their “full” first name, but usually “go by” a nickname
For example, let’s look at Felipe, Instantly’s partnership manager:
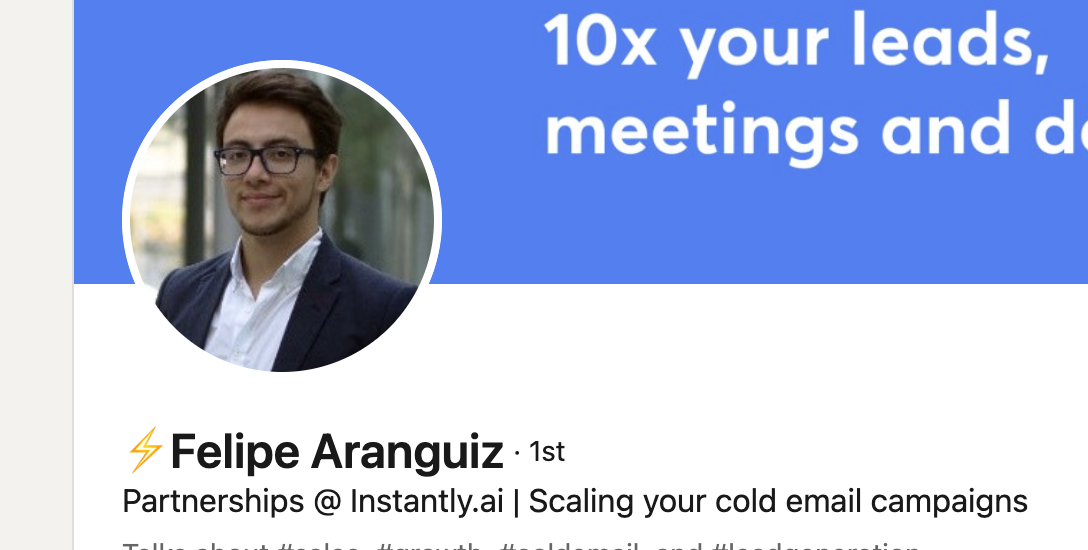
Felipe has a ⚡in his first name.
If we scraped his first name without formatting the data, we’d say “Hi ⚡️Felipe!”
He’d instantly (haha, get it?) know we sequenced him.
Instead, we’d want to format the first name to “Felipe”.
Let’s remove the emoji from the first name. Create a new column and use an AI formula to populate it with the candidate’s first name:
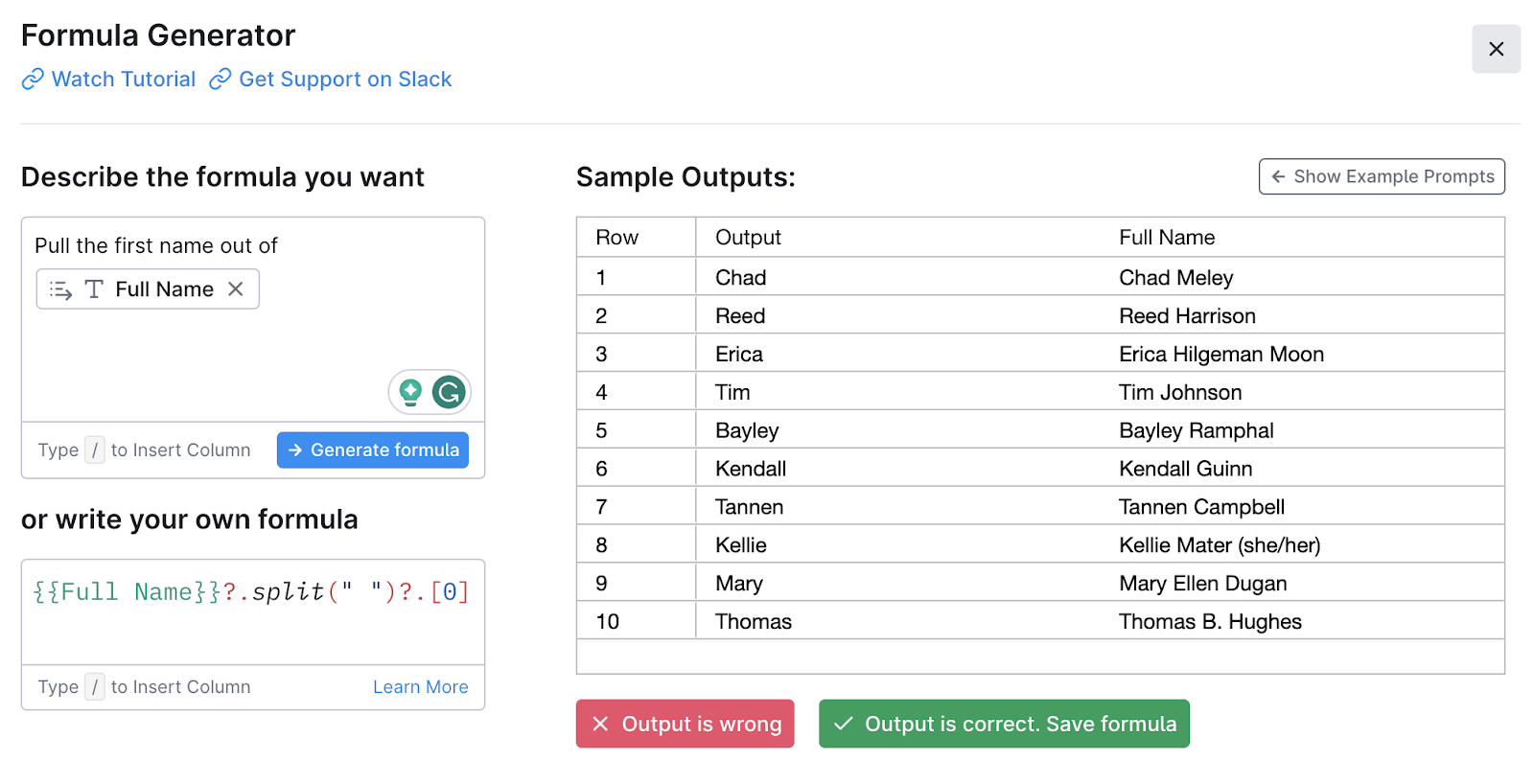
Repeat this step for the last name.
Then, pass the values into the “Normalize first and last names” integration:

As you can see, we removed the emoji from Felipe’s first name (and any other weird characters):

Pro Tip: Use AI to find preferred first names
Here’s another example:
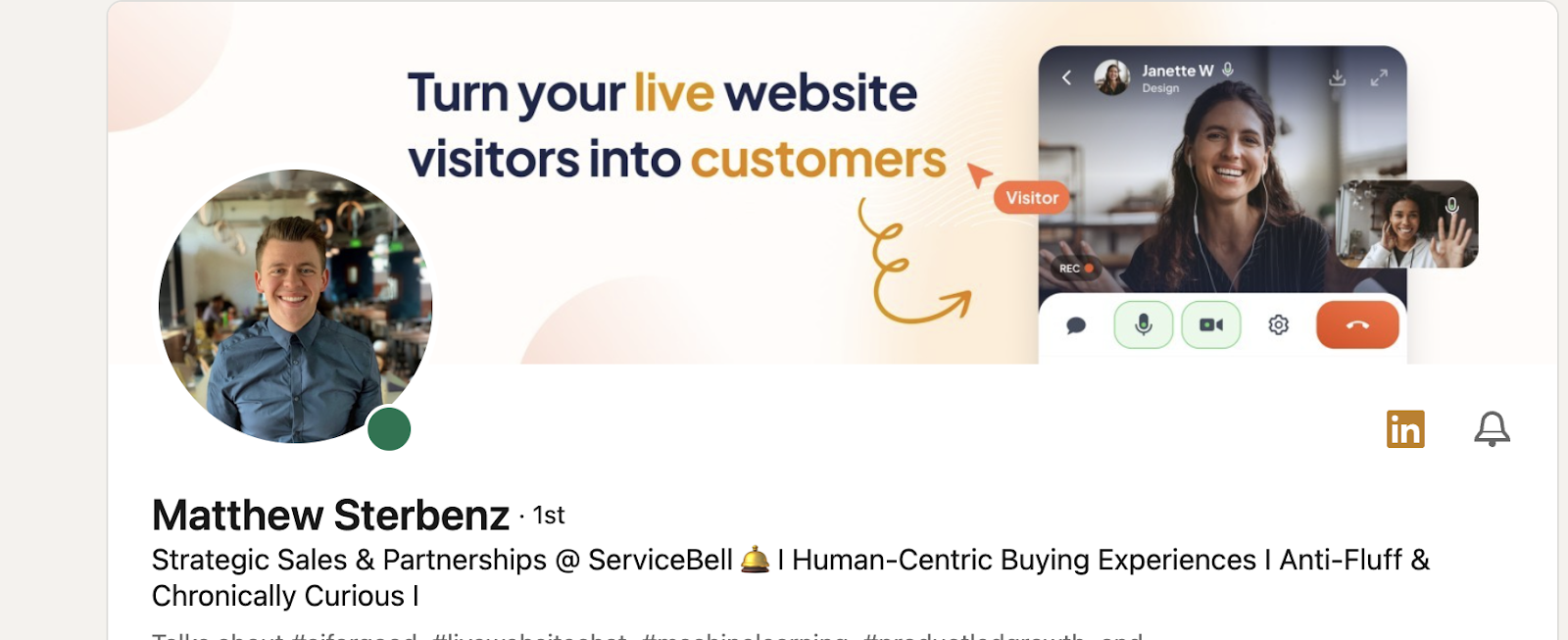
Matthew is a sales rep at ServiceBell.
His LinkedIn first name says “Matthew”, but if we look at his LinkedIn profile URL, it says “Matt”:

His email is “matt@servicebell.com”, not “matthew@servicebell.com”.
Lastly, we can see his peers call him “Matt” in his recommendations:
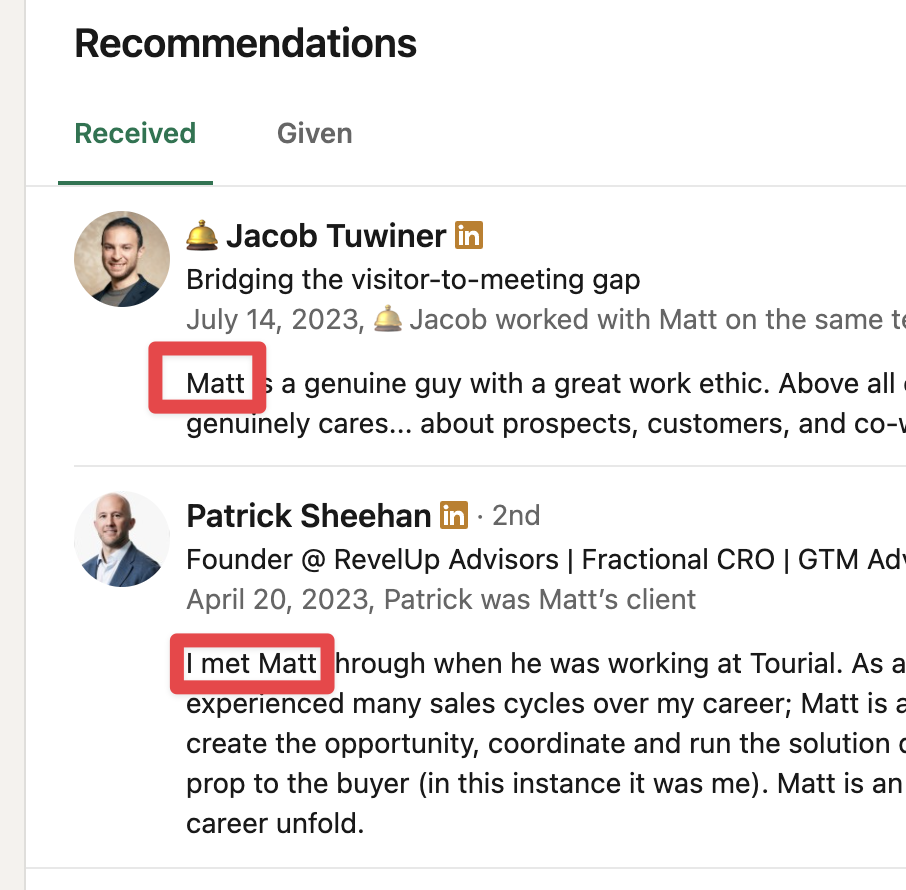
If we email him and say “Hi Matthew”, he’ll instantly know we didn’t write it ourselves (because we would have said “Hi Matt” instead.)
We can use Clay + ChatGPT to check the candidate’s LinkedIn URL and email to determine the candidate’s proper first name.
For example, this candidate calls himself “Gregory” in his LinkedIn first name, but his LinkedIn URL says otherwise.

We’ll use ChatGPT to check the candidate’s First Name, email, and LinkedIn URL, to determine the candidate’s proper first name:

Step Three: Use AI to personalize your recruiting emails
Now it’s time to personalize! We have all the raw data we need to get creative with GPT prompts.
Note: we’re personalizing after finding a verified email, so we don’t waste GPT credits on candidates without a verifiable email.
You could use GPT to write your entire cold email, but we will use GPT to insert custom variables in a pre-written email script.
Giving AI some guardrails help ensure consistency.
Here are some data points we’ll use for personalization:
- Candidate’s “general function”
- Years of experience in marketing and leadership
- Location
Candidate’s Job Function
Remember, we don’t want the candidate to feel like our email was sent to 100 other people.
Pasting the candidate’s job title into a cold email template is usually a dead giveaway that we dropped into a sequence.
Instead, we’ll use GPT to “massage” the data into a human, casual form so it comes across like we wrote it by hand.
For example, here’s a list of “general functions” using candidate job titles as the input:
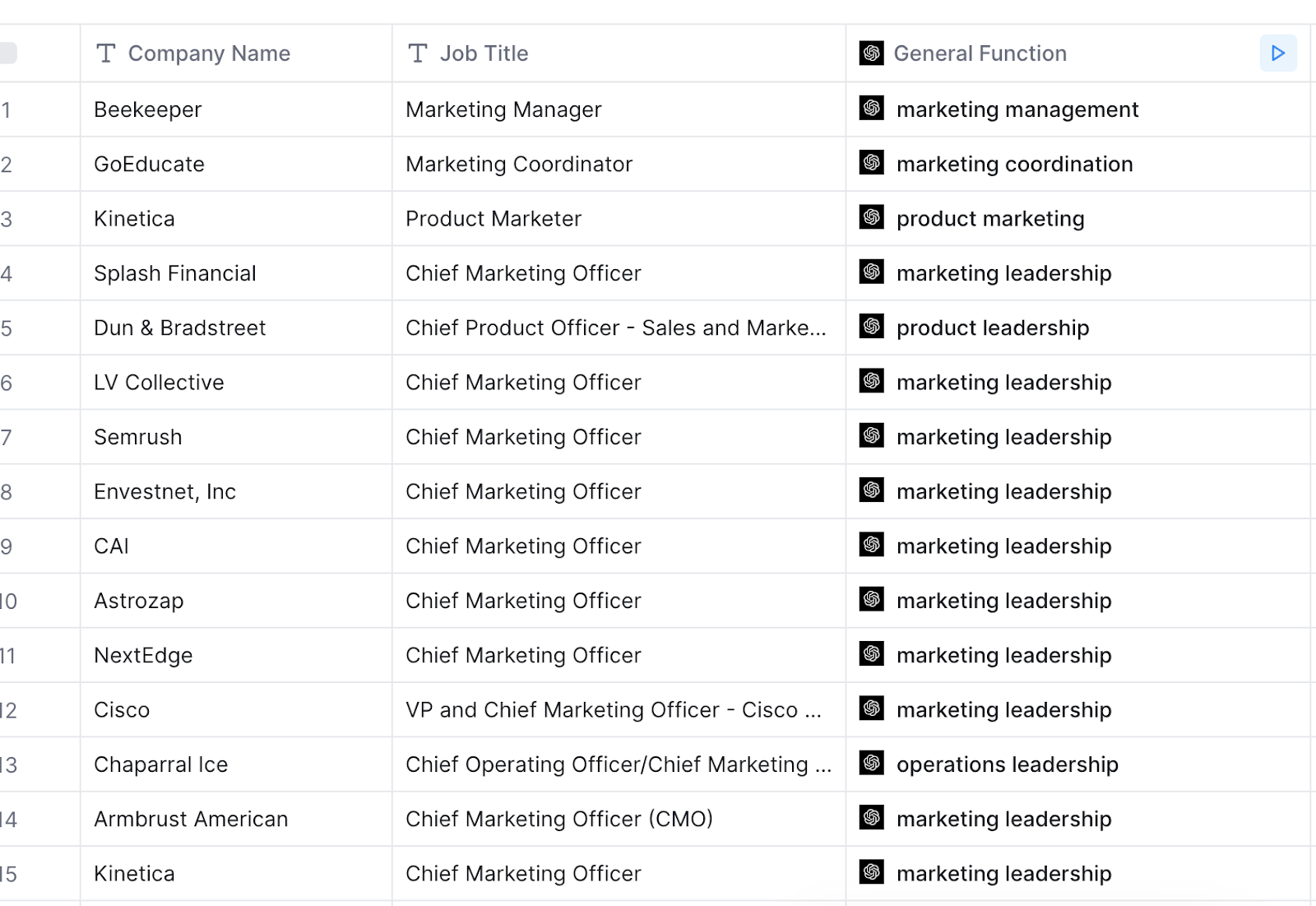
The general functions above would fit nicely in the following template:
“Hi {candidate name}, looks like you’ve been {general function} at {company name} for {years at company} years now.”
Populated with data from Clay, your opener would look like this:
“Hey Jacob, looks like you’ve been in marketing leadership at BeeKeeper for 2.5 years now.”
Here’s the GPT prompt we used:
Your job is to turn a job candidate's current job title into the job candidate's "general function". This is the candidate's job title: {Job Title}. A "general function" is a mix of their persona, and their senioriy level. Persona could be marketing, sales, customer success, operations, product, engineering, etc. Seniority could be leadership, management, or an individual contributor. For example, a "Chief Marketing Officer" job title's general function would be "marketing leadership". A "Marketing Manager" job title's general function would be "marketing management". A "Product Marketer" job title's general function would be "product marketing". The response will fit in the following script: "Hi {candidate first name}, looks like you've been in {general function} at {company} for {number of years} years now." Here's an example for a Chief Marketing Officer job title: "Hey John, looks like you've been in marketing leadership at Apple for 3.3 years now." Here's an example for a marketing manager job title: "Hey John, looks like you've been in marketing management at Apple for 3.3 years now." Here's an example for a product marketer (individual contributor) job title: "Hey John, looks like you've been in product marketing at Apple for 3.3 years now." If the candidate has multiple job functions in their title, determine the candidate's main job function, and only choose the main function. The main function is usually first in the title. For example, if the job title is "Chief Product Officer - Sales and Marketing Solutions", the general function is "product leadership". Keep the response under 5 words max. The response will usually be 2-3 words. The response should make sense in the script above. Your response is NOT the full sentence. It's only the text that will fit in {general function}. Your response should always make sense in the context of the script, but it is not the script itself. Never return your answer in quotations. Never return the title itself.
Normalize Location Name
You may want to confirm a candidate’s current location, especially if the job you’re filling requires in-person talent.
Mike Cohen’s script also had a cheeky “Still in {area}?” question in the opening line to add some personalization.
The raw data from LinkedIn is not ready for a cold email:
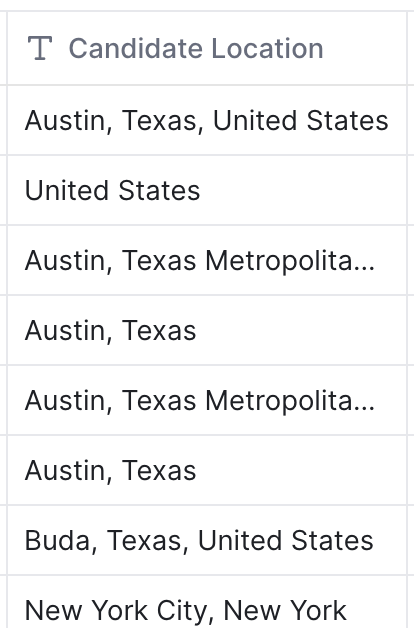
We’ll use a GPT prompt to normalize the location names, so we can use them in a personalized cold email (without looking like a robot).
Check out the results:
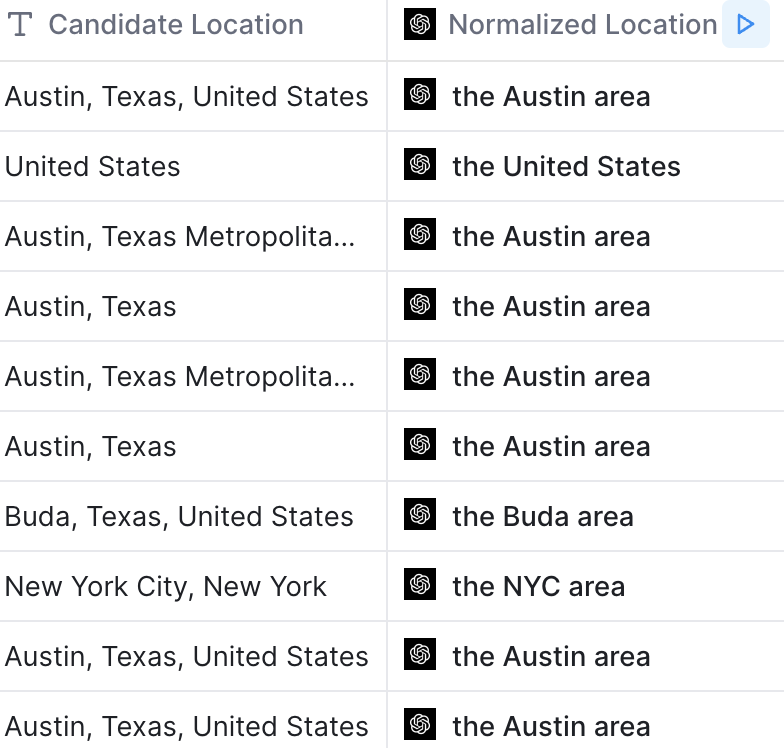
Building on our script example from before, you can now say:
“Hey Jacob, looks like you’ve been in marketing leadership at BeeKeeper for 2.5 years now. Still in the NYC area?”
Here’s the GPT prompt we used:
Your job is to normalize a candidate's location for use in a cold email script. You'll be given a candidate location. The candidate location usually contains the city, state, and country, but inputs will be different every time. This is the candidate location: {Candidate Location}
We want to normalize the text to fit in the following email script: "Still in {location}?" Usually, {location} output will be the name of the city or metropolitan area where the person lives. If you have a city or metropolitan area, return the name of the city or the metropolitan. For example, if the location is "New York City, New York", return "the NYC area" If the location is "Austin, Texas, United States", return "the Austin area" If the location is "United States", return "the United States." If the location is "Texas", return "Texas". Keep your response short, always under 5 words.
A Full AI Recruiting Email Sequence Template
Now we have all of the data we need to write a killer personalized cold email, using Mike Cohen’s email template that got a 31% message to meeting booked conversion rate!
Email 1: Initial outreach
Here’s the killer first email template Mike shared with us:
“Hi {first name},
Looks like you’ve been {general function} at {normalized company name} for {years in role}. I also noticed from your LinkedIn profile you’re living in {area}. Are you still with {company name} right now?
We currently have a unique opportunity for an experienced {job title you’re filling} here at {company name you’re hiring for}, one of the top {industry} companies in the world. You will be leading marketing, sales, and rev ops for new product execution and GTM strategies.
I’m offering two days and times for a quick phone call either this Thursday or Friday. I look forward to connecting soon.”
If you want to get creative with the email, you can add a personalized PS line to the end using the other data we gleaned from the profile:
“PS given your {years in marketing} and {years of leadership experience}, I think you’d be a perfect fit!”
Email 2: Stable Personality Type
The second email is meant for the “Stable” personality type on the DISC personality scale.
Stable personalities like lots of “we” language.
Here’s Mike’s script:
Hey {{first name]} - following up on my message {{previousStepDay}} about the VP of RevOps role.
What type of company are we?
Here’s an article from {{news network}} about {{topic}}:
{{link}}
And last, a few reason we should at least chat:
- {{Reason 1}}
- {{Reason 2}}
- {{Reason 3}}
Let me know if you have any availability early next week!
Email 3: Personalized Meme
Mike uses SourceWhale’s sequencing tool and data from Clay to generate personalized memes in his third email:
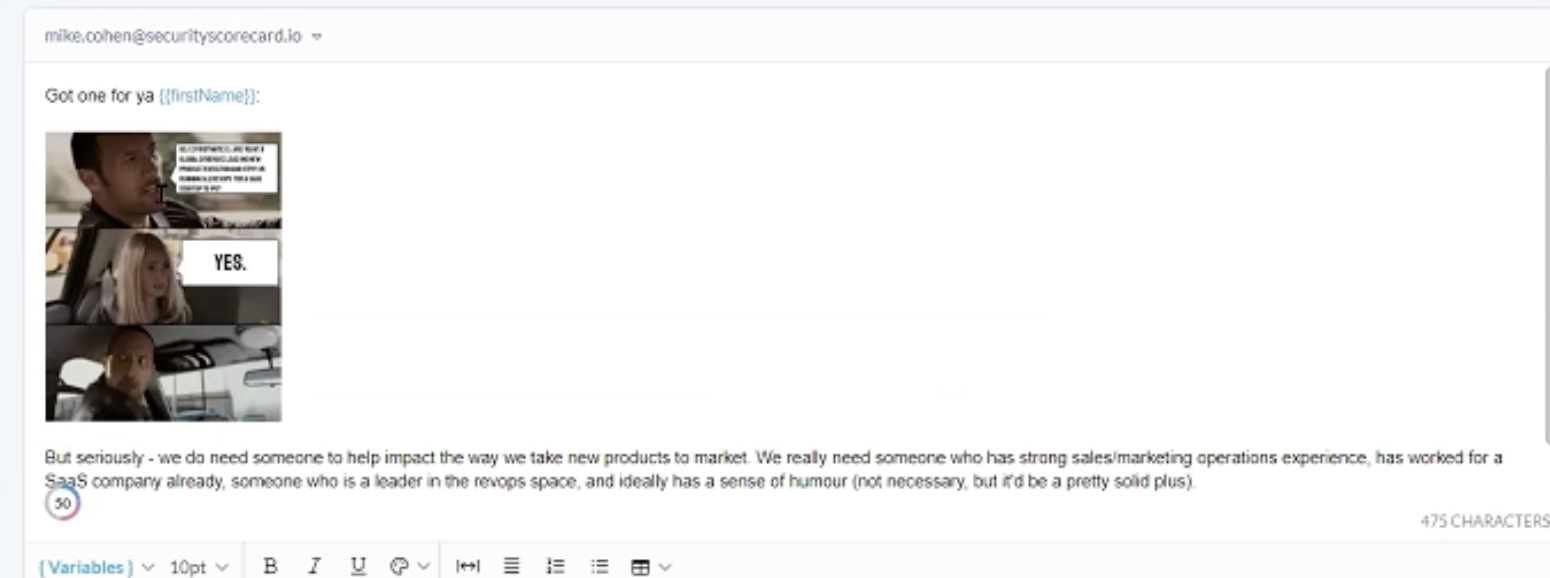
Fourth Email: Short and Sweet
The fourth email is short and sweet for people who don’t want to read:
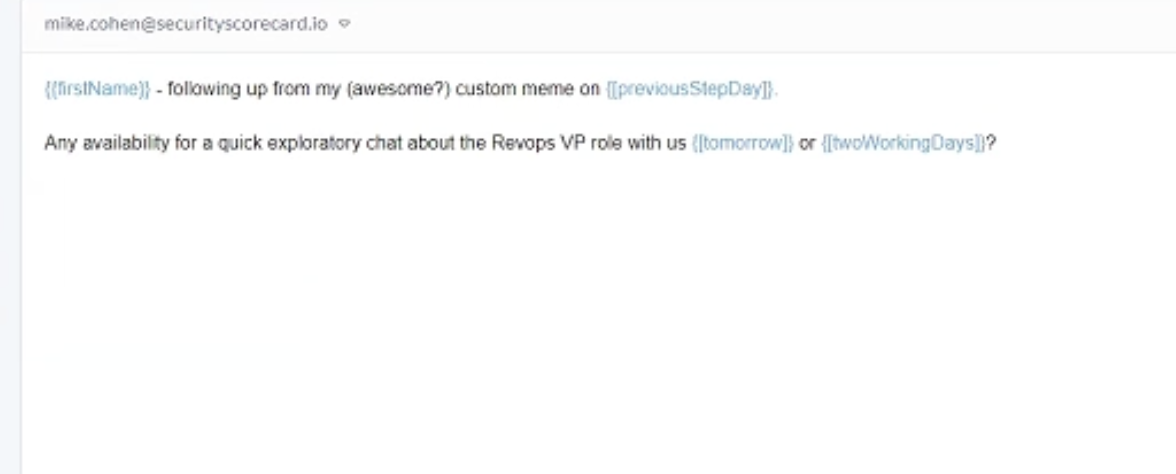
Fifth Email:
Mike ends his sequence with a picture of his dogs, for high “I” personalities.
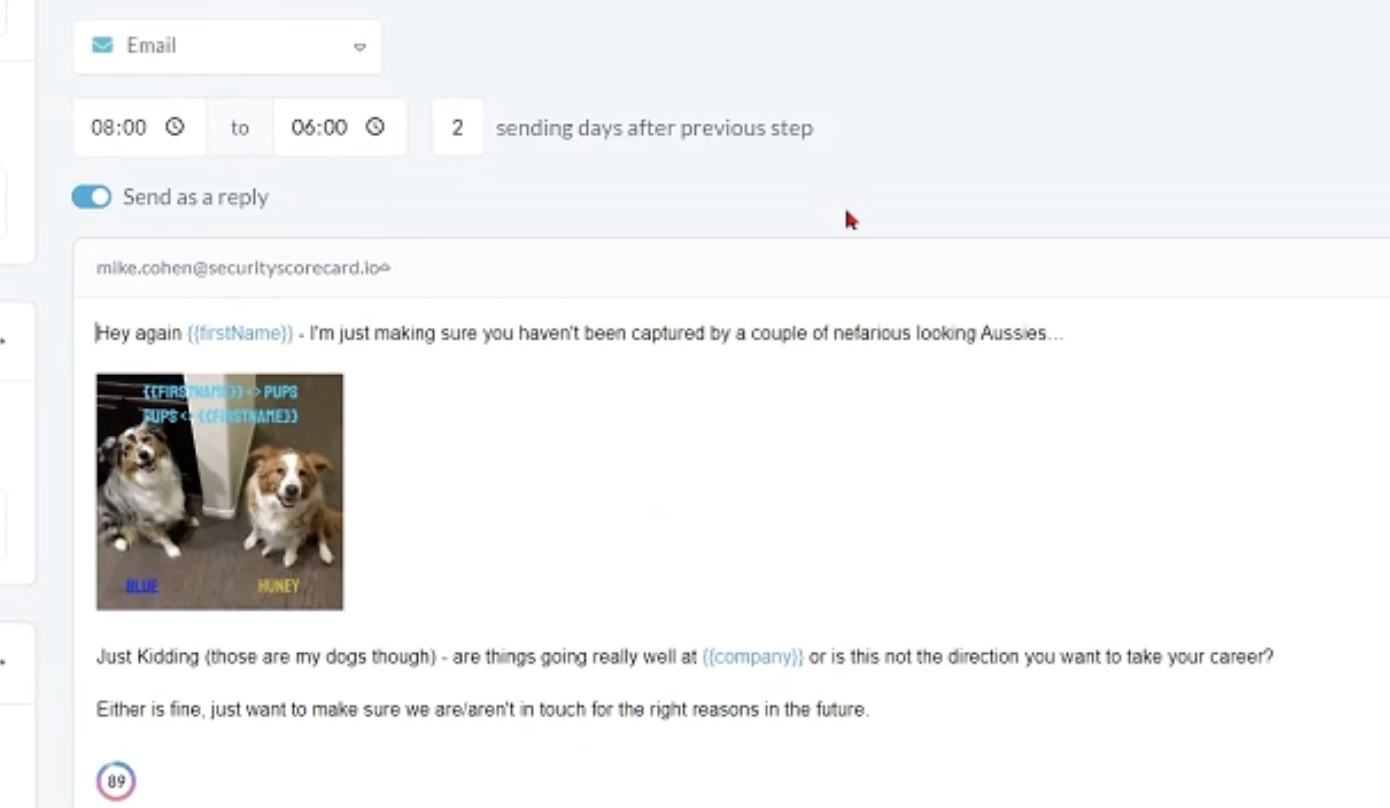
Results
The proof is in the pudding as they say… or in this case, the sequence statistics.
Using these AI recruiting strategies, here are Mike’s results for one of his sourcing clients.
One campaign:
- 102 candidates messaged
- 59.8% response rate
- 38 booked intro calls (37%)
Global client results:
- 561 candidates messaged
- 269 responses (48% response rate)
- 173 booked intro calls (31% messaged candidates booked. 64% of replies are interested)
Not too shabby, eh? Try our AI recruiting workflows out for yourself and let us know your results in the comments below 👇🏻